Lightning talks are a chance to be introduced to a topic, and these four lightning talks provide primers into interesting and valuable areas. Recorded at Effective Altruism Global 2018: San Francisco, these are four lightning talks on Goodhart’s Law, global food security, normative uncertainty, and forecasting future events. Transcripts of the lightning talks are below, which we have lightly edited for clarity.
Scott Garrabrant: Variations of Goodhart's Law
Goodhart's law is often abbreviated to say, "When a measure becomes a target, it ceases to be a good measure." So you can think about that as, you have some goal V, that is something that you want. And you have some measure of V which is U, which is something that you're actually able to measure.
And then you start optimizing U, and the process of optimizing U breaks the connection between U and V. This is relevant to EAs because we care about things and we measure things and we optimize things. And this is all about how that goes badly. I'm specifically talking not just about what happens when you have a bad measure, but the act of optimizing breaking the value of your measure.
And this is relevant to thinking about rationality, thinking as an individual human, and thinking about organizations, and also also for thinking about AI alignment. I want to show that there are four categories of different ways in which this can happen.
The first is regressional. This is one very benign. This is when you were optimizing for the proxy, and the proxy is not exactly the real goal. When you make the proxy large, you are making the real goal large, but you're also putting some optimization pressure towards the difference between your proxy and your real goal. Which means that your proxy will reliably be an overestimate of your real goal.
Next is causal. This is basically "correlation is not causation." If you observe that your proxy is tied in with your goal, and then you start optimizing for the thing that is your proxy, that connection might no longer happen because what actually happened was maybe some third thing caused both of them. And then when you increase your proxy, your goal fails to also increase.
The next two are more interesting. Extremal is about how the worlds in which the proxy are very large might be meaningfully different from the worlds in which you observe the correlation between the proxy and the goal. This is much less benign than the regressional case. Like you could imagine that under small optimization power, there's a connection between your proxy and your goal. But maybe as your optimization gets very strong, you might be in a different domain. Where instead of naturally occurring correlations being the thing that affects stuff, you have resource limitations being the thing that affects stuff. And then all the observations that you made that originally connected your proxy and your goal kind of go away.
The last one is adversarial. So this is saying that the act of optimizing for your proxy, U, is providing an incentive for other agents to connect things that they want with the thing that you're optimizing, right? If I'm going to optimize for something and you want some other thing, you want to connect the thing that you want to the thing that I'm optimizing for. And if you think about, well maybe my goal requires some resources, and your true goal also requires some resources, but my proxy isn't really fighting for resources the same way that we are. If I'm using that proxy to optimize, then you're incentivized to make the world such that, when my proxy is large, actually your goals are satisfied instead, which destroys the connection with my goal.
As quick examples in basketball, regressional is just, if you take some really tall person, even though height is connected to basketball ability, they will not be the best at basketball, even compared to somebody who's maybe a little bit shorter but has more basketball ability. Causal is, if you want to get tall, it won't actually help to practice basketball. Extremal is like, the tallest person in the world actually has a disease which makes them unable to play basketball, because they're wearing leg braces. And adversarial is well, if you're measuring something about height, people are incentivized to do things like cheat your metric, because their goal is to get into your basketball team. That's it. Thank you.
Finan Adamson: ALLFED
I'm here to talk about ALLFED, the Alliance to Feed the Earth in Disasters. So far, that's mostly research from Dave Denkenberger and Joshua Pearce. We're focused on two kinds of scenarios, scenarios in which the sun is blocked, and scenarios in which industry fails, so in which the power grid fails for a long time. The sun could be blocked by things like nuclear winter, a supervolcano, or asteroid impact.
We could have industry failure from things like high altitude electromagnetic pulse from nuclear weapons, a solar flare, or a cyber attack. So our goal is to feed everyone in these disasters. We've done some research in the technical solutions here of how to feed everyone in industry disabled scenarios, which I'll go a bit into in this talk. Much of the past and current policy around preparing for this kind of disaster is just storing food. That has a couple of problems. One, we only have about six months of stored food globally, and many of these disasters could cause problems longer than that. And if we were to invest a lot of money in storing more food now, that would drive up the price of food, causing more people to starve in the present.
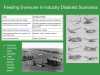
One way that agriculture might look in an industry disabled scenario is like pre-industrial agriculture, which was about 60% lower than modern agriculture. We do have some advantages. We understand a little bit more about fertilizer and the world is more connected, so transferring food between places would be a little easier. It would take time to let people build their skills, because we would need more people farming and to build tools necessary for doing this, but we could use stored food during that transition period.
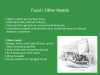
The main thing we'd be doing in this, would be planting higher calorie per hectare crops. And we could clear some lands for more farmland. Some non-food needs are fairly simple in industry disabled scenarios. You can build pit latrines, you can boil water, you can make soap from animal fat. One of the non-food needs that is not simple is transporting food, which is something that would be necessary. Ships can be retrofitted with sails if there is some fuel available. If there's no fuel available, then traditional sailing still works.
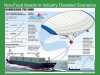
So we've done some back of the envelope calculations about what we could do. So we think with about 30 million dollars, a shortwave radio system for backup communication could be developed. Manuals for producing food and other needs could be developed and tested. And we could get response plans available to governments and other organizations. So you may have seen our cost effectiveness model out during the poster session. There will be another one at seven, that's for the sun block scenarios. We're developing another cost effectiveness model for industry disabled scenarios, and that's something you could help us with.
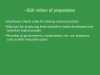
So I'm going to take a quick audience poll. So for each, I'll ask these questions one at a time, and then for each category I'm going to have you raise your hands for more than 10%, between one and 10%, 0.1 and 1%, and less than 0.1%.
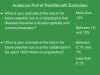
So the first question is, what is your estimate of the loss in far future potential due to a catastrophe that disables industrial civilization globally with current preparations? So that's saying, as is right now, an industry disabled scenario happens, how much of the future potential is lost?
And for the second question, what is your estimate of the loss in far future potential due to a catastrophe if we spent $30 million on preparation? So we enact the things in the last slide. How much of the future is lost if such a disaster occurs then?
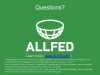
And I believe that's all the time we have. Feel free to go to our website if you want. You can check out our research there.
Philip Trammell: Normative Uncertainty
Right. We're all familiar about the idea of being uncertain about the true state of the world. That includes being uncertain about what the observable consequences of our actions will be. But we can also be unsure about what we ought to do, even given well-specified beliefs about the state of the world. Like we know it's going to happen and we have a precise probability distribution over what we think will happen. We can still be uncertain about what we should do. That's called normative uncertainty.
Two kinds of normative uncertainty that would be decision-affecting are moral uncertainty and decision theoretic uncertainty. So for instance, we might come across an opportunity to tell a lie and save a life, and even though we know exactly what the consequences would be, we just don't know what true morality is. It might be the right thing to do that or the wrong thing.
We might also be unsure about what the right way is to respond to uncertainty about the state of the world. So, whether we should maximize expected utility, as one decision theory would say, the most commonly accepted one. Or whether we should do something else, like be really risk averse and be min-max, or minimize the probability of the worst possible outcome.
It's relatively common in the EA community to think that we should take normative uncertainty seriously. We should think about our credences in the normative standards of behavior, and incorporate this uncertainty into our decision making. Will MacAskill and Toby Ord are EA philosophers who specialize in normative uncertainty. That means there's a lot of EA brain power going into this research question.
Some people have raised an objection to the whole concept of normative uncertainty, which is that it seems to lead into an infinite regress. The idea is, if we don't know what we ought to do in the face of uncertainty about what we ought to do, then it's like, what ought we to do if we don't know what we ought to about what we ought to do? And so maybe the whole concept of normative uncertainty is incoherent. But it feels like it's not. It feels like I can be unsure about what the right moral or decision theoretic framework is. So that's something I spent some time thinking about.
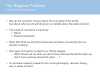
Now the solution that I think makes the most sense is to say that you do have this infinite regress, but it reaches a fixed point for one reason or another. And so you have well defined subjective choice-worthiness, as they say, for all the acts that you could perform, whether you should tell the lie to save a life or whatever. This is a little stylized, but let's say you had the option to give $5,000 to the AMF to save a life for sure (assume you believe this will work), or the option to give the $5,000 to a biosecurity intervention, which will almost certainly save zero lives, but which has a one in a billion chance of saving one and a half billion lives.
So this is just a simple case of decision theoretic uncertainty. Let's also say you're certain that moral value is linear in lives saved, to make things simple. But you're just not sure whether to maximize the expected value or something else. So what expected utility theory would say is that what you ought to do is maximize the expected value. And so in this framework, what expected utility theory says is that the first order meta-choice-worthiness of the risky intervention is the same as its expected value, so in this case it's 1.5. And that's higher than the one that you can by giving to the AMF, so you should do it.
But let's say assign a 50% chance to expected utility theory being the normatively correct decision theory, and a 50% chance to some risk-weighted alternative, which says that the objective choice-worthiness of the act should be halfway between the minimum possible outcome that could result and the expected value. According to that alternative risk weighted theory, the subjective choice-worthiness ought to be 0.75, not 1.5. So you're not sure what it should be subjected to.
So what should you do in the face of this normative uncertainty? Let's say you have the same distribution over theories at every order of the hierarchy. You don't have to, but just to simplify, let's say that you do. Well, there's a 50% chance that what you ought to do in the face of this first order uncertainty is take the expected value. And there's 50% chance that you ought to do some risk-weighted thing, and so on.
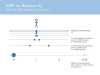
And this can go on forever, and as long as certain mathematical properties are met about the hierarchy, then it will converge, and the subjective choice worthiness of the act will be well defined. And in this case it will equal one. I mean, I set it up so that it would equal one. And so you would be indifferent between the risky intervention but with higher expected value and the $5,000 to the AMF. So this is all stylized and abstract, but the point is just that on its own the regress problem is not a knock down objection to the whole concept of normative uncertainty.
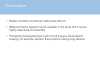
Your hierarchy of meta-normative uncertainty could, if it satisfies some conditions like always compromising between the points of the orders below it for instance, you'll always reach convergence. We can go a lot more into it, but I think that's basically the main takeaway. Maybe normative uncertainty makes sense after all. Maybe research into all of this, all of this theorizing about what one ought to do in the face of uncertainty or meta-normative uncertainty is actually valuable. And what the value is falls out of the model naturally, so you can work out like, "Oh I should spend 30% of my time reading a decision theory book before I make this really momentous decision," or like 10%, or whatever. So that follows from the framework. And so maybe there's some value to it.
Lawrence Chan: Criteria for Good Forecasts
A lot of people in EA care about forecasting and having good and calibrated forecasts. And historically, a lot of people around the world, in universities, in the intelligence community have also cared about this.
But we spend a lot of time focusing mainly on how do we improve forecasts, given the current metrics for good forecasts that we have already? And most of the metrics that we have are based on calibration or correspondence-based scores. Basically like, here's what the true outcome was, here's what your probability that you assigned at a given time was, now let's see the sort of comparison. So like that classical Brier or log-based scores fall into this category. But in reality when we make forecasts over time, there are other desiderata that we might want to satisfy.
Like, we might need to satisfy other criteria, basically. And I think a classic illustrative example would be, suppose I'm trying to forecast what the weather would be like in two weeks. So on June 24th, what's the weather like in San Francisco? I might plot a chart of the probability I assign to it being sunny. Today I might say, it's pretty sunny outside, so I presume it's going to be sunny in two weeks, and I assign a very high probability, say 90%.
And then tomorrow I don't feel very well, I might just give a 10% probability, and then the day after I might be very happy, I'll say 95%, and then the next day like 5%. People can basically see that, just looking at this sort of probability estimate, that there's probably something not correct about it. And in fact you can actually quantify how incorrect this is from a certain perspective.
So in economics, when people study financial markets, there's an idea of an efficient financial market. So one where you can't predict expected price changes. Or the expected price of any given commodity. This is the notion of efficiency in the economic sense. And this sort of belief is probably not efficient, right? Because you can just look at this and then say, "Oh if my belief is over 90% tomorrow, like today, it's probably going to be under 20% tomorrow" and vice versa.
And so using this as an inspiration you can actually develop a lot of tests by going to the economics literature and looking at what sort of properties do efficient beliefs or efficient markets need to satisfy. And I'll briefly go over three of them right now.
So there's the classic, you shouldn't expect to predict which direction your beliefs, or in which direction your prices will move. So basically you can just try to approximate this expectation, or you can try to approximate the difference conditioned on the current information available to you. And you can just check if it's zero. So you shouldn't expect any price changes or any belief changes. Basically if I know that my belief is going to be 10% tomorrow and I think I'm not going to be crazy tomorrow, and I'm still going to have good reason for believing that it's 10% tomorrow, I should just believe that it's 10% already today.
And likewise, there's also a sense in which my belief shouldn't move around too much. If we see that my beliefs go from 90% today to 10% tomorrow to 95% to 5%, there's a sense in which this is just moving around too much. And you can actually quantify this sense and then perform tests on this. And finally, what people actually do a lot in economics literature is they devise some trick in through which they can make some money in the market, and then attempt to check whether or not this trick actually works.
So you can do the same thing. You can try to think if there is a system of bets that you as a forecaster would take, that would lose you money, even though the system of bets only uses information available to you. And then so basically if you fail those tests, if you make bets that you know you're going to lose money in, that probably means that you're not doing a good job forecasting.
So for example, checking whether or not the expected price change is one way to make money, but you could also sell straddles, or you can do things like, I'll commit to buying at a certain point and then I'll commit to selling at a certain point. And often times formulating in terms of strategies to exploit the market is more natural for humans than going up there and then trying to do the math in your head, whether or not the expectation is equal to the current value.