I recently tried to figure out where DALYs come from.
After a bit of searching, the best I could find was this report on the origin of the metric (the first Global Burden of Disease assessment). The report includes this explanation:
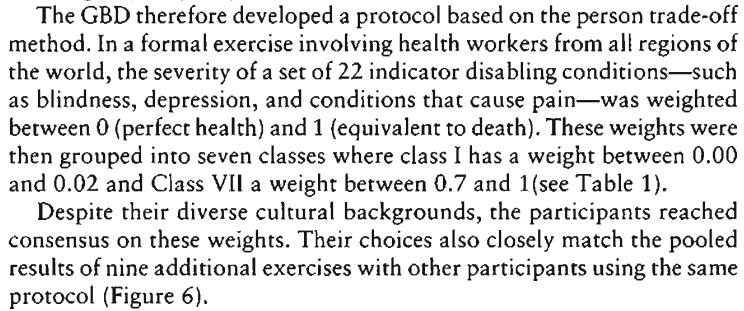
And:
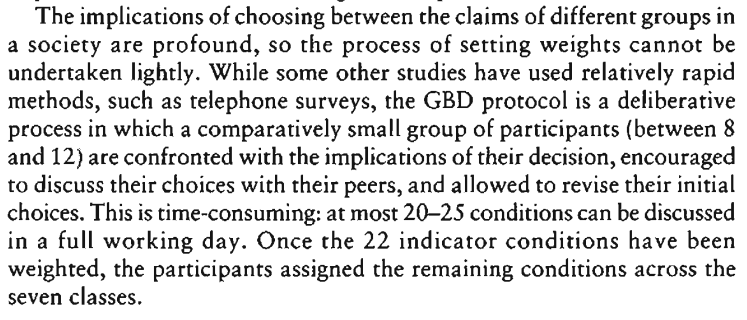
But I'm left with many other questions:
- How many health workers were consulted?
- Were people other than health workers consulted, especially people who have themselves experienced the relevant health issues?
- Were DALY values updated in successive instances of the GBD?
- Are transcripts of any of these "formal exercises" available somewhere?
Ideally, I'd love to find a document/video that covers DALYs in the style of a factory tour video; I want to know what goes into them, who is involved, and what the creation process looks like.
Does anyone know of such a resource, and/or the answers to any of my questions?
This is excellent, thanks!
These two papers, in particular, were what I was looking for. The corresponding information on QALYs was also great.
(For future readers of my post, the relevant info is under the "descriptive system" and "valuation methods" subheadings in Derek's post.)
I just had the exact same question, so thanks Aaron for asking this, and Derek for giving this answer :)