Introduction
When I first became interested in effective altruism, I would describe it to people I met as “checking whether a given charitable intervention was helping.” This turned out to be less accurate or unique than I thought at the time, but is still something I value quite strongly. So when QURI approached me with the idea of investigating “evaluation science”, I was quite excited.
“How to do evaluations” is a far bigger topic than can be covered in one post, so I selected an easier target. The post will address the question “on what occasions, or in what circumstances did evaluations help at all?”. The goal was to counterbalance the abundant stories of evaluations backfiring and to look for patterns in the successes.
But, as I wrote, I realized that even the examples I was fairly sure of were hard to quantify in their impact. Did they improve things by 10%? 100%? 1000%? I started looking for quantifications. These were surprisingly hard to find, and sometimes what I did find cast doubt on the examples. So, I started digging in more rigorously.
You can guess where this is going. The stories I found were frequently exaggerated, controversial, self-validating, or were just going to take a lot more time to quantify, than what I had available. As a result, this post became a list of case studies on trying to determine the value of evaluations.
The case studies are heavily weighted towards topics I already had some familiarity with. Topics where, even if I didn’t necessarily know everything about each example, I knew what to look up. Reaching similar thoroughness in new-to-me examples would have been quite valuable, but was not possible with the time allotted. I decided that a biased selection of somewhat more thorough examples was preferable to a less-but-still-somewhat biased selection of more shallow examples. Additionally, I only included external attempts to evaluate evaluations (rather than make my own Fermi estimates). However, I have no doubt I am about to invoke a tsunami of Cunningham’s Law, and welcome both brand new case studies and additions to my own.
Case Studies
Physics
Things like mass, distance, and time should be among the easiest traits to measure, and they are, now. However, finding universal definitions for each of these concepts has been a challenge. For example, the definition of a kilogram has gone from being described by the weight of a liter of water, to a reference object, to being defined by fundamental constants. There’s an entire book on Inventing Temperature.
How useful are these measurements? That’s probably the wrong question. Even certain ants can measure distances, we’re not going to suddenly wake up and forget how to figure out which of two things is longer. But I was hoping to find information on the marginal value of marginal precision. I’m sure this exists, because people would not buy a $1000 level if a $12 one would do the job. But I could not easily find it.
Moneyball/Sabermetrics
As described in the book Moneyball, Sabermetrics was a program by the Oakland Athletics to quantify baseball players’ skills and systematically hire those who were undervalued by the market, creating a team that punched above its financial weight in wins. This is frequently touted as a strong victory for statistical methods, marred only by the fact that it’s anti-inductive; once a trait becomes known as undervalued, it will rapidly stop being so.
When I looked into this, the question of how much and even whether Sabermetrics translated to more wins was surprisingly controversial. Some of the defenses (“They didn’t win more, but they got better players than they would have otherwise”) are essentially self-validating (others are not). I would need to spend several hours learning the basics of baseball before feeling confident enough to draw a conclusion.
Armed Forces Qualification Test
The military uses a battery of tests, including The Armed Forces Qualification Test (AFQT), in order to determine who to accept and where to place them. From the military’s perspective, success means placing applicants in jobs they can excel at, and rejecting people who are worse than the counterfactual.
How well does it work? When standards have been lowered—either due to massive troop needs, or a testing error—the newly allowed troops performed much worse than those who scored better on the test. Of the top scorers, 95% finished basic training. Of the lowest, 20% do so. When standards were lowered during the Vietnam war, sub-standard troops were 11 times more likely to be reassigned—a signal they failed at their placement—and 10 times more likely to require remedial training.
This is all nicely quantified, good job military.
Apgar score
This is a very crude test in which five aspects of a newborn’s health (e.g. “are they breathing?”) are visually assessed by a doctor and combined into a single score from 0 to 9. In addition to flagging when a newborn needs help, it reportedly helped create a culture where struggling-but-viable infants received intervention, instead of being dismissed as stillborn.
I was unable to find specific numbers for how the creation and uptake of Apgar scores affected infant mortality. From eyeballing infant mortality statistics, it’s obvious the decline started long before the score was invented, and there’s no obvious discontinuity after the Apgar score was invented in 1952.
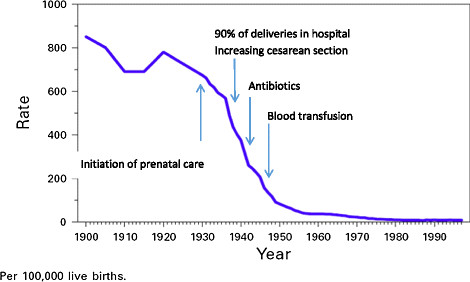
PageRank
PageRank was Google’s initial algorithm for determining the relevance of web pages to search queries. It was universally hailed as an enormous improvement over the top search engines of the time, and launched a company now worth over one trillion dollars. This gives some idea of the value Google captured from PageRank. But, how much value did it generate? What did a better search engine mean to users? In 10 minutes of searching, I was unable to find any estimates.
This would not be so big a deal—everyone agrees early Google Search was head and shoulders above contemporaneous search engines. However, everyone also has a vague sense that internet search has slowly degraded over time, and I would like a way to quantify that.
PageRank became less valuable for Google Search as it became better known. This is because the people most incentivized to maximize their page rank were not the ones with the best content. However, the algorithm is still considered fairly useful in harder-to-game areas like scientific publishing.
SATs
Apocraphylly, the SATs were originally intended to make universities (or perhaps just the Ivy League) accessible to students who didn’t go to one of a handful of feeder schools, by making their talent legible. Did they succeed in this goal? The number of non-prep-school attendees at the Ivy League schools has definitely gone up since 1926, but I couldn’t find anything to speak to the causality of this change. It’s worth noting the tests are now frequently accused of doing exactly the opposite, serving as an obstacle to underprivileged students attending top universities, to the point that many colleges are dropping them.
The purpose of modern SATs is described as “to measure a high school student's readiness for college, and provide colleges with one common data point that can be used to compare all applicants”. How are they doing at that?
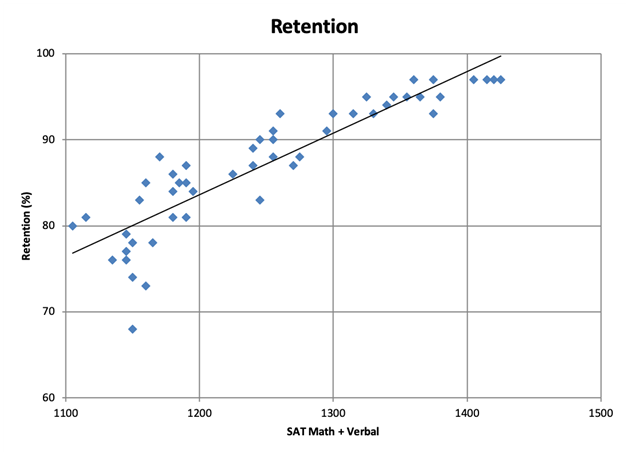
SATs vs 1-> 2 Year Retention at State Flagship Universities
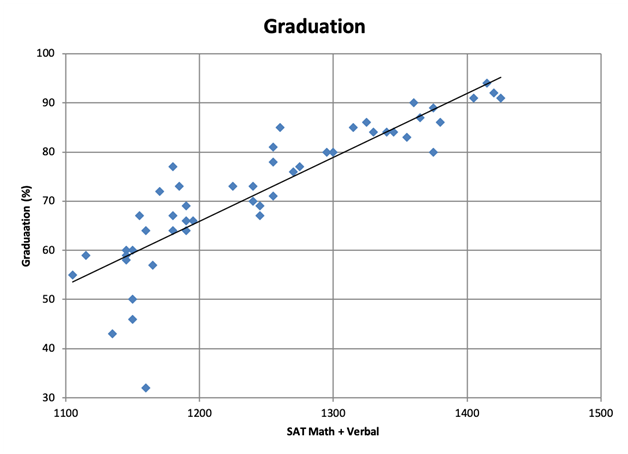
SATs vs 6 Year Graduation Rate at State Flagship Universities
The charts might suggest a strong correlation. But! The SAT scores are also correlated with a number of other factors that may also affect college success, for instance, high school GPA and parental SES. Having said that, this site controlled for GPA and found the predictive power still held.
There is a lot of literature about SATs. Even without diving into all of it, if we assume the SATs do predict some combination of first year college grades and six year graduation rates—how much does that matter? The answer partially depends on how much trust we place in college grades themselves, but graduation is quite a useful metric. College costs are up-front and independent of graduation, but the economic rewards are typically only bestowed upon people who get a diploma. This suggests that a student who does poorly on the SATs should reconsider college, even if college graduation itself is only important for signalling. This makes SAT scores useful to the students even if they measure nothing we care about.
Conclusion
Evaluation is a hard task, and that includes evaluating the success of evaluations themselves. This would not be a surprising conclusion, except that I started from a sample set that should have been heavily biased towards success. Even when using commonly known success stories, quantifying success in evaluation was quite difficult. Based on this experience, I have greatly upped my estimate of how difficult it is to create really useful assessments—and it wasn’t small to start with.
Nothing I learned made me think good evaluations were less valuable—when they do succeed, they are quite powerful. This makes me hopeful about the value of continued research in the area.
Possible next steps include: a deep dive on specific case studies to either find hidden estimates of usefulness or create novel ones, or a broader survey of evaluations to look for patterns across a much larger collection of data. I started the latter as a part of the process that led to this post. The (barely populated) spreadsheet is available here. I suspect getting truly useful results with either of these approaches would be measured in dozens of hours.
This work was paid for by QURI, as part of its larger investigation into evaluations. Thanks to Ozzie Gooen for comments and Marta Krzeminska for copyediting.
Offline, someone suggested the Marine Chronometer as a physics measurement device that straightforwardly created a lot of value by enabling long distance navigation at sea.
In the summary you wrote
Do you mean useful assessments of evaluations or useful evaluations?
the latter, in part because of the former.