This post is going to assume some knowledge of AI safety as a field. The short explanation is that some people think that artificial general intelligence (AGI) has the potential to cause human extinction, or close to it, because of the difficulty of correctly specifying human goals. To try to get a sense of this, imagine designing a fully autonomous, superintelligent cleaning robot and trying to design a numerical reward function that it can use to learn how to clean stuff. Now imagine a baby goes into the room it’s trying to clean, or that the room has priceless ming vase, or frayed electricity wires. AI safety is the study of how to try to make sure that any very powerful AI systems we design are good for the world.
Cross posted to Lesswrong and The Good Blog
Summary
- AI safety research improves capability by making AIs done what humans want
- Having more capability means that AI is more likely to be deployed
- If AI safety is really hard then AI we think is safe at deployment is likely to be unsafe
- This effect is mitigated if safety failures are continuous - in this world the more total safety research done the better
- Highly theoretical AI safety research is plausibly not going to be done anyway and so adds to the total amount of safety research done
- Empirical safety research has a smaller counterfactual impact
- The effect of this could go either way depending on weather safety failures and discrete or continuous
What do we mean by capability
There is an argument that safety research is bad because getting a utility function which is close to one that kind, sensible humans would endorse is worse than missing completely. This argument won’t be the focus of this blog post but is well covered here.
I will argue that another harm could be that safety research leads to an unsafe AI being deployed more quickly, or at all, than without the safety research being done.
The core of this argument is that AI safety and AI capability is not orthogonal. There are two ways capability can be understood: firstly as the sorts of things an AI system is able to do and secondly as the ability of people to get what they want using an AI system.
Safety is very clearly not orthogonal under the second definition. The key claim made by AI safety as a field is that it’s possible to get AIs which can do a lot of things but will end up doing things that are radically different from what a human principal wants it to do. Therefore improving safety improves this dimension of capability in the sense that ideally a safer AI is less likely to cause catastrophic outcomes which presumably their principals don’t want.
It’s also plausible that under the second definition of capability that AI safety and capabilities are not orthogonal. The problem that value-learning approaches to AI safety is trying to solve is one of attempting to understand what human preferences are from examples. Plausibly this requires understanding how humans work at some very deep level which may require substantial advances in the sorts of things an AI can do. For instance it may require a system to have a very good model of human psychology.
These two axes of capability give two different ways in which safety research can advance capabilities. Firstly by improving the ability of principals to get their agents to do what they want. Secondly, because doing safety research may, at least under the value learning paradigm, require improvements in some specific abilities.
How does this affect whether we should do AI safety research or not?
Whether or not we do AI safety research I think depends on a few variables, at least from the perspective I’m approaching the question with.
- Is safe AI discrete or continuous
- How hard is AI safety
- What are the risk behaviours of the actors who choose to deploy AI
- How harmful or otherwise is speeding up capabilities work
- How likely is it that TAI is reached with narrow vs general systems
How does safety interact with deployment?
I think there are few reasons why very powerful AI systems might not be deployed. Firstly, they might not be profitable because they have catastrophic failures. A house cleaning robot that occasionally kills babies is not a profitable house cleaning robot.[1] The second reason is that people don’t want to die and so if they think deploying an AGI will kill them they won’t deploy it.
There are two reasons why an AGI might be deployed even if the risk outweighs the reward from an impartial perspective. There’s individuals having an incorrect estimation of their personal risk from the AGI. Then there’s also individuals having correct estimations of the risk but there are very large - potentially unimaginably vast - externalities, like human extinction.
So we have three ways that AI safety research might increase the likelihood of a very powerful AGI being deployed. If AI systems have big discontinuities in skills then it’s possible AI systems, if there’s at least some safety work, look safe until they aren’t. In this world, if none of the lower level safety research had been then weaker AI systems wouldn’t be profitable because they’d be killing babies while cleaning houses.
It seems very likely that AI safety research reduces existential risk conditional on AGI being deployed. We should expect that the risk level acceptable to those taking that decision to be much higher much higher than socially optimal because they aren’t fully accounting for the good lives missed out on due to extinction, or the lives of people in an AI enabled totalitarian nightmare state. Therefore they’re likely to accept a higher level of risk than is socially optimal, while still only accepting risk below some threshold. If AI safety research is required to get below that threshold, then AI safety research takes the risk below that threshold meaning AI could be deployed when the expected value is still massively negative.
Relatedly, if AGI is going to be deployed it seems unlikely that they’ve been lots of major AI catastrophes. This could mean that those deploying AI underestimate their personal risk of AGI deployment. It’s unclear to me whether, assuming people take seriously the threat of AI risk, whether key decision makers are likely to be over or under cautious (from a self-interested perspective.) One on hand, in general people are very risk averse, while on the other individuals are very bad at thinking about low probability, high impact events.
Value of Safety research
If AI being safe - in the sense of not being an existential risk - is a discrete property then there are two effects. Firstly, if AI safety is very hard then it’s likely (though not certain) that the marginal impact of AI safety research is small. The marginal impact of safety research is given by two variables: the amount that safety research increases the total amount of research done, and the amount that that increases in the total amount of research done reduces the probability of x-risk. If we’ve only done a very small amount of research then adding any extra research means we’ve still only done a very small amount of research so AI is still unlikely to be safe. There’s a similar effect from doing a large amount of research - adding more research means we’ve still done a lot of research and so it’s very likely to be safe. The large effect on the probability comes when we’ve done a medium amount of research.
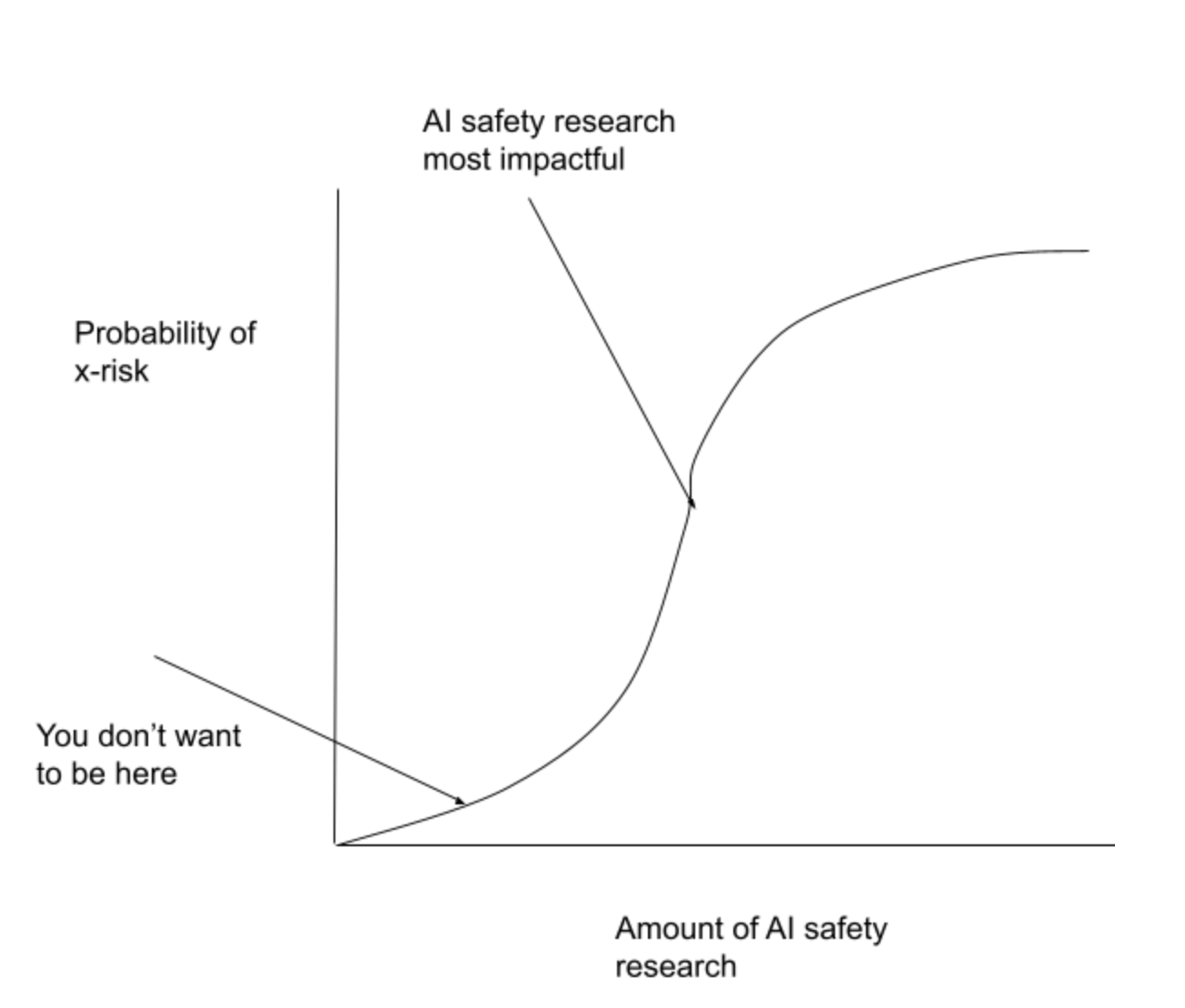
How bad this is depends on the specific way in which AI in which AI failure manifests and how discontinuous the jump is from ‘normal’ AI to x-risk threatening AI. The worst world is the one in which getting very near to safety manifests as AI being safe until there’s a jump to AGI because in this world it’s likely that firms will be successfully building highly profitable products meaning that they’re expecting their next, more powerful, AI system to be safe. This world seems plausible to me if there are discontinuous jumps in capabilities AI systems improve. Alternatively there could be certain skills or pieces of knowledge, like knowing it’s in a training environment, that dramatically increase the risks from AI but are different problems faced by less powerful systems.
On the other hand, if we’re in a world where it’s touch and go whether we get safe AI and prosaic AI alignment turns out to be the correct strategy then AI safety research looks extremely positive.
This looks different if AI safety failures are continuous. In this case any research into AI safety reduces the harms from AI going wrong. I think it’s much less clear what this looks like. Potentially a good sketch of this is this blog post by Paul Christiano where he describes AI catastrophe via Goodhearting to death. Maybe the closer an AGI or TAI (transformative AI) values are to our own, the less harmful it is to fall prey to goodhearts law, because the the thing being maximised is sufficiently positively correlated with what we truly value that it stays correlated even in a fully optimised world. I haven’t tried to properly work this out though.
Implications for altruistically motivated AI research
There are few quite different ways in which this could work. Extra research could just be additional research that wouldn’t have been done otherwise. This seems most likely to be the case for highly theoretical research that only becomes relevant to very powerful models, meaning there’s little incentive for current AI labs to do the research. This seems to most clearly fit agent foundations and multiagent failure research. This research has the property of applying to large numbers of different classes of models working on very different things. This means it displays strong public good properties. Anyone is able to use the research without it being used up. Traditionally, markets are believed not to supply these kinds of goods.
On the end of the scale research is done to prevent large language models saying racist things. There are only a very small number of firms that are able to produce commercially viable large language models and it’s plausible you can find ways to stop these models saying racist stuff that doesn’t generalise very well to other types of safety problems. In this case firms capture a lot of the benefits of their research.
The (dis)value of research between these two poles depends on how useful the research is to solving pre AGI safety problems, whether failure is discrete or continuous, and how hard the safety problem is relative to the amount of research being done.
The best case for empirical research on currently existing models being valuable is that failure is that the safety problem is relatively easy, prosaic alignment is possible, this sort of safety research doesn’t advance capabilities in ability to do more stuff sense, and that preventing x-risk from AGI is all-or-nothing. In this world altruistic safety would probably increase the total amount of relevant safety research done before AGI is deployed, and if it means that AI is more likely to be deployed that safety research will still have at least some effect because failure is continuous rather than discrete.
The world where this is worst is where AI alignment is very hard but key decision makers don’t realise this, safety is discrete and we need fundamentally new insights about the nature of agency and decision making to get safe AGI. In this world it seems likely that safety research is merely making it more likely that an unsafe AGI will be deployed. Because the problem is so hard it’s likely that the safety solution we find and relatively small amounts of research is likely to be wrong, meaning that the marginal contribution to reducing x-risk is small, but there’s quite a large effect on how likely it is that unsafe AI is deployed. The best case here is that safety has a very small marginal impact because it’s replacing safety work that would be done anyway by AI companies - this case the biggest effect is probably speeding up AI research because these firms have more resources to devote to pure capabilities research.
The worst case for more abstract research, ignoring concerns about the difficulty of knowing that it’s relevant at all, is that it actually is relevant to nearly-but-not-quite AGI and so provides the crucial step of ensuring that these models are profitable, while also facing safety being a descretre property and AI safety being a really hard problem. This could easily be worse than the worst case for empirical alignment research because it seems much more likely that this theoretical research wouldn’t be done by AI companies, both because currently this work is done (almost?) exclusively outside of industry and exhibits stronger public goods properties because it isn’t relevant only to firms with current access to vast amounts of compute.
Why aren’t AI labs doing safety research already?
If AI safety labs weren’t doing any AI safety research currently, this would point to at least some part of the theory that capabilities and safety aren’t orthogonal being wrong. It’s possible that safety displays strong public goods properties which means that safety research is much less likely to be done than other sorts of capabilities research. Basically though, I think AI safety research is being done today, just not of the sort that’s particularly relevant to reducing existential risk.
Victoria Kranova has compiled a list of examples of AI doing the classic thing that people are worried about an AGI doing - taking some goal that humans have written down and achieving it some way that doesn’t actually get at what humans want. The process of trying to fix these problems by making the goal more accurately capture the thing you want is a type of AI alignment research, just not the type that’s very helpful for stopping AI x-risk, and highly specific to the system being developed which is what would be predicted if more theoretical AI safety work had stronger public goods properties. This article gives a really good description of harm caused by distributional shift in a medical context - trying to change I think should be thought of as a type of AI alignment research in that it’s trying to get an AI system to do what you want and focus is on changing behaviour rather than trying to make the model a better classifier when it’s inside it’s distribution.
Takeaway
I think this area is really complex and the value of research is dependent on multiple factors which interact with one another in non-linear ways. Option value considerations dictate that we continue doing AI safety research even if we’re unsure of its value because it’s much easier to stop a research program than to start one. However, I think it’s worthwhile trying to formalise and model the value of safety research and put some estimates on parameters. I think it’s likely that this will push us towards thinking that one style of AI research is better than another.
I think the opposite is often true. Once there are people who get compensated for doing X it can be very hard to stop X. (Especially if it's harder for impartial people, who are not experts-in-X, to evaluate X.)
Yeah I think that's very reasonable
I think this is a pretty important topic, and one I haven't seen discussed as often as I'd like! Thanks for writing it up.
I think you could get more engagement with this topic if you spent some more time smoothing out the presentation of your writeup. For example, there are a few typos in the summary section that made me less excited to read the rest of the piece. Given that you now have a pretty interesting piece of thinking written, it might be pretty feasible to find a smart junior person who could give you copyedits and comments.
Thanks for you feedback! Unfortunately I am a smart junior person, so looks like we know who'll be doing the copy editing
Ha!
Personally, I've gotten a lot of value in having a buddy look over my work and chat with me about it -- a fresh perspective is really useful, not just for copyedits but also for building on my first thoughts. If you don't yet know people you could ask for this, you might find it valuable to reach out to SERI, CERI, or other community orgs that aim to help junior x-risk researchers. (presumably ZERI and JERI are next.) Happy to chat more via DM if that would be useful :)
What are ZERI and JERI?