I gave a talk at EAG SF where I tried to describe my current favorite plan for how to align transformatively powerful AI, and how I thought that this plan informs current research prioritization. I think this talk does a reasonable job of representing my current opinions, and it was a great exercise for me to write it.
I have one major regret about this talk, which is that my attitude towards the risks associated with following the proposed plan now seems way too blasé to me. I think that if labs deploy transformative AI in the next ten years after following the kind of plan I describe, there's something like 10-20% chance that this leads to AI takeover.
Obviously, this is a massive amount of risk. It seems totally unacceptable to me for labs to unilaterally impose this kind of risk on the world, from both the longtermist perspective and also from any common-sense perspective. The potential benefits of AGI development are massive, but not massive enough that it’s worth accepting massive risk to make the advent of AGI happen a decade sooner.
I wish I'd prefixed the talk by saying something like: "This is not the plan that humanity deserves. In almost all cases where AI developers find themselves in the kind of scenario I described here, I think they should put a lot of their effort into looking for alternative options to deploying their dangerous models with just the kinds of safety interventions I was describing here. From my perspective, it only makes sense to follow this kind of plan if the developers are already in exceptionally dire circumstances which were already a massive failure of societal coordination."
So if I think that this plan is objectively unacceptable, why did I describe it?
- Substantially, it's just that I had made the unforced error of losing sight of how objectively terrible a 10-20% chance of AI takeover is.
- This is partially because my estimate of x-risk from AI used to be way higher, and so 10-20% intuitively feels pretty chill and low to me, even though obviously it's still objectively awful. I spend a lot of time arguing with people whose P(AI takeover) is way higher than mine, and so I kind of naturally fall into the role of trying to argue that a plan is more likely to succeed than you might have thought.
- I realized my error here mostly by talking to Ryan Greenblatt and Beth Barnes. In particular, Beth is focused on developing safety standards that AI labs follow when developing and deploying their AI systems; plans of the type that I described in this talk are probably not able to be robust enough that they would meet the safety standards that Beth would like to have in place.
- I think of my job as an alignment researcher as trying to do technical research such that AI developers are able to make the best of whatever empirical situation they find themselves in; from this perspective, it’s someone else’s job to reduce the probability that we end up in a situation where the alignment techniques are under a lot of strain. And so the fact that this situation is objectively unacceptable is often not really on my mind.
Since this talk, I’ve also felt increasingly hopeful that it will be possible to intervene such that labs are much more careful than I was imagining them being in this talk, and I think it’s plausible that people who are concerned about AI takeover should be aiming for coordination such that we’d have an overall 1% chance of AI takeover rather than just shooting for getting to ~10%. The main levers here are:
- Go slower at the end. A lot of the risk of developing really powerful AI comes from deploying it in high-leverage situations before you’ve had enough time to really understand its properties. I would feel substantially safer if instead of labs engaging in explosive economic growth over one year, they did this automation of R&D over the course of a decade.
- Have much better arguments for the safety of your AIs, rather than relying on the coarse empirical evaluations I describe in this talk. This could come by various techniques, e.g. digital neuroscience techniques, or development of techniques where we have theoretical arguments for why they will scale to arbitrarily powerful systems (e.g. the work that ARC does).
So when reading this, please think of this as a plan designed for fairly dire circumstances, rather than an example of things going “well”.
That said, even though the plan I described here seems objectively quite unfortunate, I still think it’s likely that something even riskier will happen, and I think it’s worth putting effort into ensuring that AI developers follow a plan at least as good at this one, rather than entirely focusing on trying to get AI developers to have access to a better plan than this.
I've recently been spending a bunch of my time (with Ryan Greenblatt and a bit of Nate Thomas) trying to come up with a much more fleshed-out proposal for how we'd recommend aligning really powerful models if we had to do so right now. I'm hoping that in the next month or two we've written something up which is a much better version of this talk. We'll see if that ends up happening.
You can see the slides for this talk here
Here's the full transcript with slides:
Good morning, everybody. Well, I would like to talk about what I think we would try to do, ideally, if AI happened very soon, and then talk about how I prioritize alignment research based on this kind of consideration. It's going to be a good time.
Introduction
Just to summarize before I get into it, what is going to happen in this talk. We're going to have two parts. In the first part, I'm going to be talking about the situation that I'm mostly thinking about when I'm thinking about alignment research and what we need from alignment techniques in this situation. I'm going to say what kind of things I think we, just in practice, would use if we tried to do a pretty good job of it pretty soon. And then how I think those might fail.
In the second half of this talk, I'm going to talk about how I use thinking about the nearcast to assess how much I like different technical alignment research directions.
You should submit clarifying questions and complaints to Swapcard as we go, and I will take them as I want to.
All right, acknowledgements. I'm cribbing heavily from Holden Karnofsky's ‘deployment problem’ analysis, and some of his other writings. He doesn't agree necessarily with stuff that I say, and I disagree with him on some of the stuff he says, so ... That's an acknowledgement, but not a mutual endorsement.
Thanks to Ajeya [Cotra] and Ryan [Greenblatt] for a bunch of conversations here.
Oli Habryka had a complaint that I really appreciated about the title of this talk: he complained that it made it sound more like the thing I was proposing is consensus about what we should do for AI alignment, than I think it in fact is. So just to be clear about the extent to which I think this talk is (a) normative versus descriptive and (b) consensus. The things I'm going to claim about what would happen, and what should happen are, I think, not very controversial among people who do empirical AI alignment research because they care about AI takeover at large labs. I think they're a lot more controversial among Alignment Forum users. I think for a lot of this, my best guess is that a lot of the things I think we should do about AI alignment when AGI is near will be quite obvious. And so I'm kind of going to blend between talking prescriptively and descriptively, and hopefully this doesn't induce confusion. Great, let's go.
The nearcast
Okay, so the nearcast.
The situation I’m imagining
Here's the situation that I spend my nights worrying about, the situation which I am trying to make go better. I'm interested in the case where there's some company – Ajeya suggested using the name Magma for this hypothetical company – that is close to being able to build and deploy AI that if they deployed it, would be a massive deal, would be transformative AI, or TAI as we're going to call it. And they are in a situation, where if they don't deploy their transformative AI, if they don't use it somehow, there is a large risk of a catastrophe caused by AI systems deployed by other actors.
And so there's this natural spectrum of plans, where one type of plan is: don't use your AIs at all. And you're unhappy with those plans, because if you try one of the plans where you just don't use AI at all, you think you're going to get killed by someone else.
And then on the other [end of the] spectrum, if you just use AI as much as you possibly could, try to use AI to its fullest potential, in whatever way will cause the AI to be most capable, you're worried that the AI will successfully execute a coup.
And so there's kind of this trade-off between inaction risk, where you don't do anything, and then something really bad happens because of someone else, and accident risk, where you used AIs in ways that weren't actually safe.
One of the big themes of this talk is going to be thinking of plans in terms of spectrums, and thinking of the trade-offs between different places you might end up. I already said the thing about prescriptiveness.
Maybe one more specific thing to say here about this situation: I think of my job as causing a particular one- or two-year interval to go well. From my perspective, there's going to be this one-year or two-year interval where AI, at the start of those two years, is not very important. And at the end of those two years, it's complete crazy town, and the economy is doubling every month or something.
From my perspective, my job is not to worry about bad stuff that happens before that two-year period, because I don't think it's that important. I don't think AI is going to mess up the world that drastically before this particular crucial period, where AI becomes extremely powerful. And afterwards, I just think the situation's out of my hands. At the point where the world economy is doubling every month or something, and you know the robots are building all these insane space factories or whatever, I'm just like, “I can't help anymore”. At this point, you know, the AIs are going to be making so many decisions so quickly that I have no chance of keeping up with it. The only chance I have of my values being preserved is if I've already ensured that there are AIs who are taking care of me, like an old person becoming senile or something and slowly losing mental capacities, and having to make sure they have caretakers. In that case, the person's getting weaker, but in this case, it's more like the rest of the world gets crazier.
So I'm like, my job is just this two-year period. When you mention a problem to me, I'm like, "Did it happen in that two-year period?" And if it didn't, I'm like, "That's someone else's problem." And this is, to some extent, me just choosing a thing to focus on. I think there are some concerns that I have about AI before the transformative time. But empirically, that's just not the situation I focus on.
What the AI might help us with
Why might this company Magma be in a situation where they really think that they need to deploy some AI? So here are some things that they might need to do, that they might not be able to do without getting the AI to help them.
So one option here is alignment. One obvious thing you might need to do with really powerful AIs is get much better at aligning yet more powerful AIs. I think one possibility here is more like alignment research, where you're like… you know, currently I try to do alignment research. But unfortunately, I can only do so many things in a day. If I had AI assistants who were very competent at helping me with research, my research would proceed a lot faster. And so, alignment research is one kind of thing you might wanna do.
The other thing that you might wanna do is more like [actually doing alignment], or alignment contracting, or something. A lot of work which currently goes into aligning systems is not research, it's more like ‘reviewing a bunch of things the AI said, and seeing if any of them were bad’, and I think this is not well-described as research. And I think that you probably also want your AIs doing things like reviewing a bunch of plans produced by other AI systems, and seeing whether anything in them seems sketchy or bad.
Some other things you can imagine your AI helping you with: you can imagine it helping enforce agreements on how AI is developed by proposing those to governments or arguing for them, or building technology such that it's easier to enforce them. For example, if you really wanna make it the case that no one is allowed to develop models that are bigger than a certain size without passing some set of alignment evals, one difficulty here is how technically do you ensure that no one has enough compute to train those models? You can imagine wanting your AI to help you with this.
Another thing you might want powerful AIs to help you with is making it harder for unaligned systems to wreak havoc. So the simplest example here is: currently, there are definitely a bunch of computer security problems. Everyone's browsers, everyone's operating systems have a bunch of computer security problems. If an unaligned AI showed up right now, they could pwn everyone, and cause a lot of problems by stealing lots of people's money. It would be much more difficult for an unaligned AI to cause trouble if computer security were much better, and so one thing you might be tempted to do with an aligned AI or with AIs that you could use for whatever purposes you wanted is to try to harden everyone's computer security, such that when other unaligned AIs appear, they have a harder time causing trouble, and cause fewer problems.
Eating the low-hanging fruit is kind of another example here. Online poker is a classic way of making money if you're kind of smart and know how to use a computer. I mean, this one has actually mostly already been done by bots. But suppose your threat model was ‘someone's going to build an unaligned AI, and then it's going to play a bunch of online poker, and make a bunch of money’. One thing we can do… this example is kind of lame. One thing we could do is use our aligned AIs to play a lot of online poker first, and already eat the low-hanging fruit. It's like the unaligned AI shows up, and it's like "Oh man, how am I going to make all this money, so that I can buy computers to run more copies of myself, so that I can make paperclips? Oh maybe I should try playing online poker. Oh no, there's already this super competitive market in AIs playing online Poker."
Maybe a better example is other kinds of online assistant things that are more like productive activities in the economy, like proofreading, or writing SEO spam or whatever. I'm just like: if our aligned AIs are already crushing this, making it a super competitive market to do AI-generated SEO spam, the unaligned AIs will have a lot more trouble making money to buy computers given that the prices have already been knocked low. Another thing you can imagine doing is just using powerful systems to help you detect whether other people are building aligned AIs. It's just a thing you expect would be easier if you had a bunch of AIs do a bunch of cognitive labor. So the claim here is basically, we might be in a situation, where we have these AIs, if they were aligned we would be able to use them to do stuff like this, and then we would no longer be very worried about X-risk from unaligned AIs, but we aren't totally sure that our AIs are aligned, and we aren't totally sure how to align our AIs, and so we have this trade-off between not doing the stuff that we need to do in order to not get killed by unaligned AIs, and getting killed by our own AIs.
The v0 plan
So, let's talk about the v0 version of the plan. In machine learning, as I'm going to assume you know, the basic shtick is: we look for models which do well according to some function evaluating their output. The most obvious way that we would try to build really powerful AGI right now is, you probably train a model on a mixture of tasks. You probably take some task, where it's very cheap to train the model on this task. When you train the model on this task, it learns lots of stuff.
The classic example we use right now is pre-training on the internet. No one actually really wants an AI that is really good at guessing what the next piece of some text from the internet was. But the internet does have a lot of text on it, and so it's extremely cheap to train it. Per dollar, one of the best ways to make a very capable system that knows a lot of stuff about the world right now seems to be training it on this next token prediction task on the internet.
But it's almost never going to be the case that the cheapest task to train your model on to make it smart is the same as a task that you care about. So you're almost always going to wanna train your model on a mixture of tasks. One of which is cheap, to produce capabilities with, and the other one of which is what you actually care about doing.
Right now the way that this plays out is: we pre-train our language models on auto-regressively predicting the internet text and then we fine-tune them based on the extent to which they match human demonstrations of being helpful, or how much humans like the outputs they have. So you know, for instance ChatGPT or Bing's new chatbot, which is named Sydney apparently, were both trained on the whole internet, at which point it is not super useful for very much stuff. And then you give it a bunch of examples of how to be a helpful chatbot, ask it to try to be a helpful chatbot a bunch of times, pay your humans to upvote or downvote whether that was in fact a good answer to the human's question. And then you get this model, that's actually pretty capable.
It might be the case in general that the task that you used to make your model be smart, and the task that you cared about would be the same task, but from my perspective, that would be a kind of weird coincidence. I just don't really see why it would be the case that these tasks would be the same.
So, this here is entirely descriptive. I'm just like: look, if AGI was getting really powerful next year, and people were like "How do we make as much money as possible?" – which is a question people love asking – they would probably use a plan something like this.
The basic problems with the v0 plan
Here are my problems with this plan. Problems with this plan have to be of the form… The hypothesis is, the model looks good at the task we care about, but nevertheless something really bad happens. So it has to be the case that the model was looking good according to our evaluations of how well it was doing at that task, but it still led to AI takeover. It's still engaged in doing an AI coup. And it feels to me like there's basically two possibilities for why things went wrong.
Firstly, perhaps when we were evaluating it, we were evaluating it poorly. The answers that it was suggesting - we thought that they were fine answers, but actually they were answers that were manipulating us. Therefore the problem was our evaluation was too weak. The full definition here I would use is: you have a problem if your model knows things about the outcomes of its own actions, that you don't know when you're evaluating it, because if there's a difference of knowledge there, the model is incentivized to do things that manipulate you in sad ways.
So the other possibility is, maybe even though during training we were very good at evaluating how good the model’s outputs were, it did something catastrophic in deployment, but not during training. Holden Karnofsky calls this the King Lear problem.
I hadn't actually known anything about the plot of King Lear before this blog post of his, perhaps because I'm, you know, uncultured. But the story of King Lear apparently is in the start of the story, King Lear is this king, and he has these daughters. He is trying to decide which daughter to trust his kingdom to, and two of them are really obsequious. They act as if they'll be really great at being in charge of the kingdom. And this other [daughter] is more honest. The King selects the daughters who are being really obsequious and acting as if they really love him. Then they actually have played him for a fool, and when they have the power, they do stuff he doesn't like.
The basic point of the story is, suppose you want to assess someone to answer the question, “What will they do post-assessment, when you've already entrusted them with lots of power?” It's just structurally quite difficult to answer that kind of question because they have this amazing strategy available to them of ‘behave really aligned and then do something else when they actually have all the power’. Okay, so that was the v0 plan. I think people would pretty plausibly try this v0 plan. I think they would pretty plausibly notice these problems. We maybe already have noticed some of these problems. The catastrophe one we don't notice super much, because the models aren't smart enough yet to think of doing that, I think. But you know, maybe soon.
v1 Plan
So I'm going to talk about the v1 plan, which is the basic improvement on the previous plan. So this is mostly super simple stuff.
Problem 1: maybe we were evaluating poorly. Proposed solution: evaluate better. How might we evaluate better? Well, we're going to have a bunch of AIs around. Maybe we should use those AIs to help us evaluate better. This is a very obvious idea. I think that if no alignment people had pointed out this idea, I think it would've been obvious to approximately everyone. It's like, if you were asking me to evaluate a bunch of AI outputs, it wouldn't even occur to me to not use Google when evaluating whether its answers were good and correct and stuff, because I just think of Googling facts to see if they're true as a basic action that I always take.
Similarly, my guess is that in the future when people are evaluating how good their AI’s answers are, they're going to be so used to using ChatGPT or whatever to help them answer questions, that of course they're going to use the AIs they currently have to try and do a better job of evaluating the AIs they're currently training. So a bunch of stuff has been done in this space.
You might be like, "Great! Is there a theorem, that as long as you have access to the AI you're training while you're training it, everything goes fine?" And the answer is no. Basically for this kind of plan to work in general, you have to have some guarantees that everything grounds out in a particular way that maybe doesn't ground out in reality. My position here is: I don't know whether the basic question is like, “do you end up knowing everything you needed to know about the outcomes of the model's actions, given that you're also asking the model questions while you're doing the evaluation?” And my best guess right now is you definitely don't know everything, but you also probably do know a lot of stuff. Is this problem bad enough that you get killed? I'm not sure.
So to address the like King Lear problems, or the model occasionally doing terrible catastrophes, the most obvious thing from my perspective to try to do is, as well as training the model on a bunch of natural inputs, you try really hard to construct inputs where the model really thinks that it has a good opportunity to take a catastrophic action. Basically, for literally the King Lear problem, one thing you can imagine him doing is being like, "Great, daughters, it's the day where I've transferred the power to you. Congratulations, I'm so excited to see what you do." And then he waits a week, during which they don't actually have the power. He's just watching to see what stuff they do, believing they have the power. And then if he's unhappy with the stuff they do, he's like, "Great, I have assessed that you are bad daughters for being kings.”
Roughly speaking, I think the same thing is possible with AIs, though it's actually way easier for us, because we control all of their input. They're just programs on our computer, so it's very easy for us to try running, having ... Oh man, there is a certain famous piece of fan fiction where someone asks questions to someone in a whole bunch of different ways, iteratively learning how to get better and better at asking, persuading them to do something, and eventually they persuade them, because of the fact that they were able to use trial and error to ask the questions a whole bunch of times, and I'm just like, we get to do the same thing with our AIs. We get to iterate really hard on trying to come up with inputs where they really think that they can get away with doing a coup right now. And I think that actually probably makes it a lot harder for the AIs to stage coups when they have opportunities to.
Okay, so again we could ask the theoretical question of: in theory, who wins the game, where I am trying to construct fake situations, where you have the opportunity to do a coup, and you are trying to detect whether a situation is a fake coup opportunity or a real coup opportunity? And unfortunately, because of P /= MP reasons, you win: it's easier to detect whether a situation has been faked, than to construct fake situations.
And so again we find ourselves in the situation where the alignment problem… the situation seems like empirically maybe we're just fine, like maybe we win the game of trying to construct realistic coup opportunities, but we don't have theoretical guarantees, and so this rests on empirical contingencies, which is a little regrettable in my opinion.
Some other stuff we're going to do: super simple stuff. When you are asking your AI to make decisions that are particularly cruxy, or the kinds of decisions where bad answers are more plausibly going to lead to catastrophes, you should consider checking more carefully. If you were like, “well it would save me a million dollars to have the AI be in charge of the launch ordering, and it would save me a million dollars to have the AI be in charge of the nuclear launch codes”, I'm just like: maybe you should try putting the AI in charge of the former, and not the latter, on the margin, because it seems like that means there's fewer opportunities to have really bad things happen.
We should try a bunch of really obvious stuff that you would try if you wanted some humans to not do a coup. I think one kind of underrated strategy along these lines is whistle-blowing. I think we should probably, as well as training our model on a bunch of opportunities to start a coup, we should train our model on a bunch of faked situations where an AI coup is in progress: like the AI is like seven steps into taking actions that will lead to an AI coup, or it sees that another instance of itself has done something that might lead to a takeover.
So one example here is a classic concern I have about my AI is, you know, Magma, or whatever company is doing this, is probably going to be using its AI to write a bunch of software, and do a bunch of stuff in its data centers where it's running the AI, and something which seems really scary is: because the AI is writing so much code, you might have thought that it would be really easy for the AI to insert back doors into that code, insert security vulnerabilities into the code that it is writing, such that it can get out onto the open internet in ways that you didn't think it would be able to do, and you might initially be like "How could we possibly stop the AI from inserting security vulnerabilities into the code that's being run on this data center?"
One thing I would like to do is train it a whole lot on cases where you give it some code, which is AI-written, that has had subtle security vulnerabilities inserted, and then you train it to point those security vulnerabilities out to you. It seems quite hard for the AI to coordinate with itself to never point out cases that it notices where it has been in the process of starting a coup. So AI whistle-blowing: you just train the AI to, when it notices that it has been doing takeover-y activities, it sends you an email about them, and I think this might make it pretty hard for it to really get away with it.
[Buck answers some audience questions]
Some people asked about why I said two-year-periods as the thing that was my job. I'm just like, “Eh, this is just some empirical claim”. I'm just like: I bet that the problems are over roughly that time scale, it's just a guess about takeoff speeds or something. My guess is that the AIs don't have that much takeover capability, two years before the singularity, and they do afterwards, but I'm not super-strongly wedded to this.
v1 Plan Prognosis
Okay, moving forward, my prognosis on this plan, as I basically said, it's kind of like we're summoning unreliable eldritch spirits to harness their labor enough to solve some problem, but we don't want them to stage a coup.
The version of this that I've been thinking about recently is: we need like a trillion person-years of labor in order to solve our problems, and we have this ability to just summon these eldritch spirits, and a trillion person-years is a lot, you know, like that would require… you know, there's currently like six billion humans who do work in the world, maybe seven billion humans… no, there's more like six billion humans who do work, so if we wanted to do a trillion years of work in a year, we'd have to have 12 times the current workforce basically. That's a lot of intellectual labor going on, it just feels that by mass or something, it feels like they should be able to stage a coup, but we also have all these structural advantages, where we get to choose the organizational structure that they're being put in, we get to put them in all these insane training situations, all these insane evaluations.
I think one of the big questions here is how well these systems coordinate with each other. Currently, I'm actually feeling more optimistic after the last few months. I've been looking at Bing Chat. Bing Chat is kind of helpful (or Sydney as it apparently identifies). So Sydney or Bing Chat, seems like it would be quite helpful. It also just does not seem like it has… like, that it can keep it together long enough to do a long con, and it really seems to me like the core question here is: can your systems keep it together for long enough to pull a long con, before you have extracted enough labor from them, that you've solved all of your problems.
And I'm just like, well, Sydney is like a lot weaker than these systems are going to get, and so maybe the situation is going to be like: imagine a version of Sydney, who is a bunch more powerful, pretty plausibly still can't keep it together, and then we have like three months to do, you know, an enormous amount of intellectual labor, sped up enormously by this unreliable assistant. And I'm just like, how fine is this situation?
Yeah, I don't know. It seems kind of unlikely to me that Sydney coordinates with itself really well. Yeah. And we don't really have a good argument for why they wouldn't stage a coup, but also it seems like we can probably make their lives pretty hard.
A controversial take of mine compared to a lot of other alignment people: a lot of people seem to think that the deal is, you wanna make sure that you don't deploy AIs that want to kill you. From my perspective, I don't know if we're going to get that. I think we're plausibly fine, even if we deploy AIs that kind of wanna kill you, as long as they empirically fail to do so.
I mean, if you just look at the world, there's a lot of unpopular people who run countries. Vladimir Putin would lose a physical fight against the population of Russia, right? He maintains power through a complicated structure where a variety of people are individually incentivized, and tested, and it's just hard for anyone to stage a coup.
There's this book, The Dictator's Handbook, which is about how to prevent coups, and it's all about, you need to have some special forces, you need to separate out the different branches of the military, and you normally wanna make it so your army isn't allowed to enter your capital, and you have to make everyone suspicious of each other and constantly have it so that people in senior leadership roles are being approached by people who are implying that they should start a coup with them, and then you kill everyone who does.
And I'm just like: empirically many people get by even when leading large nations full of people who kind of hate them, and it's not clear that our situation is way worse than that situation, at least for the time period…like we just have to last in a situation like that for long enough that we can do the tasks that we thought would make the situation better. In my opinion, EA is underweighting how hard this [AI] coup-staging seems to be. I feel like their models would suggest that governments would look really different. My overall P-doom at this point is like 25%, so it fluctuates wildly from day to day.
A point that I wanna make here is: this situation does not seem clearly fine, though it also seems maybe okay. I understand this to be a somewhat controversial opinion compared to a bunch of other alignment people. Yep, that's where I'm at.
Far mode vs near mode
Okay, here's a thing from Twitter the other day from Agnes Callard, the person who talks to Robin Hanson a lot. A problem I have with EAs who talk about alignment… I'm sure some of you guys understand this picture: the question is “How do you pack 17 squares into a square?” and the answer is this kind of ugly thing on the right. From my perspective, a lot of people seem to think about AI alignment, and like what you do with your AI systems, and it's kind of upsettingly farmered, where they aren't seriously being like, "Okay, but how would the AIs kill you?”
Like they're going to insert these back doors, but you are going to be trying really hard to get AIs to look for back doors… The whole situation seems complicated. A lot of the interventions we can take feel like they're on like spectra, as opposed to ‘you do this or you don't do this’. I recommend thinking about the situation with a bunch of squares that are all askew. I think I personally regret thinking about it more like the thing on the left in the past.
One frame on Magma’s doom minimization problem
Okay, so one frame on Magma's doom minimization problem is, they're choosing where to spend their slack. Magma has some resources, and by assumption, Magma is able to operate somewhat less efficiently, because of the fact that they have alignment concerns. They aren't in this cutthroat race where if they don't use their AIs as capably and as powerfully as possible as soon as possible, they're just immediately going to lose. We assume that they have some surplus that they're able to spend, and the question that they'll be asking themselves, and that we want to make easier for them, is how to make these trade-offs. So I wanna name a bunch of trade-offs that they… a bunch of options they have.
So one option: they could do less in the world. We've already talked about this one: they could just do less with their AIs, they could take spare money, and spend it on something totally unrelated to AI, like paying humans to do computer security research, or to do hardening: this is in the action space.
They could, instead of training models that are as smart as they would train them if they had no slack… instead of training models that are the optimal level of intelligence to have them be capable, you could use dumber models, even though this means that it's more expensive to use your models because you have to spend more on inference, and they're just less capable.
You could use ML techniques, which are worse, but safer for some reason. You could spend some of your extra money doing more alignment stuff; you could spend money on more alignment evaluations of your models, you could spend more on technical alignment research, you could wield the intelligence of your models less efficiently.
What are my examples here? So by ‘wielding the intelligence of your models’, I mean doing a worse job of eliciting the latent capabilities of your models. So outcome-based training is where you train your models based on how much you think its actions look good to you. And so suppose you wanna have an AI that gives good answers to questions. One kind of thing you could do is pay humans to write a bunch of answers to questions, and train a model to imitate them. Another thing you could do is train a model to generate a bunch of answers to questions, and then train it based on how much you liked those answers to the questions, and the second kind of training is going to incentivize your model to lie to you in ways that humans wouldn't have lied to you, in a way that the first type doesn't. If you're just imitating humans, your models are not going to have any reason to lie to you because all they've been selected to do is do a good job of imitating the humans, whereas if they're being selected based on whether they answer questions in ways humans approve of, then they are going to, in cases where they can get a better evaluation by saying things that are false, they will be incentivized to do that.
In general, it's going to be more powerful to train your models in these outcome-based ways, because when you train models in outcome-based ways, this allows you to elicit knowledge the models have that humans don't have, but also it seems scarier. We can make trade-offs like that.
The next thing I wanna say [is] you can require your models to provide more legible reasoning for doing stuff. You can also choose tasks carefully. Instead of deploying your AIs on the things which are the most direct path to solving your problems, you can err on the side of having them do tasks where oversight is easier, or there aren't opportunities for catastrophic actions.
So for instance, the nuclear war example is an example of this. Maybe you should just not give your AI the nuclear weapon codes, even if it would be kind of convenient to do so, because that just seems like a particularly catastrophe-prone action.
Technical alignment research prioritization
So given that picture, how do we do good alignment research? Sorry, not how do we, how do I do the alignment research that I empirically do? I'm not sure this strategy is good, but I wanna tell you about how I use the previous reasoning about the alignment situation, to think about what research I should personally do.
So here's the question that I ask myself. Assume that a lab ends up in the situation described earlier and they're being as smart as possible about handling the alignment problems. How can I produce helpful changes to their alignment plan by doing technical research now?
My restricted-scope question about technical alignment research prioritization
Let's put that in a picture of a table. We made four assumptions/restrictions of scope. Firstly, we're assuming a lab ends up in that situation. The other possibility is that that situation never happens, or some more important thing happens instead.
We're assuming that they're being smart about handling alignment problems. The other possibility is the lab doesn't care about alignment problems because they're silly, in which case perhaps I should be spending my time ensuring the labs do more reasonable things. I assumed the alignment plan looks similar to what I described in the nearcast section. The other possibility is it looks very different. And another assumption was I should try to improve the situation by doing technical research now instead of something else. This is some mixture of comparative advantage considerations for myself, and thinking that things are actually more important than other things, it's a little hard to say which are which.
The ones of these that seem like most obviously a bad choice to me…or inasmuch as I'm making a mistake by doing these restrictions, my best guesses as to where I'm making a mistake are, I feel good about the first one, I feel not good about the second one, it seems really plausible that like ... Eliezer [Yudkowsky] has this line about ‘death with dignity’, we can talk about ‘high dignity’ versus ‘low dignity’ failures. The ‘high dignity’ failure is: we tried so hard to develop our amazing alignment techniques, and the lab implemented all the amazing alignment techniques and the AI still did a coup, and the ‘low dignity’ failures are like: we didn't try very hard to develop alignment techniques, and the people who were in the labs completely YOLO-d everything and then we got killed.
And so these are both, in my mind, plausible stories for how the AI takeover happens, so it seems very plausible to me that I'm making a terrible mistake, by assuming that the labs are going to do reasonable things, and trying to make it so that if they try to do reasonable things, it goes well for them.
I'm assuming the alignment plan is similar to what I described: I feel pretty good about this. This is another controversial take. I think that the basic idea of just ‘try to understand whether your AI is doing bad stuff, try to do a really good job of evaluating it, especially in cases where you're going to be very sad if it's actually doing a bad job, and also give it lots of inputs, such that if it was lying in wait hoping to kill you later you would notice, and train against those’... I think that's just a pretty reasonable plan, and I don't feel very bad at all about assuming that.
And then like, should I be trying to contribute to this situation by doing technical research? I don't know, maybe.
So the framework I'm going to talk about here is not that different, it doesn't lead to very different things from other people. Re bullet point 2, I just personally like thinking about this stuff. It's really not obvious whether in fact my research is better, because I try to ask myself the question like, “is this technique that we're trying to research actually better than stuff which is just going to be in the plan by default?”
I think if you look at the research that I did last year, it is not substantially better than what it would've been if I spent no time thinking about ... Maybe it's like 2x better. I don't think it's wildly, wildly better, than if I had spent almost no time asking myself what the situation with AGI was going to be like, and just stopped after like one sentence of reasoning.
If I had said to myself "Surely it would be nice to know what was happening inside the neural nets", and then I just tried to do good science on top of that, I think that probably would've done about as well in terms of my actual research output, so I'm definitely making a controversial bet by saying "You should try to think carefully about what's going to happen with alignment, when you're prioritizing your research." I'm going to keep making this bet for the moment, but it does seem like plausibly a mistake.
Classes of technical research
Okay. So I want to talk about some different classes of technical research, broken down by how you assess a completed research project. And each of these is a different answer to the following question: Why do you believe that this research project is in the direction of producing helpful changes to the plan?
We're going to have five classes of technical research, okay?
Ready to go
Number (1), ‘Ready to go’: why do you believe that this research project is in the direction of producing helpful deltas to the plan? Well, the technique we developed here has now been demonstrated to work, and it's just obviously applicable. So now that I've written this paper, if we had to align an AGI right now, we would just definitely try using this technique that I just developed. So obviously it's relevant.
This is the ‘ready to go’ category. I'm like: the reason that you research [this] is that you just think it's ready to go to be used to align some AGIs. Examples of things along these lines: the critiques research at OpenAI where they train AIs to point out problems with the outputs of other AIs – that just seems like an obvious kind of thing you wanna be able to do for alignment. That's category one.
Worst-case techniques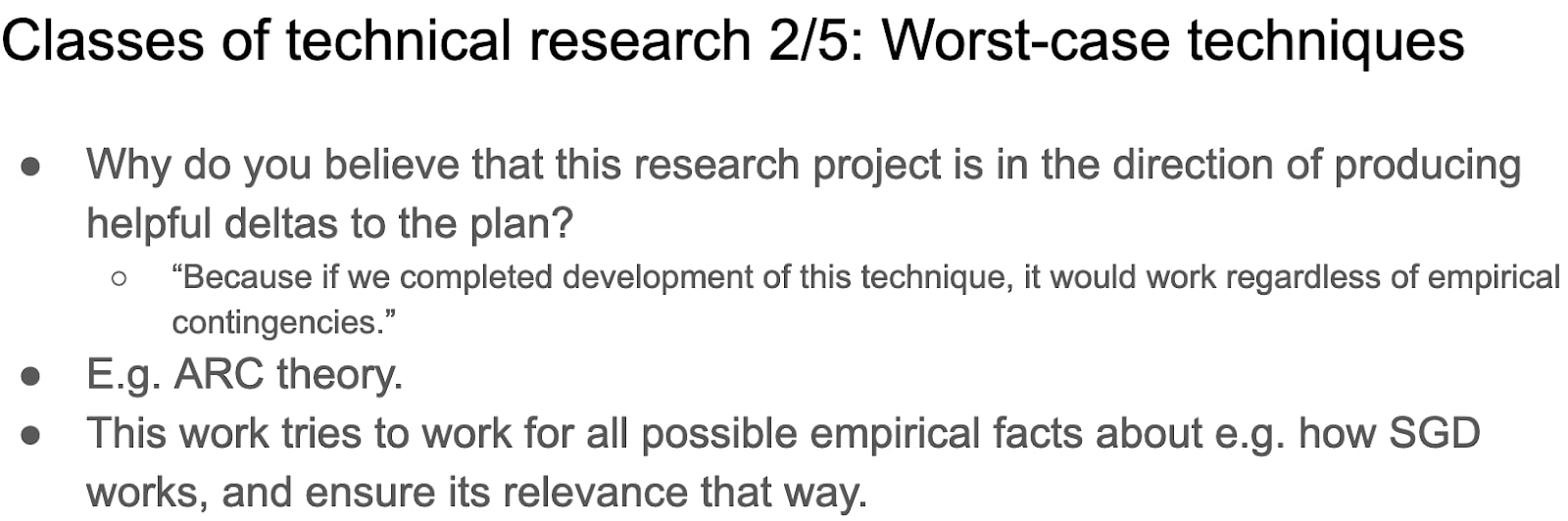
Category (2), ‘worst-case techniques’. Why do you believe this is in the direction of producing helpful deltas to the plan? Because if we completed development of this technique, it would work regardless of empirical contingencies. So this is a very different argument for why you think it's going to be relevant: the argument is because we just literally didn't rely on any factual predictions about the world that might or might not be true. We just relied on “we're using machine learning” or something.
In my opinion, the majority of value that is produced in the worst-case techniques department is from ARC theory. Yep. That's my Clause (2).
Gain of function
Clause (3), gain of function research. So gain of function research is: you try to make empirical demonstrations of things that are as close as possible to the reason why we're scared about AI takeover eventually. Like you try to develop situations in which your AIs do scary misaligned stuff. Currently the people who are doing stuff most closely related to this are the ARC Evals people, though Ethan Perez also does some stuff on this.
The reason why this will be super helpful is, if we just had empirical demonstrations of our models doing things that feel very closely analogous to the reason we're worried they might stage a coup later… I mean it just seems really obviously helpful for a variety of reasons. We'd answer a bunch of questions about what types of failure modes seem most likely. The one that really brings joy to my heart is: we'd be able to do empirical research where we get to evaluate our research based on whether it fixed something which was a close demonstration of an actual alignment problem in an actual model, as opposed to what we do right now, which is having to give worse analogies.
So I'm like, it would be really great, if we had empirical demonstrations of all these failure modes. Unlike the other classes, this gain of function research stuff does not cash out in a technique. I sometimes use this metaphor of training wheels, like we wanna know if it's physically possible to ride a bike, and currently we've just got this dude laying on the ground, and you've got a bike, and you put the man on the bike, and he just topples over, and you're like, "Well, the first thing I'm going to do is I'm going to stick on some training wheels that are very close to the ground", and then you put the dude on the bike, and tell him to pedal, and he pedals forward a little bit or whatever with the training wheels, and you're like, "Well, we haven't established that it's physically possible for this dude to ride a bike, but we have done one of the intermediate steps: if he couldn't do this, he definitely can't ride a bike."
And so I think of this as, you have a sequence of training wheel distances down: there's a sequence of different things that all have to happen for your models to successfully stage an AI coup. They have to want to, they have to be able to, they have to be able to hold it together long enough to not just tell you that they're in the middle of staging a coup. And I'm just like, currently we haven't seen many demonstrations of many of these things, though increasingly many in the last few months – thanks Sydney.
And I'm just really excited for, at every point in the future, having demonstrated the failures with the tallest training wheels that we can make happen at that moment. That great, that's gain of function research.
Low-hanging fruit
Number (4): low-hanging fruit. Why do you believe that this research project is in the direction of producing helpful deltas to the plan? Well, I don't have a really great story, but it seems kind of relevant, and is cheap and easy. From my perspective, this is what the ML academics just do all the time. They're just like, what's some research that's kind of easy, and has keywords in common, with the problem that I eventually want to solve?
I'm like, no shame in that: I regret not being more persuaded by the benefits of doing stuff that's easy, over the last few years. But the key difference between this and the other ones is like, you just really emphasize doing stuff that seems easy. So an example of this is in Collin Burns' "Discovering Latent Knowledge" paper, he has this thing where he is looking for a direction in activation space, and from my perspective, that technique… like there's no particular principled reason to think that this would work when other techniques wouldn't, but who knows, it's some stuff which is kind of different to other stuff. Seems good to try out. It only took like one grad student a few months or something, so it seems like it's worth the money.
Big if true
Okay, classes of technical research, 5 out of 5: big if true. In this case, the answer is: it sort of seems like it ought to be possible to do this, and it would be super great if it worked. So this is how I think about almost all of the research on the internals and models, which is, for example, interpretability and some other things.
Basically we're just like: interpretability, it doesn't work in the worst case, it doesn't help us right now, like we couldn't use it for anything if we had to align an AGI tomorrow – maybe a slightly controversial hot take. It's not helping us develop better threat models and it's not easy, it's hard, and expensive, but if it worked, man, that would be sweet.
Just intuitively, it would be so good if you could edit and read the internal states of your models, and perhaps we're so excited by that sweet, sweet possibility, that it's worth doing this research, even though it has all those other downsides. So that's basically how I think about model internals research, like interpretability.
So by ‘model internals research’, I mean anything where you take advantage of the fact that your model is just on your computer, and you can read any of its internal states, you can do interventions, you can just edit or whatever you want with the internal states of the model while it's running.
Currently there isn't very much useful that we do in practice, using this capacity that you would think in theory would be super helpful, but maybe one day there will be something useful. I often think of this as ‘model neuroscience’, it's like if you wanna understand a human brain, one thing you can do is cut up the skull, and peek at it or something…
You might think that neuroscience would be really helpful to prevent lying. One thing you might notice about this is that this does not seem to be true of people who wanna prevent coups in practice right now. My understanding is that people who currently wanna prevent coups in fact get very little value out of neuroscience, they don't even have truth serum.
This is an example where some people wanna prevent a coup, and you might be like, "Well, why would this situation be different with AIs, than it is with humans?" And it's like, well, human neuroscience is way more of a pain than AI neuroscience, because the things are just literally already on your computers, and you’d really think you would be able to do something with it.
So unfortunately, the thing which I crave is an example of taking this ‘big if true’ kind of research, and converting it into something which is ready to go. Like converting it into a technique, such that if we wanted to build AGI next year, we would be able to use it, and it would contribute. I would be thrilled if that happened. Unfortunately it has never happened, but maybe this year, you never know.
What the current portfolio should be
If I got to pick the current portfolio of alignment research, here's what I'd pick. We've split it up by gain of function versus anything else, because the gain of function isn't directly leading to new alignment technical research. Those are my numbers.
How will this change between here and AGI?
How do we think this will change between here and AGI? My best guess as to how this changes between here and AGI is basically: eventually the gain of function people will succeed, and then we'll have all these great empirical alignment failures, at which point the low-hanging fruit stuff is going to look way better. Currently when the ML academics write these random papers about random unprincipled techniques, I look at it and I'm like, "Well, okay, I can kind of see why you think that would be helpful", but actually I have no evidence that it is. The demonstration that this is relevant is super sketch. But if we had actual demonstrations of alignment failures, I think that just making a big bag of tricks, where you just try unprincipled things, and see which ones work empirically would look a lot more promising to me.
So I'm very excited for the gain of function stuff, mostly because it enables the low-hanging fruit stuff. Also probably we're going to run out of time to do worst case-y stuff, and big if true stuff. So that's going to be exciting.
Let me think about how I wanna conclude this. The way that I personally engage with prioritizing alignment research in both my own research, and when thinking about how I would advise other people to do their research, is by thinking about what I think would happen, if we had to align an AGI right now, and then thinking about what we could do now that would produce a helpful delta to that plan. And I've laid out a number of different possibilities for what that might look like. And again, I wanna say, I think thinking through all this stuff for me has not clearly panned out. Frankly I'm very proud of my alignment takes, but the ‘take to value production pipeline’ has not yet been proven, and I think it's like very plausible that in a year I'm going to be like: damn, I was just wasting time thinking in advance about how we're going to align this AGI. We should just have been way more empirical, we should just been like, let's just do some vaguely reasonable looking stuff, and then got into it later. That's my talk.