This post is prompted by John Halstead's piece The ITN framework, cost-effectiveness, and cause prioritisation. In my D. Phil. thesis I have a chapter which is similarly motivated to clarify and assess EA's cause prioritisation methodology. I was planning to re-write the chapter and put on the forum eventually; seeing as John has made this area topical, my analysis is somewhat distinct, and I do not currently have time to change the (5,000 word) chapter, I will simply put the original chapter it here in its entirety in the hope it's useful.
The chapter title and abstract are below, followed by a quick note on what I see as the key differences between Halstead and I's analyses of the topic, and then the text of the chapter itself. To be clear, my chapter is not written as a response to Halstead and thus I do not distinguish, except in section 0.5 and the following sentence, how my analysis differs from his. One terminological distinction is that Halstead talks of the 'importance, tractability, neglectedness' framework and I use the word 'scale' instead of 'importance'.
Chapter 5: How should we prioritise among the world’s problems?
0. Abstract
In this chapter I set out what I call the ‘EA method’, the priority-setting methodology commonly used by members of the effective altruist (EA) community, identify some open questions about the method, and address those questions. I particularly focus on the three-factor ‘cause prioritisation’ framework part of the EA method. While the cause prioritisation framework is regularly appealed as a means of determining how to do the most good, it appears not to have been carefully scrutinised; its nature and justification are somewhat obscure. I argue that the EA method should be moderately reconceptualised and that, once it has been reconceptualised, we realise the method is not as useful we might have thought or hoped. Some practical suggestions are made in light of this.
0.5. The differences between this article and Halstead's
I assume readers have already read Halstead's piece. The differences between this article and Halstead's, and the further contributions I make, are : (1) I explore and explain the conceptual distinction between evaluating (a) 'causes' in terms of scale, neglectedness and tractability and (b) evaluating interventions in terms of marginal cost-effectiveness. I argue this distinction is often non-existent or slight; Halstead's analysis works on the assumption there is a distinction (a) and (b); (2) I show how scale, neglectedness, and tractability can sometimes be used to determine which problems are low-priority; (3) I explain why it's plausible to consider scale, neglectedness, and tractability as the three, and only three, factors which together determine marginal cost-effectiveness, and hence why all three need to be considered in cases where problems have diminishing marginal returns; Halstead claims neglectedenss is better understood as a factor that determines tractability; (4) I propose a different three-step priority-setting process; (5) I argue that it is generally mistaken to think we can evaluate problems 'as a whole' - our evaluations of which problems are a priority should really be understood as assessments of the cost-effectiveness of the best particular solutions to those problems.
1. Introduction
Let’s begin with a long quote from William MacAskill, one of the effective altruism community’s leaders, describing what I call the ‘Effective Altruism method’ or ‘EA method’, for short:
Suppose we accept the ideas that we should be trying to do the most good we can with a given amount of resources and that we should be impartial among different causes. A crucial question is: how can we figure out which causes we should focus on? A commonly used heuristic framework in the effective altruism community is a three-factor cause-prioritization framework. On this framework, the overall importance of a cause or problem is regarded as a function of the following three factors
· Scale: the number of people affected and the degree to which they are affected.
· Solvability: the fraction of the problem solved by increasing the resources by a given amount.
· Neglectedness: the amount of resources already going toward solving the problem.
The benefits of this framework are that it allows us to at least begin to make comparisons across all sorts of different causes, not merely those where we have existing quantitative cost-effectiveness assessments. However, it’s important to bear in mind that the framework is simply a heuristic: there may be outstanding opportunities to do good that are not in causes that would be highly prioritized according to this framework; and there are of course many ways of trying to do good within highly ranked causes that are not very effective. (Italics in original)[1]
As such, it seems the EA method has two main steps:
1. Cause prioritisation: comparing the marginal cost-effectiveness of different problems. This is done using the three-factor framework, i.e. assessing causes by their scale, neglectedness and solvability (these sometimes go by different names)[2]
2. Of the causes which are most promising, move on to intervention evaluation: creating quantitative cost-effectiveness estimates of particular solution to given problems.
Although the three-factor cause prioritisation method is popular—central, even—to effective altruists’ discussions about how to do the most good, there has been little analysis of the topic. There are a number of open questions about the method. I’ll list and motivate a number of them.
First, what’s the distinction between cause prioritisation and intervention evaluation? Following the quotation from MacAskill, it seems we can evaluate which causes are higher priority, that is, have greater cost-effectiveness, before making quantitative cost-effectiveness assessments of particular interventions. But it’s not immediately clear how we can determine which causes are higher priority prior to considering, at least implicitly, the interventions we might use in each case and how cost-effective those interventions are.
Second, and assuming there is a distinction between the two steps, what reason is there for engaging in the cause prioritisation at all? We could just jump to intervention evaluation—e.g. assessing different poverty alleviation charities we could give to—and bypass cause prioritisation altogether.
Third, what role do the three factors—scale, neglectedness and solvability—play in helping us to determine a cause’s priority?
Fourth, why are there three factors (rather than, say, four) and why are these particular factors used?
I aim to clarify matters by working through these questions.
The chapter is structured as follows. Section 2 suggests an initial distinction between cause prioritisation and intervention evaluation and explains how it is sometimes possible to determine causes are low-priority without examining particular. Section 3 considers how to evaluate plausibly high-priority causes, distinguishes two stages of cause prioritisation and explains why and how to use scale-neglectedness-solvability framework to determine the cost-effectiveness of causes at this second stage. Section 4 argues there is a very thin distinction between the second stage of cause prioritisation and intervention evaluation, suggests a slight reconceptualisation of the EA method, notes some worries that result from this, and asks whether this reconceptualisation is surprising. Section 5 makes some concluding remarks.
2. How and why to prioritise causes
It will help us to answer the questions posed if we realise that ‘causes’ and ‘interventions’ are just different words for ‘problems’ and ‘solutions’, respectively.[3] To be clear, if we want to solve a problem, we will eventually have to use some particular solution(s) to the problem. Hence, reframed this way, the query becomes whether and how it is possible to evaluate problems in some sense, ‘as a whole’, as a distinct process from evaluating particular solutions to those problems.
The explanation seems to be that we can evaluate problems as a whole if and when we can say something about the cost-effectiveness of all the solutions to a given problem. (From here, from stylistic reasons, I will usually talk of ‘evaluating problems’ instead of ‘evaluating problems as a whole’ even though the longer phrase is more appropriate). In this case, we can evaluate the priority of a problem without having to look too closely at any of the particular solutions. Doing this is convenient because it allows us to quickly discard problems in which all the solutions seem cost-ineffective and hence focuses our attention on investigating the more promising problems. There seem to be three situations where we can evaluate a problem and discard it as low-priority.
First, if the problem is not solvable by any means. For instance, we might say putting our resources (i.e. money or time) towards gun control in America is not a good altruistic decision. All solutions seem to involve political reform; opposition to gun control is sufficiently strong it’s hard to imagine any political effort would succeed. Hence, it’s unnecessary to get into the specifics of any particular solution/intervention to gun control because we are confident none of them will be as cost-effective as some alternative altruistic option we already have in mind (perhaps e.g. alleviating poverty). Another example would be if someone suggested building a perpetual motion machine and showed us their blueprints—we can rule out all particular solutions as being unfeasible without needing to look at the schematics for this version.
Second, if the problem will be solved by others whatever we do, our efforts will have no (or only a tiny) counterfactual impact. Some people think working to reduce climate change is a bit like this: there are now so many concerned individuals that my impact would be nearly nil, and I’ll do more good by doing something else. A further, stylised example would be that a child has fallen into a shallow pond and someone else is already saving them—it won’t help if you jump in too.
Third, if the problem is so tiny it’s clear that putting any effort towards solving it would be a waste (compared to some already known potentially effective alternative). Potentially, saving some rare species of beetle is like this. While it would (perhaps) be good if you did so, rather than doing nothing, you might conclude, without needing to look further at the details, the value will be so small that something else must be a higher priority.
Rather neatly, and perhaps unsurprisingly, the three types of situation each draw on one of the three factors used for cause prioritisation—solvability, neglectedness, and scale, respectively. As we’re understanding intervention evaluation as the process of examining particular solutions to a problem, the foregoing analysis explains how and when we can engage in cause prioritisation without needing to get into intervention evaluation: sometimes we can ‘screen out’ entire problems quite quickly by noting something about all their solutions and concluding that none of them is comparatively cost-effective.[4] Given we’re looking to do as much good as possible and our resources are finite, time spent investigating many different ways to solve unimportant problems is time wasted.
A potentially helpful analogy here is determining where to go on holiday. In the end, we have to visit a particular part of a country, rather than every part (or an average part) of a country.[5] Nevertheless, when thinking about our choice, we might start by seeing what we can say about countries as a whole (cause prioritisation) before considering the individual locations in more detail (intervention evaluation). If the country has features that make it unappealing—it’s ruled by a dictator, they speak French there, it’s very expensive, etc.—we can rule out going there without the need to look too closely at any particular places in those countries we could visit.
3. Prioritising causes using scale, neglectedness, and solvability
In the examples above, we could easily screen out those causes for one reason or another. Of course, we would also want our cause prioritisation methodology to tell us, of the problems which remain, which are the highest priority. Let’s distinguish two stages of cause prioritisation. Stage A is where we screen out some of the problems, which we do by assessing all their solutions—this is the stage we have already discussed. At stage B, we determine the marginal cost-effectiveness of the problems which remain. In this section, I explain how the three factors (scale, neglectedness, solvability) combine to determine marginal cost-effectiveness of different causes and allow us to make progress at stage B.
MacAskill, in an explanatory footnote, offers more formal definitions of the terms used informally in the first quote and sets out how the three factors combine to determine the cost-effectiveness of a cause:
Formally, we can define these as follows: Scale is good done per by percentage point of the problem solved; solvability is percentage points of a problem solved per percentage point increase in resources devoted to the problem; neglectedness is percentage point increase in resources devoted to the problem per extra hour or dollar invested in addressing the problem. When these three terms are multiplied together, we get the units we care about: good done per extra hour or dollar invested in addressing the problem.[6]
We can write this as an equation:
Scale x Solvability x Neglectedness = Good done/dollar
(Good done/% solved) x (% solved/% increase in resources) x (% increase in resources/extra dollar) = Good done/dollar
Hence, scale, neglectedness and solvability are defined as factors which, when multiplied together, calculate the cost-effectiveness of whichever cause is being analysed.
It’s worth briefly noting the existence of an earlier/alternative version of the three-factor framework that is qualitative in nature.[7] On the qualitative version, the same three factors were thought relevant to understanding a cause’s cost-effectiveness—causes that were larger, more solvable and more neglected were presumed to be higher priority. However, there was no mechanism to trade-off the factors against each other or to combine them to determine cost-effectiveness, something this quantitative version allows. I use the newer quantitative version as it—unlike the older, qualitative one—allows us to determine the cost-effectiveness of causes and thus compare them to each other on that basis.
This brings us to the question of why there are three factors (rather than, say, four) and why these particular factors are used. This becomes clear once consider that causes generally have diminishing marginal returns: individuals will pick the ‘low hanging-fruit’ first, which means that initial resources put towards the problem will do more good per unit of resource than those added subsequently. This is represented in figure 5.1.
We can now point out the role each of the three factors plays. To determine the cost-effectiveness of additional resources, we need to know three things. First, the scale of the problem—how far up the Y-axis, which represents value, the line goes (how much fruit there is to pick in a given field).
Second, how much of the problem is solved for different amounts of resources, which is what solvability refers to (how easy it is to pick the fruit in this field and how this gets progressively harder). Combined with scale, this gives the shape of the cost-effectiveness line, which I’ll call the solvability line. We cannot determine the solvability line with a single assessment, which is what we might have thought, but a series of assessments: given diminishing marginal returns, the fraction of the cause that is solved for a given amount of resources reduces as more resources are added. If there were instead constant marginal returns, that is, the cost-effectiveness was linear, then one assessment of the solvability line would suffice.
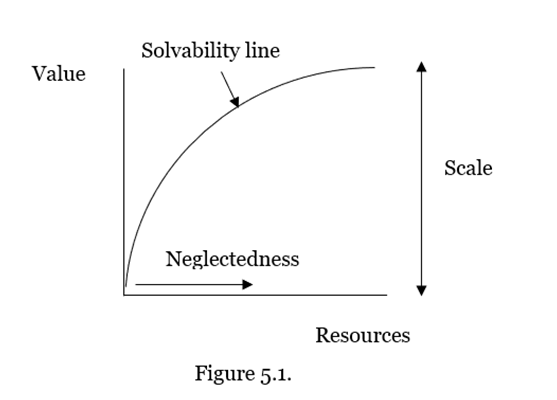
Third, we need to know how many resources are being directed at the problem, which is what neglectedness captures. Imagine two identical problems, A and B, with the same solvability line. Suppose no one will try to solve A but many people will try to solve B. Assuming people will pick the low-hanging fruit first, then the cost-effectiveness of additional resources to B will be lower than A. The way we’d represent that on the graph is pushing along the X-axis the point at which we start counting marginal resources. This fact is illustrated in figure 5.2. overleaf.
Thus, we need to know the scale, solvability and neglectedness in order to correctly locate both ‘where on the curve we are’, so to speak—that is, where we should start counting the effectiveness of our marginal resources from—and where we would get to for the additional units of resources we contribute. If we know the three factors, we can determine the cause’s priority (its cost-effectiveness). Note, however, that if there were constant marginal returns, i.e. cost-effectiveness was linear, we would only need two pieces of information to determine cost-effectiveness: first, the scale of the problem; second, a single assessment of solvability, i.e. the absolute number of resources required to solve X% of the problem.
Let’s state the answers to the third and fourth questions posed earlier (what role do the three factors play? Why there are three factors (rather than, say, four) and why these particular factors are used?). To the third, the response is that the three factors are multiplied together to determine the cost-effectiveness of a cause; to the fourth, our goal is to determine cost-effectiveness, and these three are individually necessary and jointly sufficient for that task, hence the factors allow us to meet that goal.
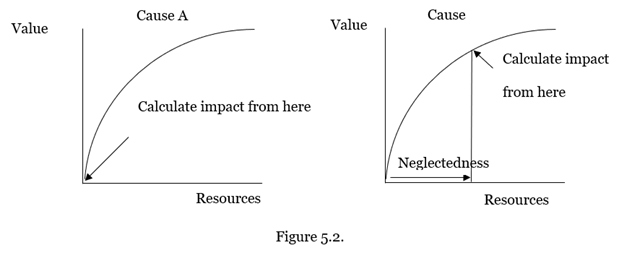
4. Distinguishing problem evaluation from solution evaluation
We haven’t yet got to the bottom of the first two questions (what’s the distinction between cause prioritisation and intervention evaluation? What reason is there for engaging in the cause prioritisation in the first place?). In this section, I argue the distinction between stage B of cause prioritisation and intervention evaluation, if there is one, is very thin.
Suppose that we want to determine which of two causes, X and Y, is the priority. What we need to do at stage B of cause prioritisation is to plug in some numbers for each of scale, solvability and neglectedness and see what comes out the other end. But it’s unclear there is any way to do this without considering, implicitly or explicitly, particular solutions to the problems at hand. Where else could the numbers come from? More specifically, as we’re trying to do the most good, the relevant comparison is between the best solutions we are aware of for each problem, as opposed to (say) the median solution to the problem. The quality of our analysis will depend on our inputs to the formula being realistic, hence we want to have actual, particular solutions in mind, even if we are just intuitively weighing these up in our heads. Hence, what we’re doing is evaluating particular solutions to given problems. Yet, intervention evaluation is the process of evaluating particular solutions to given problems, so stage B of cause prioritisation isn’t a distinct process.
We might think that although two processes are answering the same question—how cost-effective is a particular solution to a problem?—these are nevertheless somehow distinct. This is perhaps because we don’t have to consider the whole problem (scale) when we evaluate solutions, or the resources going to the problem (neglectedness). But notice that when we evaluate interventions, if you know the cost to solve a given fraction of the problem and the value of solving that fraction, it’s trivial to extrapolate those and work out the problem’s scale; further, to determine the counter-factual impact of the solution, you do have to consider the resources that are going to the problem anyway.
Even though we may think we are referring to two different processes—stage B of cause prioritisation and intervention evaluation—both require the same inputs. What’s more, not only do both produce an output in the same terms—good done per additional unit of resources—we’ll presumably get the same answer whether we think we’re doing one or the other: it would be very strange if our cost-effectiveness estimate of contributing extra resources to a problem is not identical to our estimate of how cost-effective the best solution(s) to that problem is. As a further indication these things are not distinct, notice that we could easily relabel any of the figures I introduced as representing the cost-effectiveness of causes, as representing the cost-effectiveness of interventions.
I imagine an advocate of the EA method might try to insist there is a meaningful distinction between stage B of cause prioritisation and intervention evaluation. There are two distinctions we might draw. First, the former is done intuitively—the three factors are combined in the head to make comparisons—whereas the latter necessarily involves making explicit, quantitative cost-effectiveness assessments. Second, the former is an assessment of the best solution to the problem, whereas the latter is any assessment of some solution to a problem.
We can grant these distinctions, but it is still the case that stage B of cause prioritisation and intervention evaluation both consist in the same task: evaluating particular solutions to problems. Hence, if we assumed there was some deep difference between them, we are on thin ice. I note the second distinction is somewhat awkward: what follows from it is that if we use the three-factor framework to determine the cost-effectiveness of a problem and do this in our heads, it’s ‘stage B of cause prioritisation’, but if we get out our calculators we’re suddenly doing ‘intervention evaluation’. Note that even if we do stage B in our heads, it is still a quantitative comparison of marginal cost-effectiveness that we are making.
What this analysis suggests is that we should conceptualise the EA method for setting priorities as having three steps:
1. ‘Screen out’ problems where it’s clear all their solutions are cost-ineffective. This is done by appealing to one or more of scale, neglectedness, and solvability individually.
2. Make an intuitive cost-effectiveness evaluation of the most promising solution(s) to each problem. This is done by combining scale, neglectedness and solvability.
3. Make explicit, quantitative cost-effectiveness evaluations of particular solutions to problems.
These steps map onto (what I’ve called) stage A of cause prioritisation, stage B of cause prioritisation, and intervention evaluation, respectively.
We might have thought that evaluating causes consists only in assessing the problem as a whole; that is, all possible solutions to it. What follows from the preceding analysis is that once we’ve done the initial screening step and are trying to determine the relative priority of the causes that remain—i.e. how cost-effective resources will be in each—that analysis rests on an assessment of the cost-effectiveness of a particular solution(s) to the problem. Hence, while it may be more natural to make claims such as “poverty is a higher priority than climate change”, it would be more accurate, though less elegant, to say instead “the best solutions to poverty we are aware of are more cost-effective ways of doing good than the best solutions to climate change we are of”.[8] When phrased this latter way, it’s clear that the cost-effectiveness of the solutions is doing the work in determining which of the problems is deemed the priority. Two concerns follow from this.
The first worry is that although the scale-neglectedness-solvability framework seems very sophisticated, it is just assessing the cost-effectiveness of particular solutions; our analysis here is only as good as the information we put in. Hence, if we have overlooked excellent solutions or wrongly estimated the cost-effectiveness of those solutions we (intuitively) considered, we will be mistaken about which problems are higher priority. If we thought that cause prioritisation via the three-factor framework resulted in a more holistic evaluation of the problem, we would not have this worry.
The second concern compounds the first. The EA method invites us to divide the world up into cause ‘buckets’, look for the best item in each bucket, compare what we’ve found, throw away the buckets that seem to have nothing valuable in them, and then look further in the buckets that remain. The issue with this is that is if we have thrown away a bucket because we overlooked something valuable, the method discourages us from looking in that bucket again. The risk is that causes are determined to be low-priority prematurely: if we’d have looked for potential solutions longer, a good one would have been found. In the previous chapter, I argued mental health had been overlooked; this is perhaps part of the explanation.
The practical upshot of the analysis is as follows: if we want to find the most cost-effective ways to do good, and be confident we haven’t overlooked the best options, we need to get stuck into the particular things we can do to make progress on each problem. Incorrectly believing that we can just take a quick look at a problem, consider its scale, neglectedness, and solvability, and thereby gain an accurate picture of a problem’s cost-effectiveness, is liable to lead us to overlook things. It’s these concerns which motivate, in the next chapter, proposing and testing a new approach to cause prioritisation that aims to overcome this issue.
To be clear, I’m not claiming these concerns are ones that (sophisticated) effective altruists are unaware of, or they demonstrate that effective altruist’s prior prioritisation efforts are systematically mistaken and they must go back to the drawing board.[9] I am merely noting the concern and highlighting the limitations of the methodology.
It’s unclear if this proposed reconceptualisation is surprising or not. On the one hand, what I suggest seems to be in tension with comments others have made on this subject. For instance, Michael Dickens writes:
The [three-factor] framework doesn’t apply to interventions as well as it does to causes. In short, cause areas correspond to problems, and interventions correspond to solutions; [the three-factor framework] assesses problems, not solutions.” (emphasis added)[10]
Hence, Dickens implies that it is possible to assess problems without assessing solutions at all, from which it would follow that cause prioritisation (stages A and B) is really distinct from intervention evaluation. As argued earlier, it’s unclear how this could be possible.
Robert Wiblin says of the three-factor framework that:
This qualitative framework is an alternative to straight cost-effectiveness calculations when they are impractical […] In practice it leads to faster research progress than trying to come up with a persuasive cost-effectiveness estimate when raw information is scarce […] These criteria are 'heuristics' that are designed to point in the direction of something being cost-effective.[11]
If the framework which we use for cause prioritisation is qualitative, then it must be distinct from the quantitative cost-effectiveness estimates we make of particular interventions. What seems to be going on here is that Wiblin is referring to the older, qualitative version of the three-factor framework I mentioned in the previous section. The newer version—the one we’re using—is a quantitative framework where the factors do combine to produce ‘straight cost-effectiveness calculations’ and so the distinction between using the framework and making cost-effectiveness analyses of interventions disintegrates.
More generally, it is somewhat surprising that no one seems to have made it explicit that the priority-setting process should be broken into (at least) the three steps I have suggested. We might have expected someone to offer a clarificatory description of the EA method along the following lines: “first, we ignore the problems with no good solutions; then, we make intuitive judgments of how cost-effective the best solution to each of the remaining problems is; finally, we make some explicit, numerical estimates of those solutions to check our guesses”.
On the other hand, I don’t think anyone has explicitly denied priority-setting can work in the way I have just stated. Indeed, it seems clear, on reflection, that this is how we can and should approach the task. When we decide where to go on holiday, I presume most people make rough judgements about how much they want to visit entire countries, then consider particular in-country destinations in more detail and, at the final stage, compare prices etc. for the different options.
As a further comment against this being surprising, my analysis seems at least compatible with MacAskill’s. In the opening quote, MacAskill states the three-factor framework allows us to make comparisons between causes even if we lack numerical cost-effectiveness assessments. What MacAskill is perhaps claiming is that the scale-neglectedness-solvability framework allows us to do step 2—make an intuitive comparison between problems—without having to go as far as step 3—making explicit quantitative cost-effectiveness assessments; his use of the word ‘heuristic’ seems to support this interpretation. As it happens, it seems plausible that we can and do use three-factor framework in our heads to construct cost-effectiveness lines for different problems: we think about the size and shape of the curve and adjust where we are on the curve to account for what others will do.
As such, it’s not obvious whether I am suggesting something different from what others thought of making explicit something that was previously implicit.
5. Conclusion
I began this chapter by asking: ‘what’s the distinction between cause prioritisation and intervention evaluation?’ This was motivated by the apparently odd suggestion that we can do the former before the latter. I’ve argued there is a sense in which we can evaluate causes(/problems) as a whole: if and when we can evaluate all their solutions – this was stage A of cause prioritisation. If intervention evaluation requires the assessing of particular solutions in some depth, then stage A of cause prioritisation can happen prior to intervention evaluation. Both stage B of cause prioritisation and intervention evaluation require the cost-effectiveness of solutions to a given problem to be determined. If we stipulate that stage B of cause prioritisation can be done intuitively, whereas intervention evaluation requires the writing down of some numbers and then crunching those, the former can be also prior to the latter; I noted this distinction is pretty flimsy. If we don’t stipulate this ‘in-the-head’ vs ‘on-paper’ distinction, then the two are the same process and hence stage B of cause prioritisation is not prior to intervention evaluation.
The second question related to why we should engage in cause prioritisation rather than leap straight to intervention evaluation. Following what we just said in the last paragraph, if we can evaluate causes as a whole—i.e. what happens at stage A—that can usefully save time. We can now see there’s not much difference between starting at stage B of cause prioritisation or with intervention evaluation in any case.
In this chapter, I’ve set out to address some of the outstanding questions about how the EA priority-setting method functions. What I’ve suggested here is a modest reconceptualisation of the EA method. I have not tried to argue the EA method is mistaken in some deep way, because it is not. Rather, I have tried to clarify something that seemed plausible but the details of which were murky. A practical conclusion emerged from the reconceptualisation: while we might have thought the three-factor cause prioritisation method assessed problems ‘as a whole’, this is only partially true: once we’ve sifted out unpromising problems (ones with no good solutions), our analysis of how problem A compares to problem B is nothing more and nothing less than a comparison of the best solutions to problems A and B that we’ve considered. Hence, if we want to find the most cost-effective ways to do good, we need to look carefully at these solutions.
Endnotes
[1] (MacAskill, 2018)
[2] ‘Scale’ has been called ‘importance’, ‘neglectedness’ called ‘(un/)crowdedness’, and ‘solvability’ called ‘tractability’. For different uses see, e.g. (MacAskill, 2015) chapter 10, (Cotton-Barratt, 2016), (Open Philanthropy Project and Karnofsky, 2014).
[3] After coming to realise this reframing myself, I subsequently discovered it had previously been used by (Dickens, 2016).
[4] If clarity is needed, I mean all the solutions that are actually possible, as opposed to, say, metaphysically possible.
[5] Except, I suppose, very small countries.
[6] I believe (Cotton-Barratt, 2016) was the one who first identified how the factors could be combined to assess cost-effectiveness. EA career’s advice service (80000 Hours, no date) also uses this quantitative version of the framework.
[7] This is version is used by (MacAskill, 2015) in chapter 10 and (Wiblin, 2016). It seems to have been originally proposed by (Open Philanthropy Project and Karnofsky, 2014). In conversation, Hilary Greaves suggests that this version is arguably implicitly quantitative in nature, or at least used in that way: individuals applying the framework would assume something like the following: if cause A was X% larger in scale but Y% less neglected than cause B, the two causes would be equally cost-effective at a given ratio of X:Y. It might be true that framework has been in used this way, but given that no explanation has been offered as to why the factors could be traded-off like this, or what the appropriate ratios are, it’s hard to see what justification there could be for using this framework to set priorities as opposed to just making educated guesses about the cost-effectiveness of particular interventions.
[8] Peter Singer asks whether a claim such as “poverty is a higher priority than opera houses” requires us to compare the best solutions in each case. I do not think we need to think carefully about the available solutions in each to defensibly make such a claim, but it still seems that we are (implicitly) appealing to the relative cost-effectiveness of the top solutions. To illustrate this, note how odd it would be to claim “poverty is a lower priority than opera houses”, even if one thought the top poverty solution was more cost-effective than the top opera house solution, simply because there are some ways of doing good via opera houses than are more cost-effectiveness than some solutions to poverty.
[9] I say this despite the fact that, in the next chapter, I go back to the drawing board to (re)consider what the priorities are if we want to make people happier during their lives. This is, however, a combination of both (a) wanting to create and test a different prioritisation method and (b) because, as argued in chapter 4, I think effective altruists have been using a non-ideal measure of happiness and this alone prompts a reevaluation of the priorities.
[10] (Dickens, 2016)
[11] (Wiblin, 2016)
Bibliography
80000 Hours. (n.d.). How to compare different global problems in terms of impact. Retrieved June 26, 2017, from https://80000hours.org/articles/problem-framework/
Cotton-Barratt, O. (2016). Prospecting for Gold. Retrieved April 17, 2019, from https://www.effectivealtruism.org/articles/prospecting-for-gold-owen-cotton-barratt/
Dickens, M. (2016). Evaluation Frameworks (or: When Importance / Neglectedness / Tractability Doesn’t Apply). Retrieved January 14, 2018, from http://mdickens.me/2016/06/10/evaluation_frameworks_(or-_when_scale-neglectedness-tractability_doesn’t_apply)/
MacAskill, W. (2015). Doing Good Better. Faber & Faber.
MacAskill, W. (2018). Understanding Effective Altruism and Its Challenges. In The Palgrave Handbook of Philosophy and Public Policy (pp. 441–453). https://doi.org/10.1007/978-3-319-93907-0_34
Open Philanthropy Project, & Karnofsky, H. (2014). Narrowing down U.S. policy areas. Retrieved January 14, 2018, from https://blog.givewell.org/2014/05/22/narrowing-down-u-s-policy-areas/
Wiblin, R. (2016). The Important/Neglected/Tractable framework needs to be applied with care.
Do you think there's more useful research to be done on this topic? Are there any specific questions you think researchers haven't yet answered sufficiently? What are the gaps in the EA literature on this?
Hello Risto,
Thanks for this. That's a good question. I think it partially depends on whether you agree with the above analysis. If you think it's correct that, when we drill down into it, evaluating problems (aka 'causes') by S, N, and T is just equivalent to evaluating the cost-effectiveness of particular solutions (aka 'interventions') to those problems, then that settles the mystery of what the difference really is between 'cause prioritisation' and 'intervention evaluation' - in short, they are the same thing and we were confused if we thought otherwise. However, if someone thought there was a difference, it would be useful to hear what it is.
The further question, if cause prioritisation is just the business of assessing particular solutions to problems, is: what the best ways to go about picking which particular solutions to assess first? Do we just pick them at random? Is there some systemic approach we can use instead? If so, what is it? Previously, we thought we have a two-step method: 1) do cause prioritisation, 2) do intervention evaluation. If they are the same, then we don't seem to have much of a method to use, which feels pretty dissatisfying.
FWIW, I feel inclined towards what I call the 'no shortcuts' approach to cause prioritisation: if you want to know how to do the most good, there isn't a 'quick and dirty' way to tell what those problems are, as it were, from 30,000 ft. You've just got to get stuck in and (intuitively) estimate particular different things you could do. I'm not confident that we can really assess things 'at-the-problem-level' without looking at solutions, or that we can appeal to e.g. scale or neglectedness by themselves and expect that to very much work. A problem can be large and neglecteded because its intractable, so can only make progress on cost-effectiveness by getting 'into the weeds' and looking at particular things you can do and evaluating them.