This post is intended to be an entry to the GiveWell Change Our Mind Contest.
0.Summary
Givewell’s cost-effectiveness model for Seasonal Malaria Chemoprevention (SMC) is clearly laid out and generally very well evidenced. I have identified two areas where I believe an improved methodology could significantly change the results.
Firstly, the cost estimation used by Givewell underestimates the economies of scale in delivering SMC, and so underestimates the cost-effectiveness of the intervention in future.
Secondly, Givewell utilizes disease burden estimates for malaria from the Global Burden of Disease. An alternative WHO malaria data source however finds significantly different, and generally lower, estimates of malaria burden in three of the five country estimates used by Givewell. Givewell should investigate these differences more closely and potentially conduct primary data collection to seek to validate their use of country estimates. Alternatively, cost-effectiveness using the lower WHO estimates could also be presented to show a range of cost-effectiveness.
- Cost estimation
To estimate costs for a given country, Givewell takes the approach of developing a cost per SMC cycle over the 6 years of available data (2015 – 2020) inclusive. Whilst defensible, I feel that this approach does not fully utilize the available information and neglects the importance of economies of scale.
I believe the analysis should aim to project forwards what the cost-effectiveness of future money directed to SMC would be, rather than simply averaging the cost of previous programs.
Taking the year 2020 as an example, we can see that the cost of commodities, freight, and stock management came to 41% of the overall cost (pre-management fee). Other cost buckets in the analysis (monitoring & evaluation, equipment, research staff, communications, planning) are unlikely to scale linearly as the program increases.
I would argue therefore that the majority of the program cost is unlikely to scale 1:1 with an expanded program. There will be efficiencies of scale which can be realized.
We can see this when we plot a cross-country comparison of scale of the project (represented by total person months of coverage) and cost per SMC cycle as shown below. Each dot represents the cost of an SMC cycle for a single year across all countries. As we can see, as the scale of the project increases, the cost per SMC cycle delivered falls.
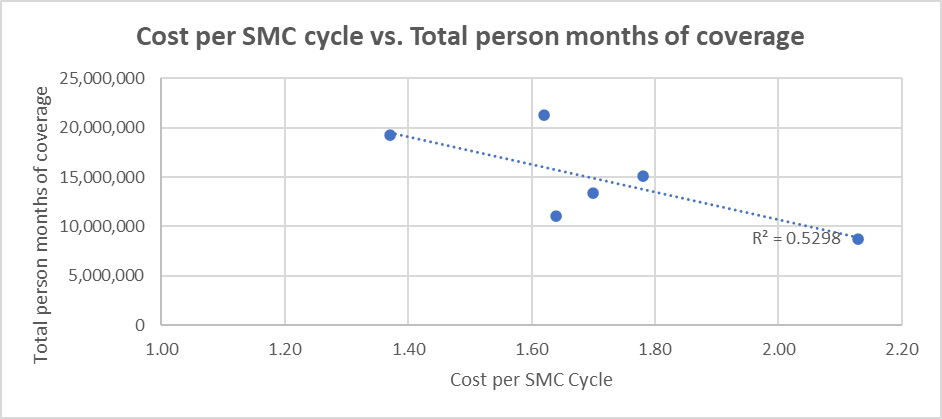
I believe it is an important message, which is missed at present – that increased donations to the SMC project are likely to have increasing returns, not constant returns.
The current approach also means that if the cost-effectiveness of the program changes in future, any change will be under-weighted – because the average cost over the period will only change slightly, due to the weight of previous years.
This second graph shows largely the same point – that as the total program cost has increased for SMC, the cost per SMC cycle has fallen. If we accept the trendline shown below, spending over $35m could lead to an estimated cost per SMC cycle of ~$1.5, rather than the $1.66 used in Givewell’s cost-effectiveness analysis.
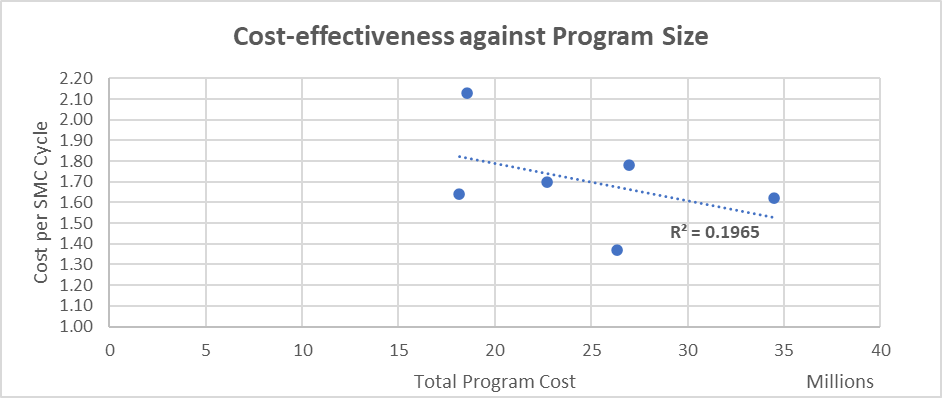
There are several ways to implement a change to address this issue.
My proposed approach would be for Givewell to work with Malaria Consortium to understand which costs are relatively fixed for future years (e.g. staffing levels) and which are variable (e.g. purchase of commodities). This could then be used to create a theoretical cost per SMC cycle based on the estimated amount of donation that Givewell anticipates giving to Malaria Consortium.
2. Estimation under-5 malaria deaths
The figures used in the cost-effectiveness model come from the IHME Global Burden of Disease. The IHME breaks out these figures by age-related groups and by country which is helpful for the analysis Givewell has conducted.
However, it is notable that the IHME estimates of malaria impact tend to be higher than the World Health Organization’s estimates[1].
If we assume that the age distribution of deaths in the IHME and WHO estimates are equal, then adopting the WHO estimates would substantially change Givewell’s estimates for cost-effectiveness in individual countries.
Across the five countries analysed in the Givewell model – the IHME estimates of malaria deaths are higher in four countries, but substantially lower in Chad. The overall proportional differences between the two models across the five countries is relatively small, this is largely driven by the fact that the estimates are very similar for Nigeria, which is a very large country.
The WHO malaria report estimates the number of child deaths from malaria according to a verbal autopsy multicause model which estimates causes of death for children aged 1–59 months. The resulting cause-specific estimates were adjusted, country by country, to fit the estimated 1–59 month mortality envelopes (excluding HIV and measles deaths) for corresponding years. Estimated malaria parasite prevalence was used as a covariate within the model.[2] The WHO also estimates a range of malaria deaths, and in three of the five countries the IHME estimates are outside of the WHO range.
Recommendation: Givewell should investigate the reasons behind the WHO & IHME divergence, with particular focus on Chad, Burkina Faso and Togo where the divergence is large and outside the WHO’s reported confidence intervals. Givewell might wish to consider using WHO data as an alternative for any sensitivity analysis they plan.
Country | Malaria Deaths: IHME Estimate (2019) | Malaria Deaths: WHO Estimate (2019) | Difference (As proportion of IHME estimate) | IHME result outside of WHO range? |
Burkina Faso | 26,305 | 18,813 | -28.5% | Yes |
Chad | 7,194 | 11,619 | +61.5% | Yes |
Mozambique | 20,528 | 19,189 | -6.5% | No |
Nigeria | 191,106 | 187,437 | -1.9% | No |
Togo | 5,436 | 3,584 | -34.1% | Yes |
|
|
|
| |
Total | 250,569 | 240,642 | -4.0% |
|
Sources : WHO Confidence intervals: https://apps.who.int/gho/data/view.main.MALARIAESTDEATHSvc?lang=en All other data in table: https://ourworldindata.org/malaria#data-quality-definitions |
[1] https://ourworldindata.org/malaria#data-quality-definitions
[2] https://www.who.int/data/gho/indicator-metadata-registry/imr-details/4650
Thank you for your entry!
Do you have a take on whether your recommendations here would change GW's funding allocation, and how (up or down), as per the contest details? If I understand you correctly, the efficiencies of scale section implies slightly increasing funding while switching to WHO data over IHME implies slightly reducing it; I'm unclear as to what the direction of the change is on net.
Thanks for the comment. I don't think that these recommendations would significantly change GW's funding allocation to be honest, they just lead to more accurate estimates of what will still be a very cost-effective program.
My caveat is, I think there is a larger potential decrease in cost-effectiveness from the second point on burden of malaria. It's unsatisfactory I think that the estimates in several of these smaller countries are significantly different. It's possible that the cost-effectiveness of SMC in some of these geographies could be quite a bit lower than Givewell estimates, simply because there is less malaria in those countries than previously thought.
One of the problems I would have liked to have gotten into - is that other febrile diseases are often misdiagnosed as malaria (or no diagnosis is performed other than just assuming fever = malaria).
My hypothesis going into this analysis was that I might be able to investigate this further, and perhaps show that some of the disease burden is not malaria. But I haven't really turned up many good resources on this.
Given that Givewell have a lot more resources than just me & malaria is a big part of their programming, I think best way forwards is for them to look into the issue and try to get to the bottom of how much malaria is really out there.