If we knew that human-level AI couldn’t be produced within the next 20 years, we would take different actions to improve the long-term future. Asya Bergal of AI Impacts talks about her investigations into why people say we won’t soon have human-level AI, including survey data, trends in “compute,” and arguments that current machine-learning techniques are insufficient.
We’ve lightly edited Asya’s talk for clarity. You can also watch it on YouTube and read it on effectivealtruism.org.
The Talk
Aaron Pang (Moderator): Hello, and welcome to “Reasons you might think human-level AI is unlikely to happen soon” with Asya Bergal. Following a 20-minute talk by Asya, we will move to a live Q and A session where she will respond to your questions.
[...]
Now I would like to introduce our speaker for the session. Asya Bergal is a researcher at AI Impacts, where she also heads up their operations. She has a BA in computer science from MIT. Since graduating, she has worked as a trader and software engineer for Alameda Research, and as a research analyst at Open Philanthropy. Here's Asya.
Asya: Hi, my name is Asya Bergal. I work for an organization called AI Impacts, though the views in this presentation are mine and not necessarily my employer’s. I'm going to talk about some reasons why you might think [reaching] human-level AI soon is extremely unlikely.
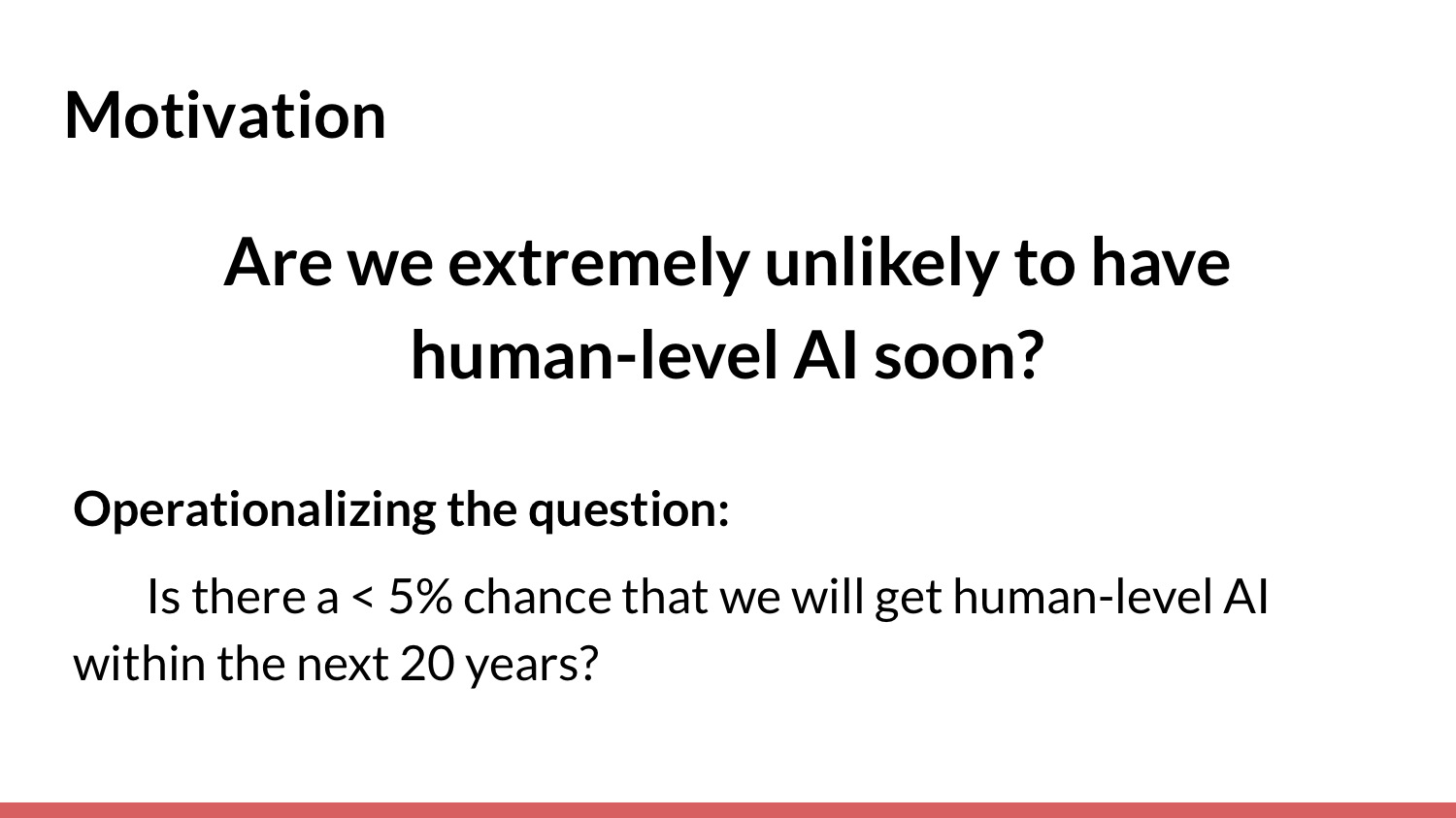
I'll start with [some common] reasons. I'm interested in these because I'm interested in the question of whether we are, in fact, extremely unlikely to have human-level AI in the near term. [More specifically], is there a less-than-5% chance that we’ll see human-level AI in the next 20 years? I'm interested in this because if [reaching] human-level AI soon is extremely unlikely, and we know that now, that has some implications for what we, as effective altruists and people who care about the long-term future, might want to do.
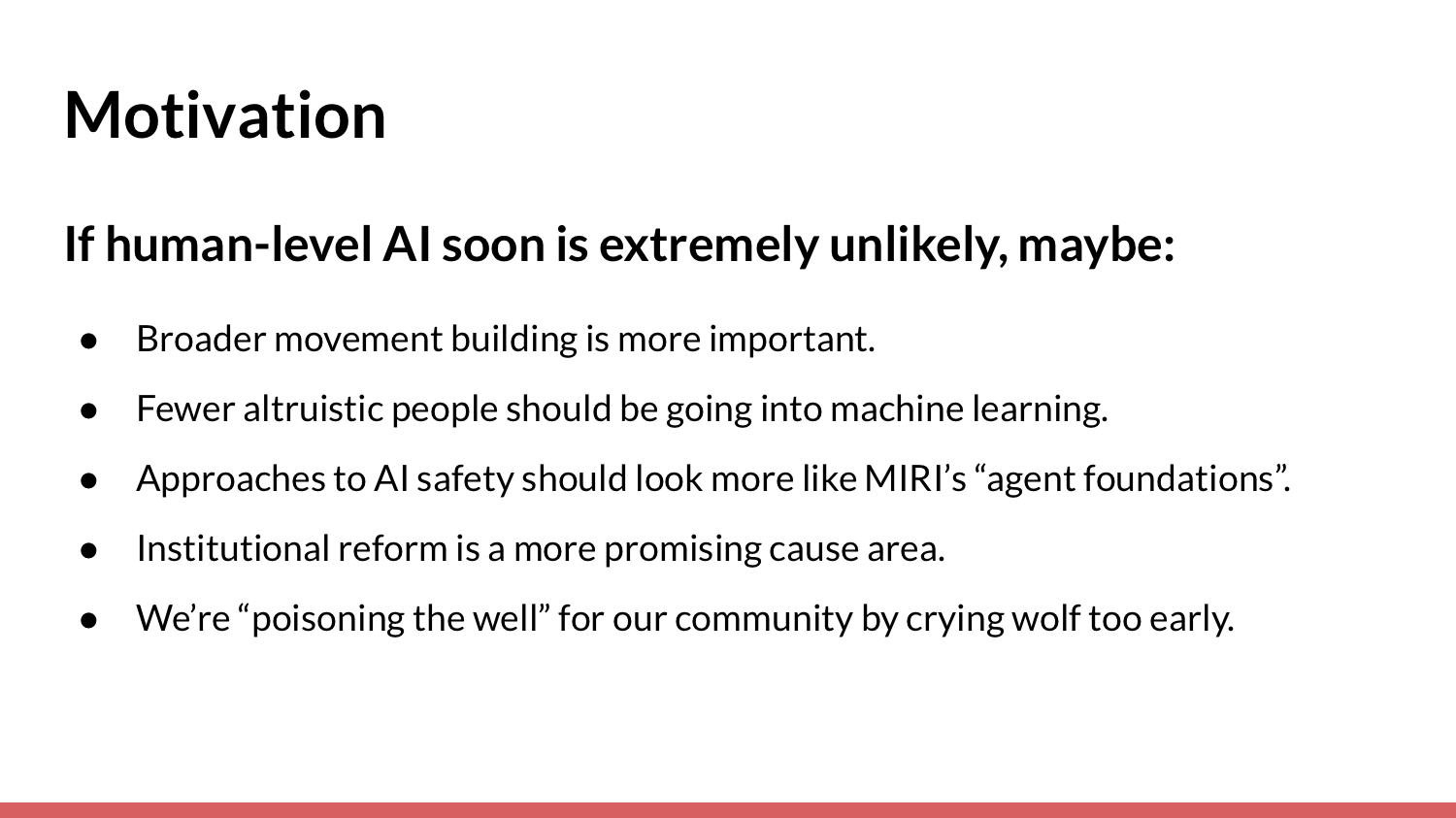
If human-level AI is extremely unlikely, you might think that:
* Broader movement-building might be more important, as opposed to targeting select individuals who can have impact now.
* Fewer altruistic people who are technically oriented should be going into machine learning.
* Approaches to AI safety should look more like foundational approaches.
* We have more time for things like institutional reform.
* There is some effect where, if the community is largely [broadcasting] that human-level AI [will soon occur], and it doesn't, we’ll lose some global trust in terms of having good epistemics and being right about things. Then, people will take us less seriously when AI risk is more of an imminent threat.
I don't know how real this last problem is, but it is something I worry about. Maybe it is a [potential] effect that we should be aware of as a community.
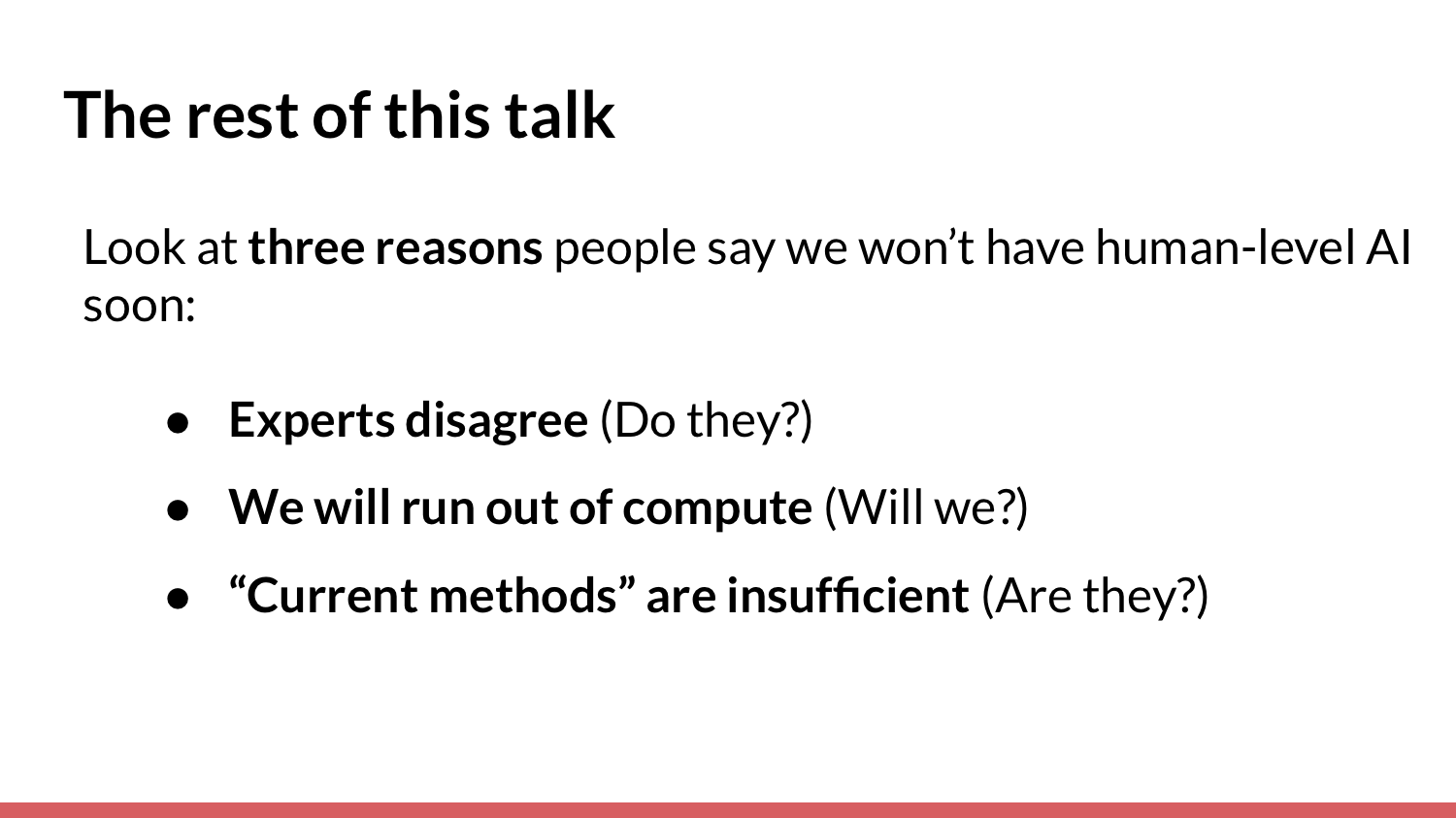
For the rest of this talk, I am going to look at three reasons I’ve heard that people [give to explain why] we won't have human-level AI soon:
1. A privileged class of experts disagree.
2. We're going to run out of “compute” [before we can reach] human-level AI.
3. Fuzzily defined current methods will be insufficient to get us there.
I don't claim that these three reasons are representative, but they're particularly interesting to me, and I spent some time investigating them. I will go ahead and spoil the talk now and say that I'm not going to answer the “5% question” [that I posed earlier], partially because my views on it vary wildly as I collect new evidence on some of these reasons. I do hope that I will, in the near term, get to the point where my views are pretty stable and I have something concrete to say.
Reason No. 1: A privileged class of experts disagree
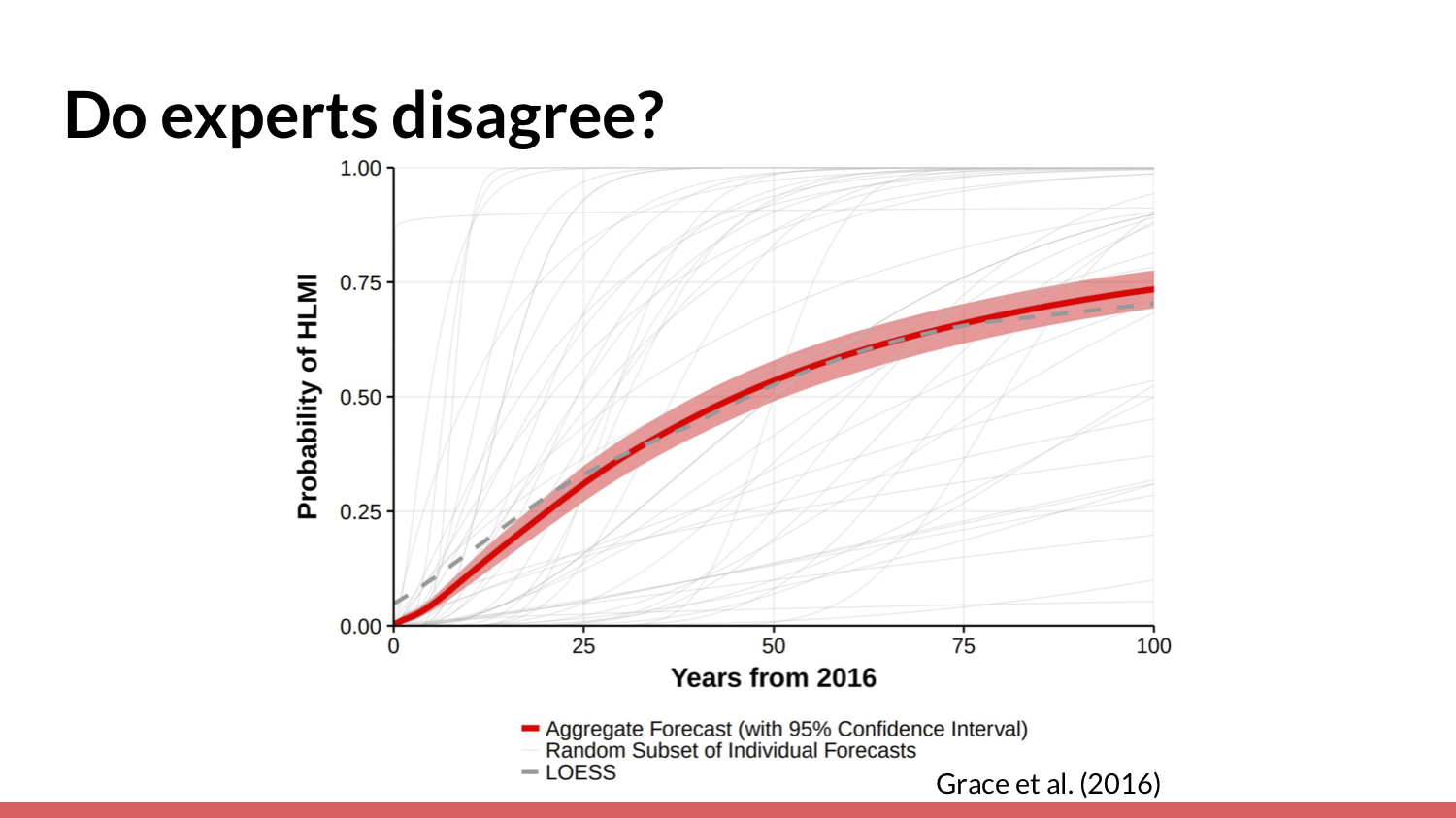
Let’s look into the first reason — that experts might disagree that we can get to human-level AI soon. This is a survey conducted by Katja Grace from AI Impacts and a lot of other people. They asked machine learning researchers and experts what probability of human-level machine intelligence they think there will be [in a given] year.
You can see the 20-year mark [in the slide above]. It really seems like they do think there's more than a 5% chance.
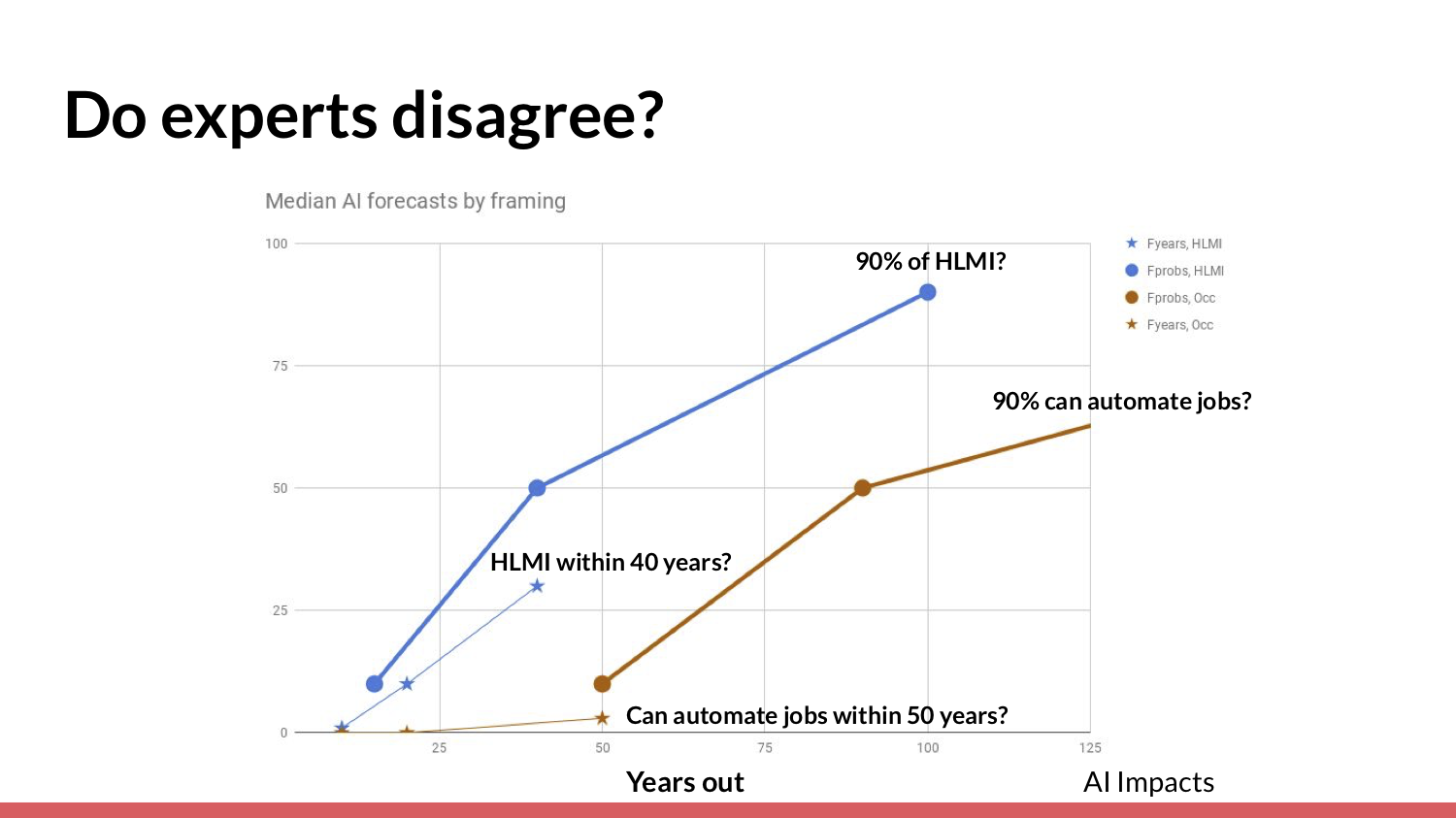
Then, if you delve a little further into the survey results, you see that the answers people give are actually extremely sensitive to the framing and the exact way in which the question is asked — [for example,] whether you ask about human-level machine intelligence, automating all jobs, or the year in which there will be a 90% chance of something happening versus in another year.
For the question “What chance do we have of automating all jobs within 50 years?”, people give pretty low odds. What that tells me — and what others have concluded from this — is that it's very difficult to know what to do with surveys of experts. We probably shouldn't put a lot of weight on them.
I was particularly interested in a kind of survey where you ask people how much fractional progress they thought had been made toward human-level AI. You can naively extrapolate that to figure out how many years it will take until we get to 100%.
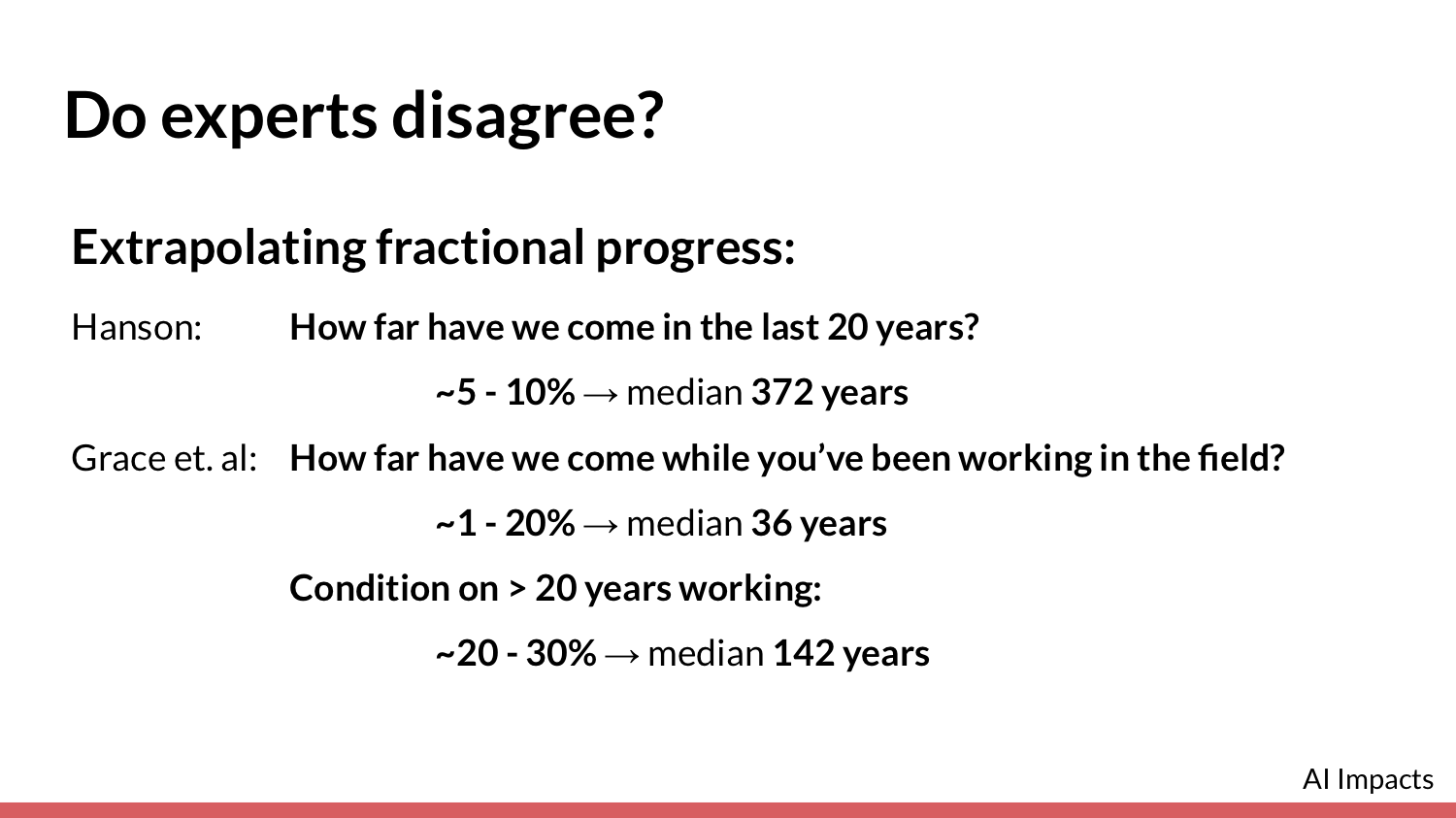
Robin Hanson did a version of this survey. He asked machine learning experts how far they had come in the last 20 years. All of the people he asked had worked in their sub-fields for at least 20 years. They answered in the 5-10% range, which, when naively extrapolated, puts human-level AI at 300 to 400 years away. That is pretty long.
Then, the Katja Grace survey that I mentioned earlier did a very similar thing, but that team surveyed people who had been working in the field for anywhere from two to many years. Their aggregated percentages forecasted human-level AI to be something like 36 years away — much shorter than Hanson's aggregated forecast.
Even if you set the condition of [only looking at responses from] experts working in the field for 20 years or more, they answer in the 20% or 30% range, which [results in] a median forecast of 140 years or so. That’s still a pretty long way off, but not as long as the Hanson survey.
There's a fairly consistent story by which you could reconcile these two results: In the last 20 years, there was a long period in which there wasn't much progress made in AI.
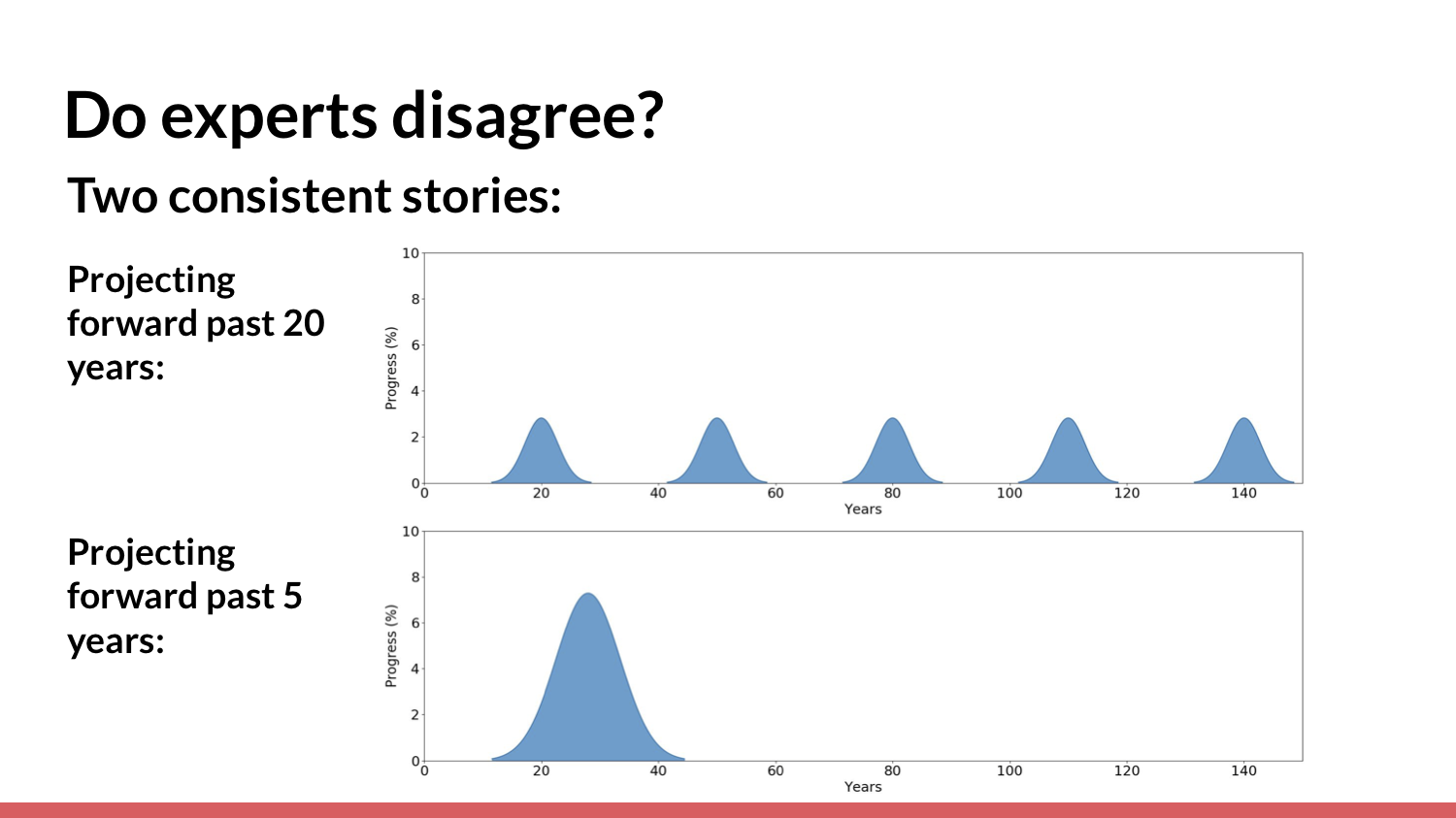
This is a very simplified graph showing that only recently has there been a lot of progress. Across these surveys, people consistently say that progress has been accelerating recently. If you naively extrapolate the past 20 years, you get a boom-bust pattern implying that we won’t have human-level AI for a long time — whereas if you somewhat naively extrapolate the past five years, and perhaps take into account the fact that things might be accelerating, you can get a “pretty soon” forecast.
It's not clear based on these survey results whether 20-year experts definitely think that we won’t have human-level AI soon.
Reason No. 2: We’re going to run out of “compute” before we can reach human-level AI
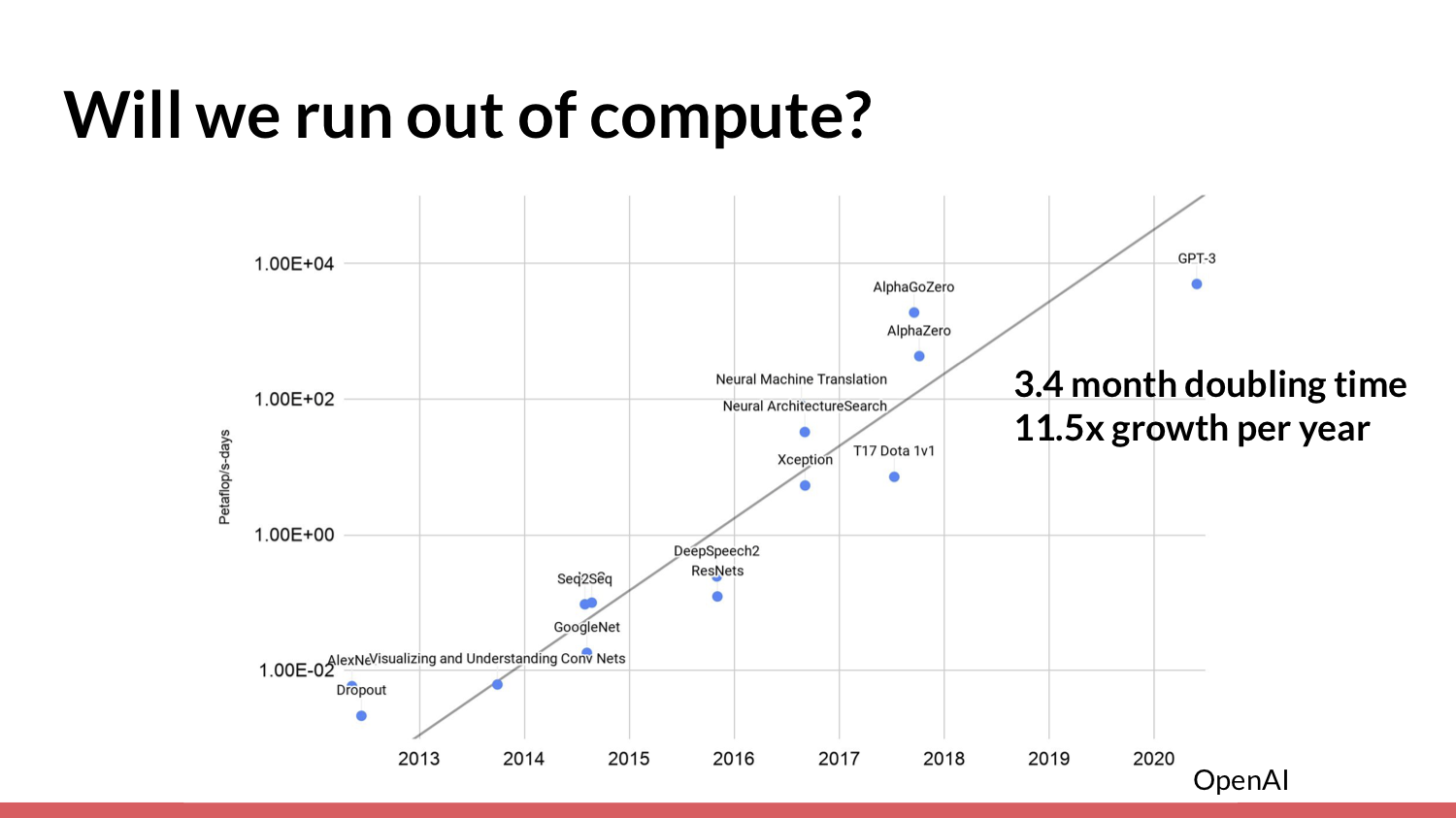
The second reason why you might think we won't soon attain human-level AI is that we're going to run out of “compute” [processing resources]. In an analysis done by OpenAI, researchers looked at the amount of compute used in the largest machine-learning experiments for training from 2012 to 2018. I also [included] GPT-3, because OpenAI recently released [data on] how much compute they used to train it.
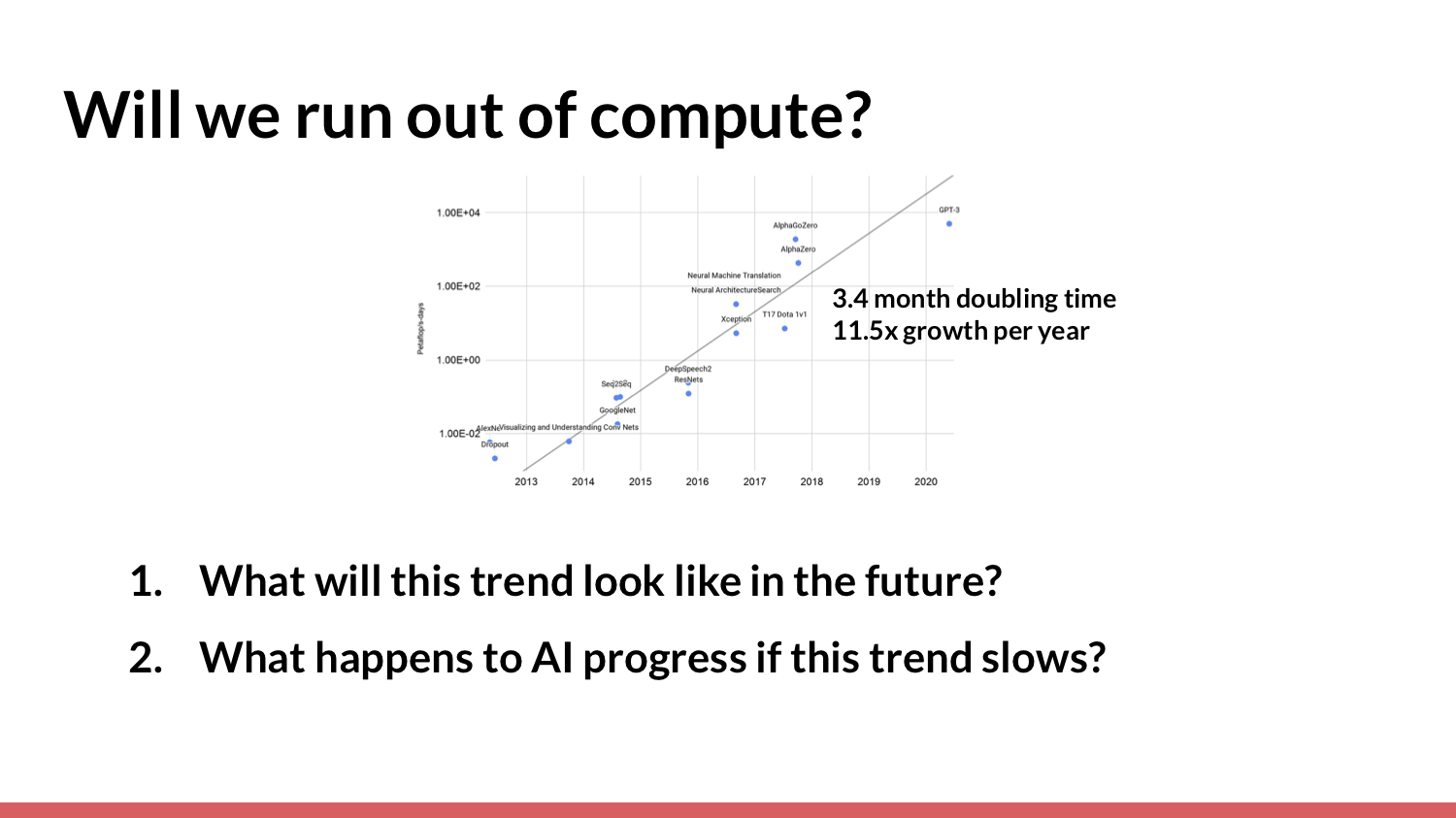
They noticed that over this period of time, there was a pretty consistent exponential rate of growth — around 11.5x per year. A natural question looking at this graph, and given that compute has historically been really important for machine-learning progress (and was important for these results), is: What will this trend look like in the future? Will it be faster? Will it be at the same rate? Will it be slower? What happens to AI progress as a result of this trend?
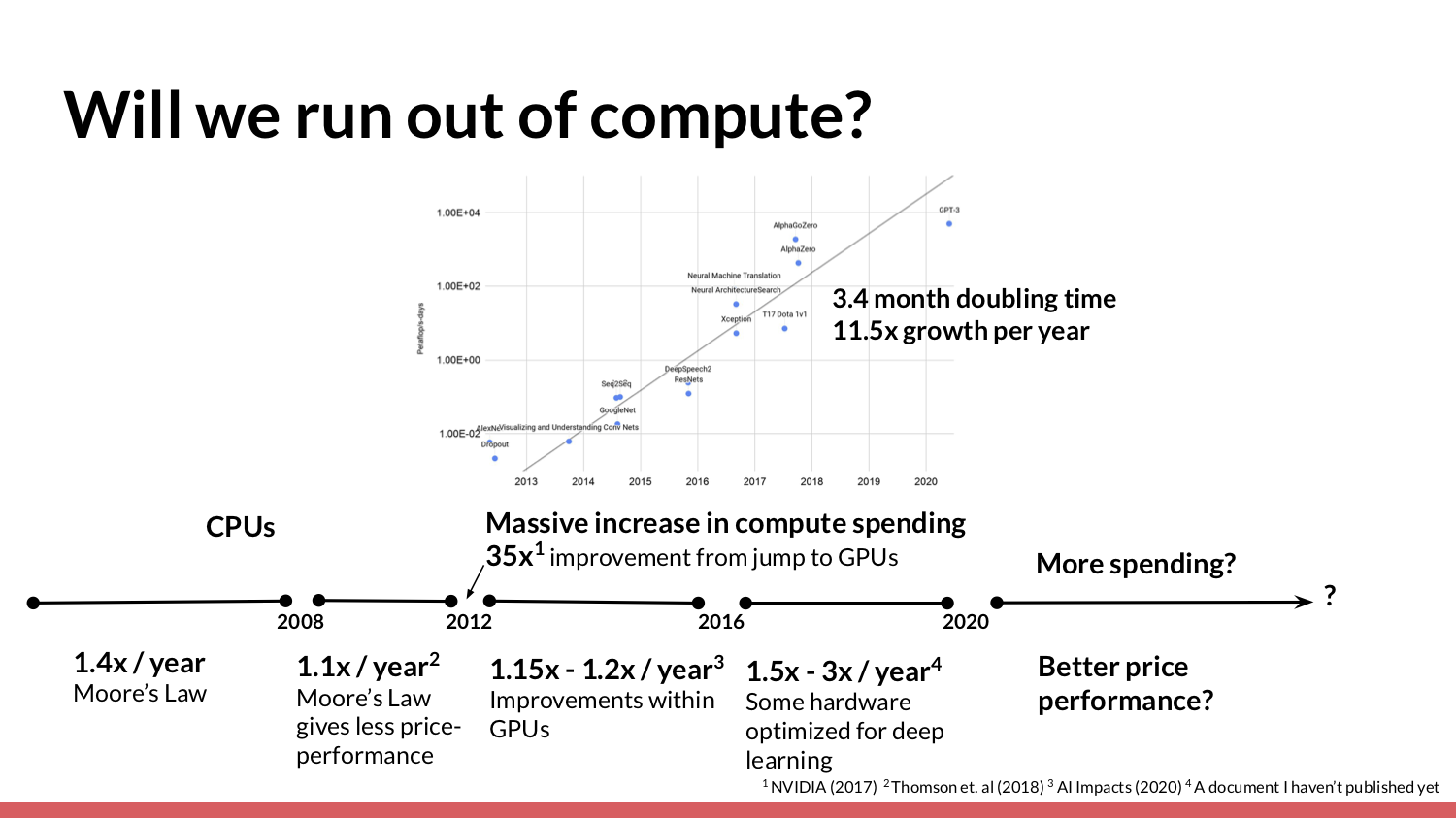
It's somewhat likely that it's going to slow down. Therefore, I'm particularly interested in asking, “What happens if it slows?” For the question “What will this trend look like in the future?”, it's reasonable to start by looking at what has happened with compute in the past. I've tried to illustrate that. I’ll now attempt to explain [the slide].
On the bottom, I put price performance (i.e. improvements in the amount of compute you can buy per dollar). On the top, I put general things that were happening in the world of machine learning training. For a long time, most computation and ML training was done on CPUs, which were governed by Moore's law and showed a 1.4x per year increase in price performance. Then, around 2008, the price performance increase [fell back] and looked more like 1.1x a year. Then, several things started happening around 2012.
One big [change] was that people started training neural networks and machine learning techniques on GPUs, which Nvidia estimated as a 35x improvement for at least one task. Then, starting in 2012 [and continuing today], in 2020, two major things happened. The biggest is that people are willing to spend way more money buying compute, whereas from 2012 to 2016, you may have seen people training on one to eight GPUs. Closer to 2018, you can see that people are using hundreds of GPUs, and the techniques required to enable training on a lot of GPUs at once are also improving. Huge amounts of parallelization [became increasingly feasible].
[Meanwhile], a much smaller effect was that the price performance [the amount of processing power you can buy with a given amount of money] within an individual GPU or an individual piece of hardware improved. From 2012 to 2016, I estimate this to have been about 1.2x a year. Then, around 2016, several different companies started creating hardware that was especially optimized for deep learning. Between 2016 to 2020 I estimate that there was a 1.5x to 3x increase in price performance.
The main factor is that people are increasing their spending. A natural question to ask, then, if you want to know what this trend might look like in the future, is: How much more will people spend? How much more price performance will we see?
[Although spending has] powered growth recently, our spending can’t continue to increase for very long. People estimate that the 2018 experiment cost around $10 million. If we wanted to match the previous trend of 11.5x a year, we could only get to 2022 on spending alone. If we spent $200 billion, which is 1% of US GDP — the amount we [could] see if governments are really interested in machine learning — we would have to compensate largely with price performance after two years.
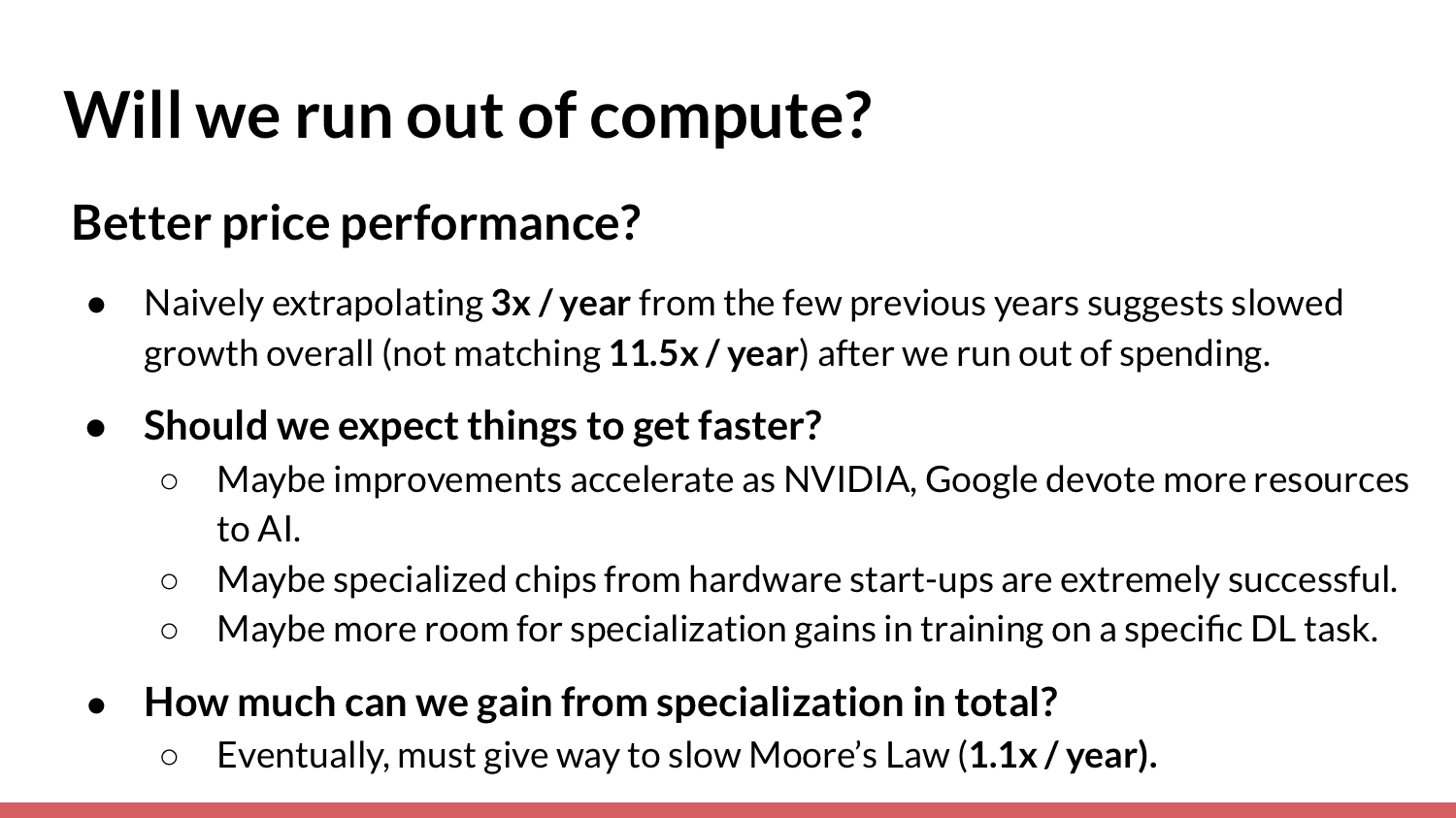
Looking at price performance and naively extrapolating this 3x [increase] from a period when people really started optimizing for this, the result is still that we won’t match the 11.5x that we saw before. [Transcriber’s note: actual yearly increases may be lower if most low-hanging fruit was taken during the initial optimization process, making further increases more difficult.]
I do think there are a lot of reasons that we should think it's plausible for machine learning to go faster in the coming years. Maybe companies like Nvidia and Google invest even more of their resources into AI, and make even more improvements. Maybe some of the startups coming out with specialized chips for AI do very well; maybe if you design a chip specifically for training on a particular type of deep-learning task, then you get much better performance.
Still, we should be aware of the fact that specialization gains have to end at some point, because you're not really making any new fundamental technological advances. You're just rearranging the bits in the computer to be very good at a particular thing. Eventually, we should expect that kind of specialization to end, and for AI to pull back to the trend in Moore's law that we saw before.
I think these two questions are important:
1. How quickly will improvements come?
2. How much [progress will] specialization improvements yield in total?
I think we must answer these in order to estimate what compute progress — and therefore AI progress — might look like in the future. That's the kind of work I'm interested in doing now.
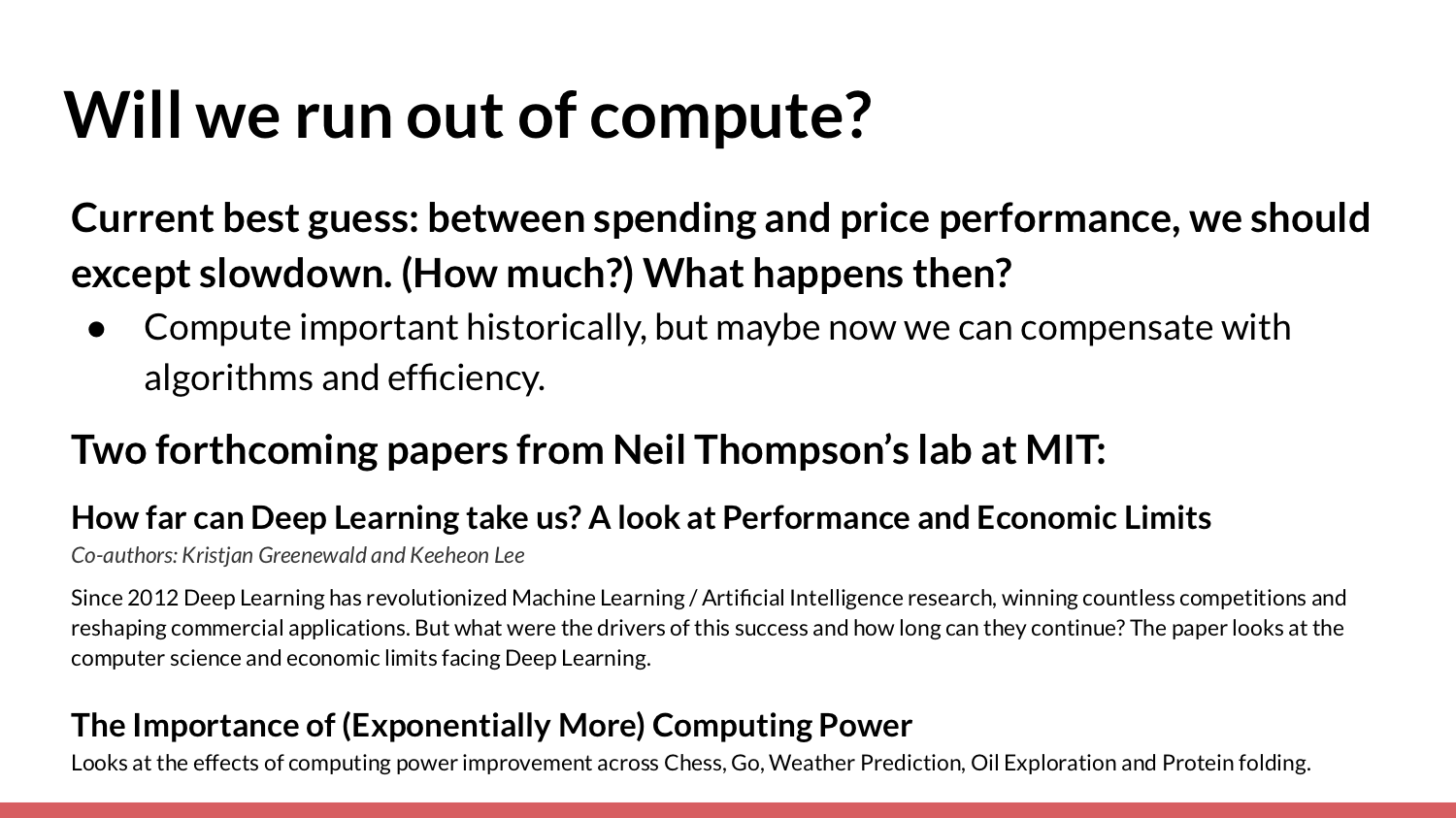
My current best guess is that, given the impossibility [of spending continuing to increase] and the unlikeliness of price performance matching the gains we've seen in the past, we should expect the growth of compute to slow down. As I mentioned, it's very important to determine exactly how much. Then the question becomes: If this trend does slow down, then what happens?
Historically, compute has definitely been important, but perhaps we're now in a period where there's just a steady stream of investment. Perhaps we can compensate for compute that doesn't grow at the same exponential rate with better algorithms and efficiency gains.
On this particular question, I'm excited about two papers coming out of Neil Thompson's lab at MIT. One of them is called “How far can deep learning take us? A look at performance and economic limits.” The other is “The importance of exponentially more computing power,” which will look at the effects of computing power across several important domains. I’m plugging these papers because you should be excited about them, too.
In general, on this compute question, the future is still up in the air. For me, it's the defining question — and the most tractable one right now — in terms of what future progress to expect. I also think what comes out of big companies and startups in the next five years will be a good metric for thinking about AI progress over the next 20 years. I'm really excited to see what happens.
Reason No. 3: Current methods are insufficient
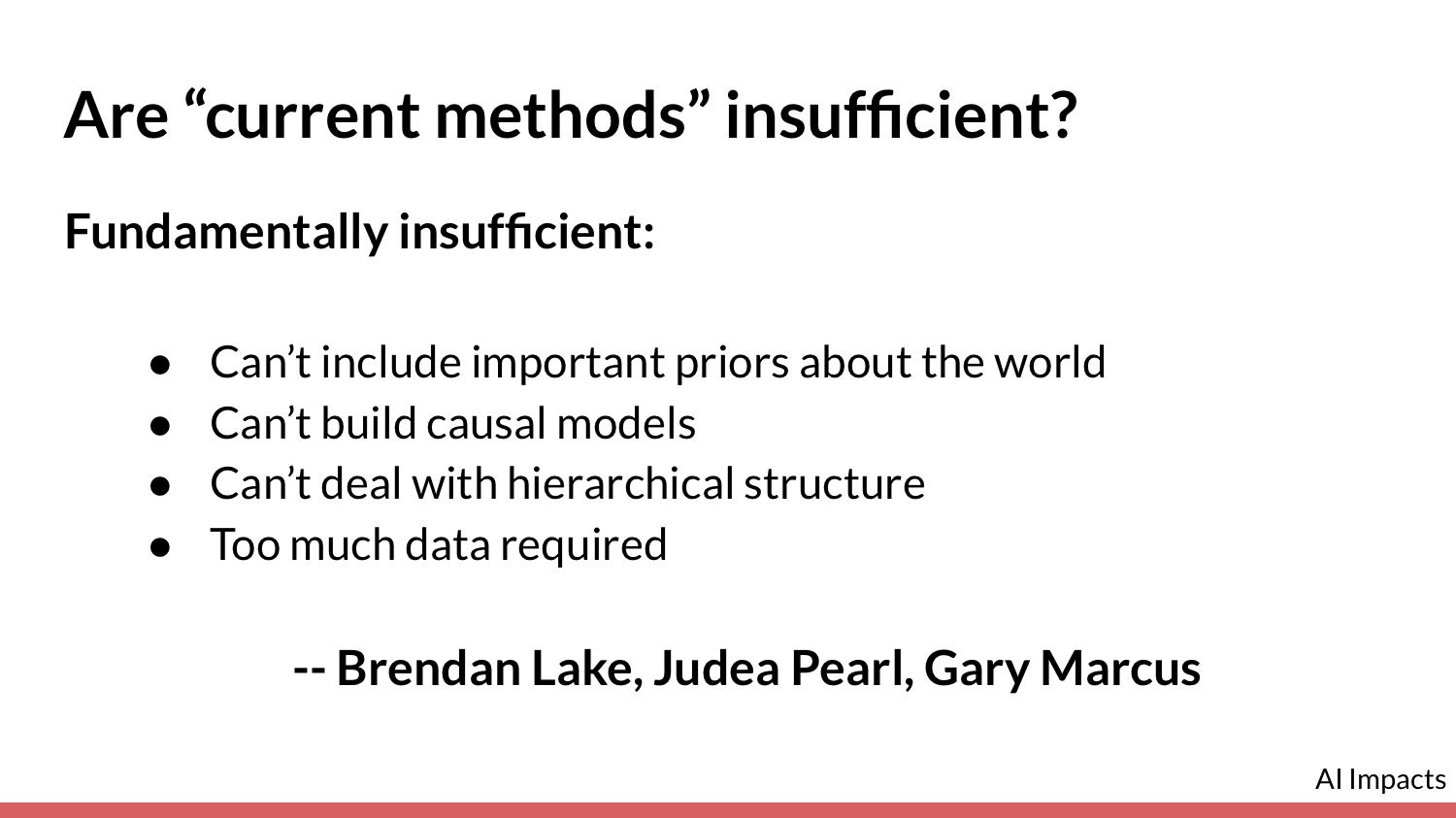
The third reason why you might think we're very unlikely to reach human-level AI in the near term is that current methods (which could refer to deep learning or neural nets in general) will be insufficient. I want to split [this idea] into two categories.
One is that the methods will be fundamentally insufficient somehow. Several legitimate computer scientists have reasons for thinking that, given how current techniques look, we're not going to get to a human level. One reason is that human intelligence relies on many priors about the world, and there’s no clear way to [inject] those priors into neural-network architectures.
Another reason is that we might not think that neural networks are going to be able to build the kind of causal models that human reasoning relies on. Maybe we think that neural networks and deep learning systems won’t be able to deal with hierarchical structure. Maybe we think that we won’t be able to collect all of the data that we would need to train something that's actually human-level.
I don't think I have the technical expertise to evaluate these [theories]. But in my non-expert opinion, we don’t have enough evidence about these methods to be able to say that they're fundamentally insufficient in any of these ways. As with compute, I think in the next five years a lot of [research will likely be released] that sheds light on what we can expect from these methods over the next 20.
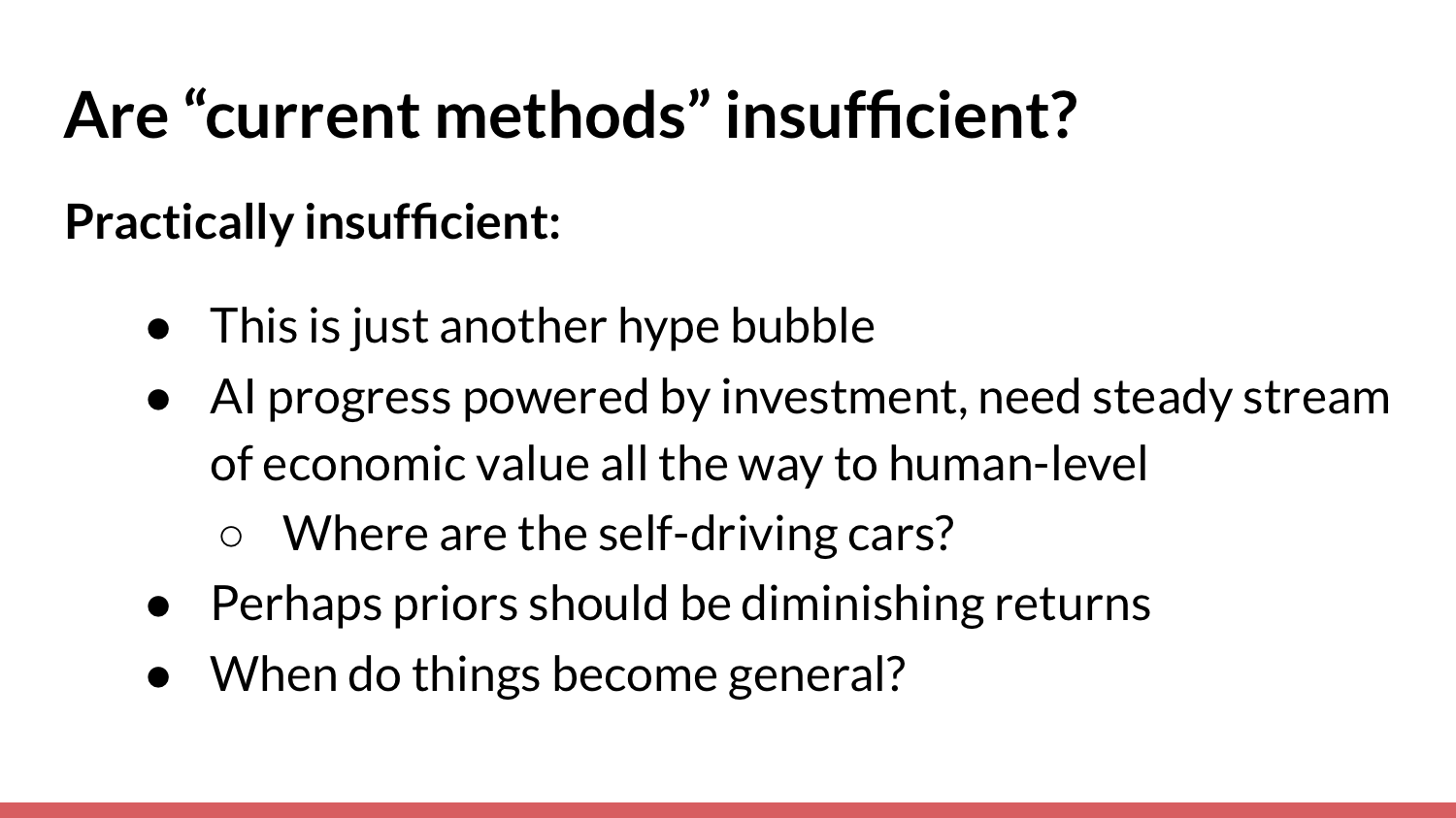
I view the other broad class of “insufficiency reasons” as the argument that current methods might be insufficient from a practicality standpoint — that we could get to human-level AI with neural nets and deep learning methods, but we won't because it'll just be too difficult. Maybe we're in a bubble of hype and investment. Given that AI progress has to be powered by investment, we need a steady stream of money flowing in, which means a steady stream of economic value encouraging people to spend more money.
People often give the example of self-driving cars as an advance that we thought [would become mainstream] years ago, but has taken much longer than we anticipated. Maybe you can generalize from there, and claim that many human tasks are very difficult to automate — and that it will be a long time before we can get value out of automating them. You might think that investment will dry up once we successfully automate the small set of human tasks that are easy for neural networks to automate.
Another general argument that people use is the idea that in scientific fields we should expect diminishing returns. We should expect fewer good things [to emerge from] neural networks and deep learning over time. It's hard to use this argument, partially because it's not clear when we should expect those diminishing returns to kick in. Maybe we should expect that to happen after we get to human-level AI. Although it has historically seemed somewhat true that we should expect diminishing returns in a lot of fields.
The last crux I want to point to [of the “practicality” argument] relates to [what people believe about] the amount of work that we need before we can create a system that's fairly general. One model of AI progress suggests that we're slowly automating away human jobs, one job at a time. If you think we would need to automate away all jobs before [AI can reach human-level intelligence], then you might think it will take a long time, especially given that we haven't yet automated even the most basic jobs.
There's another model proposing that generality isn't all that far away. This model indicates that once we have something that is essentially general, it will be able to automate a lot of the jobs away. In that case, you're looking for something that approximates the human brain — and you might think that will happen soon. This question of when we arrive at something general, and whether that happens before or after automating everything else, is in people's minds when they disagree about whether [human-level AI is practical or not].
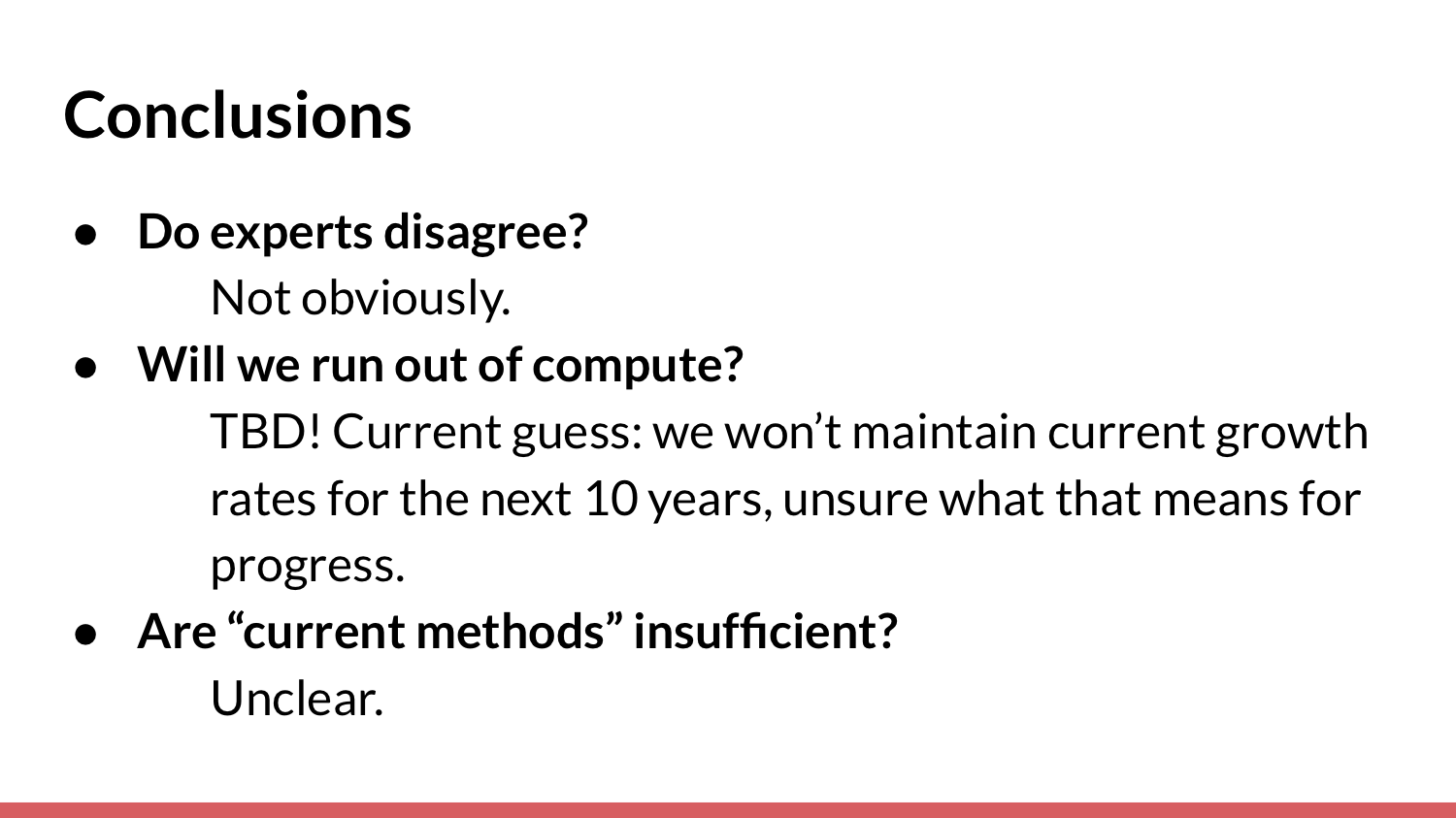
In conclusion, do experts disagree that we could have human-level AI soon? That’s not obvious to me. Even if you only consider the opinions of experts who have worked in the field for a long time, I don't think it's clear that they disagree.
Will we run out of compute? I’m still working on this one. My current guess is that we won't maintain our current growth rates for the next 20 years, but I'm not sure that we should expect that to cause progress to slow significantly. That's a harder question to answer.
Then, are current methods insufficient? We don't have evidence now that strongly suggests an answer one way or the other. But again, I do expect a lot of work to emerge in the coming years that I think will shed light on some of these questions. We might have better answers pretty soon.
That was my talk. I hope you enjoyed it. If you have questions, think I was wrong about something, or want clarification on anything, please feel free to [reach out to me] — especially if your question doesn't get answered in the upcoming Q and A. I try to be very approachable and responsive.
Aaron: Thank you for that talk, Asya. We’ve had a number of questions submitted already, so let's kick off the Q and A with the first one: What have you changed your mind about recently?
Asya: I only recently looked carefully at the data I shared in this talk on compute trends. Before that, I was thinking, “Well, who knows how fast these things are improving? Maybe we will just compensate by increasing price performance a lot.”
Seeing the new data — and that, at least so far, a lot of these hardware startups haven't been that successful — made me feel a little more skeptical about the future hardware situation. Again, that's very tentative. I'll change my mind again next week.
Aaron: Yes, and who knows what else quantum computing or such things could bring about?
If you had six or more months for further research, what would your priorities be — or what might someone else interested in this topic [focus on]?
Asya: Hopefully, I will do future research. I’m quite interested in the compute question, and there are several things you can do to try to estimate the effects. You can talk to people and look at what specific improvements have led to in other fields.
There's a lot of economic data on how much you have to spend on specialized hardware to see efficiency improvements. Looking at historical data on specializations like Bitcoin could provide some idea of what we can expect hardware specialization to look like for deep learning. Again, this is very new. We'll see what I end up looking into.
Aaron: Sounds cool. How useful do you think economic growth literature is for forecasting AGI [artificial general intelligence] development, in terms of timelines?
Asya: I've been looking into this recently. The macroeconomics work that I've seen [comprises] a lot of abstract models with different variables and guesses as to how AI will affect automation, how automation will affect several other parameters, and how those will affect economic growth. They're usually very theoretical and involve guessing at a broad swath of [possibilities].
This might be very difficult, but I'd be interested in more empirical work — specifically, the particulars of automation in the supply chain in AI. What would we expect to be automated? At what rate would we expect jobs to be automated? One common empirical model is based on automating away a constant fraction of jobs every year, or something to that effect. I don't know if that's a reasonable assumption.
If we had more empirical data, I'd be pretty excited about economic growth modeling. That requires work beyond the growth models that exist right now.
Aaron: Sounds perfect. Will the future of compute also be limited by pure physics? Faster chips are now seven nanometers [one billionth of a meter], but we can't shrink that indefinitely. Will that potentially limit the growth toward AGI?
Asya: There are definitely pure, Moore’s-law physical limits, especially once we get past specialization. You mentioned a wide swath of more exotic architectures that we can exploit before the physical limits become the most relevant factor — things like quantum, optical, stacking transistors in 3-D space, etc. I think we can [leverage] improvements from those before we have to worry about the fact that we are hitting physical limits in transistor density.
But I do think it's relevant. We shouldn't expect progress at the same rates that we used to see, because we really just can't do that anymore.
Aaron: Totally makes sense. Do you think that figuring out when we can expect discontinuous progress in AI can tell us much about whether AGI will happen soon?
Asya: It does somewhat, especially if you have a concrete metric in mind and if you don't think progress will be discontinuous, which the AI Impacts investigation suggests is unlikely (but it could be likely). Then, if you want to see continuous progress that leads to AGI in the next 20 years, you have to expect signs of fairly steep, exponential growth.
If you're not seeing those signs, and you think progress is likely to be continuous (i.e. there's not going to be a huge jump), you shouldn’t expect AGI to come soon.
So, I think it should influence people's opinions, especially if we expect something like the economic value from AI or AGI to increase continuously, and it's not increasing very much. In that case, maybe we can say something about what the future looks like.
Aaron: Sounds good. Even if AGI isn't near, shouldn't we worry about the side effects of increasingly powerful machine learning shaping our world with misaligned incentives?
Asya: We totally should. When I’m thinking about this, I consider the more nebulous risks that you get into with superhuman agents. A subset of the literature on AI risk concerns is very focused on those. In some sense, they are some of the most neglected — and the most likely to be existentially bad.
I definitely think powerful machine learning systems of various kinds that are not at a human level can be transformative and quite impactful. It's societally worth thinking about that for sure.
Aaron: Perfect. What do you think about AI talent as another factor in AI development? Will the decoupling between the US and China slow down AI research?
Asya: I should qualify my answer: I'm probably not the most qualified person to talk about AI talent in the US and China. I'm not sure if we should expect it to slow down research, because I'm not sure that it was particularly coupled before in a way that was essential for research. Therefore, I'm not sure that the decoupling should imply a slowdown, but again, I'm definitely not an expert in either AI talent or China. Don't take my opinions on this too seriously.
Aaron: Makes sense. Are there any specific, “canary in the coal mine” advances that we can [use to determine] that human-level AI will happen within 10 years?
Asya: I don't know — perhaps if there was good evidence that a lot of the theoretical problems that people think are true of deep learning systems were shown to be untrue (for example, if we suddenly felt like we could create really good simulation environments, so that training wasn't a problem). Anything that knocks out problems that are [impossible to chart with] causal modeling or hierarchical structures because they need too much data would make me much more optimistic about deep learning methods getting us to AGI in 20 years.
Aaron: Makes sense. Do you think using forecasting methods similar to the ones you've used could help predict when another AI winter could potentially happen?
Asya: I'm not really sure that I would say “similar to the ones I've used.” The “AI winter” part is a function of investment. Right now, investment looks to be exponentially increasing. Post-coronavirus, it would be interesting to see what investment looks like. That will be the type of thing that I'll be keeping track of in terms of predicting an AI winter: investment levels and how many researchers are entering machine learning PhD programs.
Aaron: Makes sense. What do you think the policy implications are for different timelines of AI progress?
Asya: That's a really good question. I think it's probable that if AI becomes a strategically important technology, there will be some government involvement. Then there's a question of what the government will actually do. One function of the government is to legislate the things that are inputs to AI: AI labs, compute manufacturers in the US, etc.
If timelines are short, given that the policy world moves rather slowly, it's important to be on the ground and figuring that out now. but if timelines are long, we have more time for things like institutional reform, and don't need to be in as much of a rush getting laws, cooperation mechanisms, and things like that implemented now.
Aaron: Cool. Expert surveys like the one you cited in your talk are often used as a starting place, such as in Toby Ord's The Precipice. Do you think there should be a revised version of the survey every year? Is it an appropriate starting point for researchers such as yourself to use these estimates?
Asya: It's not unreasonable if you don't know something about a field to ask experts what they think. From that perspective, I don't know that we have a better starting point for random forecasting exercises.
Should such a survey be done every year? It would be cool if it were. It would certainly be interesting to know how expert opinion is changing. But I don't know that we should if better forecasting tools [emerge]. If we are able to analyze economic trends and things like compute, I’m more inclined to endorse that as a starting point. As I mentioned in the talk, I've become pretty skeptical of surveys of experts. I'm not sure that a random technical expert actually has a more informed view than we do.
I do think it's an easy way to determine a good starting point. I think I do endorse frequent surveys of this type. If nothing else, it's interesting to see technical researchers’ perceptions of progress; that's a valuable data point in and of itself, even if it doesn't tell us anything about our true progress.
Aaron: Yes. Is it possible that current spending on AI as a percentage of GDP will stay constant, or even diminish, because of the huge gains in GDP we might expect from investment in AI?
Asya: Over the long term that's very possible. I don't expect it in the short term. But you could imagine in a world where AI contributes to huge economic growth — where we spend more on AI, but GDP increases because we're actually spending less in terms of a percentage. I do think that's possible, but I would find it pretty unlikely in the short term.
Aaron: Do timescales change the effect of interventions for suffering-focused or s-risks in your opinion?
Asya: That's another good question that I haven't thought about at all. I don't know. Wow, this is going to reveal how little I've thought about s-risks. At least some of the work on s-risks is related to work on AGI in that we don't want astronomical amounts of suffering to be created. In the same way that we are, even without S-risks, worried about risks from AGI, there are similar implications to the timescales on AGI that are related to s-risks. I'm not very well-read on the s-risk space.
Aaron: Perfect. I think we have time for one last question: Given the uncertainty around AGI and timelines, would you advise donors to hold off on assisting AI safety organizations?
Asya: I think not. If work seems as though it’s plausibly good, it’s [worthwhile to donate]. I think direct work is often instrumentally useful for [guiding] future direct work and for things like movement-building. Therefore, I would not hold off on funding safety work now — especially safety work that seems good or promising.
Aaron: Thank you so much, Asya. That concludes the Q and A part of the session.
In case anyone else is looking for this, it seems to have been published as The Computational Limits of Deep Learning.
Lousy paper, IMO. There is much more relevant and informative research on compute scaling than that.