Redwood Research is running another iteration of MLAB, our bootcamp aimed at helping people who are interested in AI alignment learn about machine learning, with a focus on ML skills and concepts that are relevant to doing the kinds of alignment research that we think seem most leveraged for reducing AI x-risk. We co-organized the last iteration of the bootcamp with Lightcone in January, and there were 28 participants. The program was rated highly (see below for more), and several participants are now working full-time on alignment. We expect to start on Aug 15 but might push it back or forward by a week depending on applicant availability.
Apply here by May 27.
We’re expecting to have space for about 40 participants. We’ll pay for housing, travel, and food, as well as salaries for the TAs. We will help you get visas to travel to the US for the bootcamp.
We’re now accepting applications for participants and TAs. TAs are expected to either know this material already or have a month free before MLAB to study all the content.
Last time the schedule was roughly the following:
- Prep work: Pytorch array programming
- Week 1: Pytorch, optimization
- Implement a renderer in pytorch, as an exercise in mathematical array programming
- Implement ResNet from scratch in pytorch, implementing all the layers from scratch and loading weights from a trained model.
- Implement interpretability techniques on the ResNet.
- Implement SGD and other local optimization algorithms, run remote hyperparameter searches on a simple architecture
- Implement a simple clone of some of Pytorch, with particular focus on the implementation of backpropagation
- (Optional) CUDA programming day–write various CUDA kernels, see how close to the performance of Pytorch’s kernels you can get
- Week 2: Transformers
- Implement BERT from scratch, load weights from the real pretrained BERT
- Implement GPT-2, implement beam search
- Fine tune BERT on classification, fine-tune GPT-2 on some specific corpus
- Look at various interpretability techniques on GPT-2
- Data-parallel training
- Week 3
- Pipeline parallelism
- Tensor parallelism
- Deep RL (DQN, policy gradient)
- RL algorithms on language models
- More transformer interpretability
- (Optional) ELK day
- Week 4: Optional final projects week, Q&As with various alignment researchers
This time, we’ll probably have more systematic transformer interpretability content, because we’ve spent a lot of time since MLAB doing our own transformer interpretability research and have a bunch more opinions now. We might also have more systematic content on various relevant math. I’m also hoping that we’ll be able to cover content more efficiently as a result of experience gained from running the program the first time.
Past participants report that MLAB was time-consuming; we strongly recommend against trying to juggle other commitments concurrently. About 8 hours a day, 5 or 6 (if you participate in the optional day) days a week will be spent on pair programming, in addition to daily lectures and readings. There is a lot of content packed into each day; not everyone will finish every part of the curriculum. We aim to create a learning environment that is focused but not frantic; we’d rather have you understand the material deeply than finish 100% of the day’s content.
The program is aimed at people who are already strong programmers who are comfortable with about one year’s worth of university level applied math (e.g. you should know what eigenvalues and eigenvectors of a matrix are, and you should know basic vector calculus; in this course you’ll have to think about Jacobian matrices and make heavy use of tensor diagram notation, so you should be able to pick up both of those pretty fast).
We expect that about half the attendees will be current students (either undergrad or grad students) and half will be professionals.
If you applied to the first cohort and were not accepted, consider applying again. We had many more applicants than spots last time.
Last time, we ended up hiring three people who attended MLAB as participants (as well as giving another person an offer that they turned down for a non-alignment EA job), and hired three people who had worked as TAs. Note that about ⅔ of attendees last time were students who were unavailable for immediate employment.
My guess is that MLAB is a pretty great opportunity for people who want to become more familiar with the concepts and practical details related to ML; I think that MLAB is a good use of time for many people who don’t plan to do technical alignment research long term but who intend to do theoretical alignment research or work on other things where being knowledgeable about ML techniques is useful.
TA-ing MLAB is a good opportunity for people with more prior knowledge of this material to connect with Redwood Research and the broader Bay Area alignment community, reinforce their understanding of the curriculum material, and movement-build by teaching others. It also pays competitively.
Highlights from the end-of-MLAB survey last time
MLAB was well received:
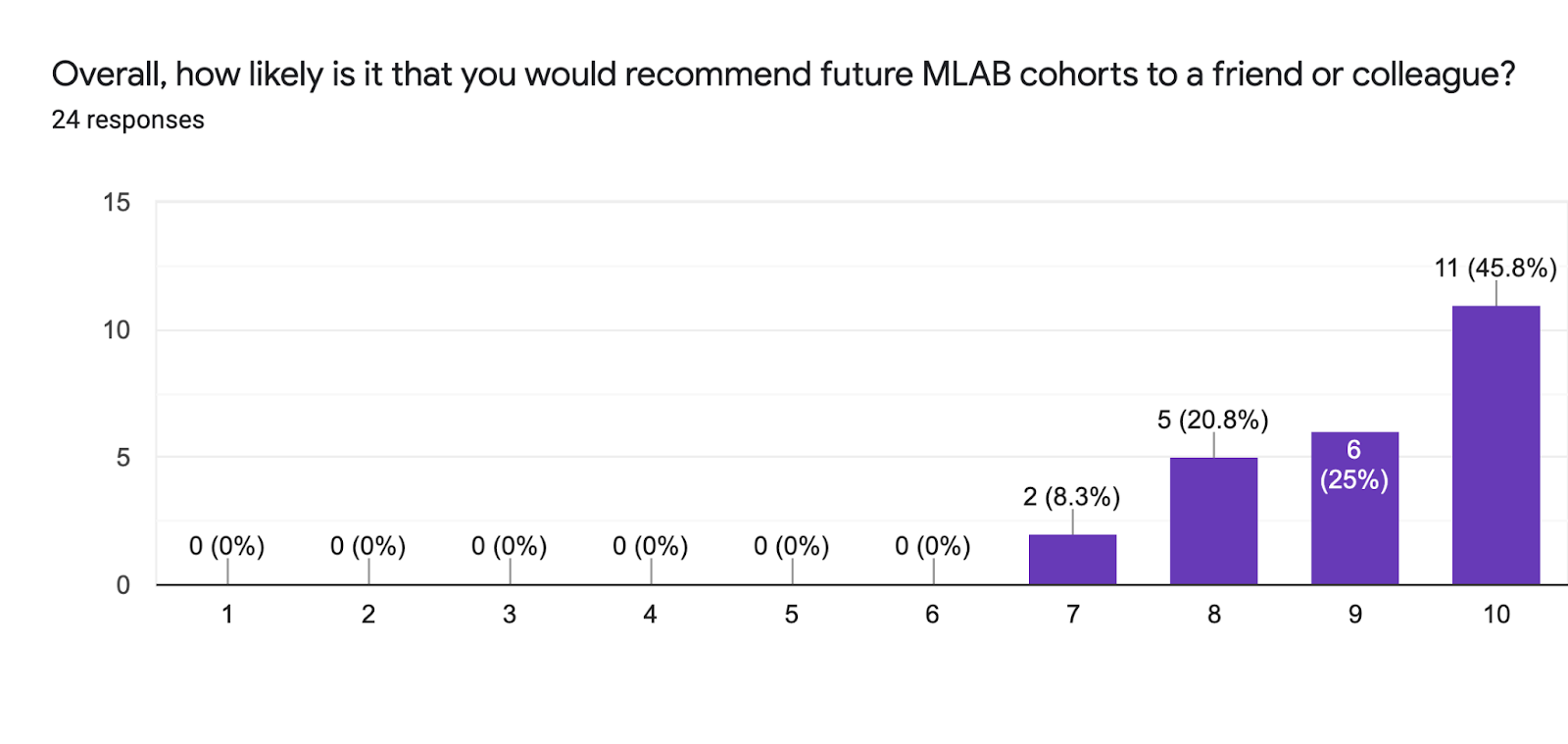
When we asked participants what they were surprised by, major themes were:
- People thought it was more useful than they expected.
- Many people were surprised by how much they liked the focus on implementing things.
- Several people were concerned that their ML background was too strong or too weak, and were pleasantly surprised by the extent to which the content was valuable anyway.
- People were surprised that there wasn’t more content on alignment in the main curriculum. The content is basically all related to understanding and working with ML systems. This focus is because I think that doing alignment research with ML systems basically just requires the same skills as understanding these systems more generally, and so you might as well just study the systems directly and apply this knowledge to alignment projects later (except that I think that emphasizing interpretability throughout is actually a pretty good way of learning to understand the systems better in a way that I expect to be useful for a variety of directions of research). There were a variety of Q&As with alignment researchers and quite a lot of casual discussion about alignment.
Logistics
The bootcamp takes place at Constellation, a shared office space in Berkeley for people working on longtermist projects. People from several longtermist organizations often work from the space, including people from Open Philanthropy, MIRI, Redwood Research, the Alignment Research Center, and more.
As a participant, you’d attend semi-regular communal lunches and events at Constellation and have a great opportunity to make friends and connections.
If you join the bootcamp, we’ll pay for travel to Berkeley (for both US and international participants), housing and food.
FAQ (mostly copied from last time)
What if I can’t make these dates? Will there be more bootcamps in future?
Maybe! We encourage you to submit an application even if you can’t make those dates, and it is very much on the table to run future bootcamps like these if there’s an interest and the first one goes well.
How does your curriculum differ from the curriculum other people might have built?
It’s way more focused on learning by implementing small things based on a carefully constructed curriculum, rather than e.g. reading papers or trying to replicate whole papers at once. This difference in focus is mostly because I (Buck) believe that focusing first on these skills makes it much faster to learn, because you get way faster feedback loops. It’s also probably partially due to some of my beliefs about how to do ML research which are slightly unusual among ML people (though many of the ML people I’ve talked to mostly agree with me).
How different is this curriculum from the optimal curriculum for learning the skills required to work on ML stuff at places other than Redwood?
In Buck’s opinion not that different.
What’s the application process?
You fill out the form and then do some online tests then talk to one of us.
How useful is this bootcamp if I’m already somewhat experienced with ML?
I (Buck) would guess that it’s pretty robust to being experienced. I personally feel like I learned some details I’m glad to know from preparing the curriculum, and I’d appreciate having an opportunity to drill a bunch of the skills taught. If you read the curriculum listed above and your response is “yawn, I already know all these things or don’t care about knowing them”, then probably you don’t want to do this bootcamp. I personally enjoyed App Academy quite a lot despite being more experienced than the other students. As I noted above, many people mentioned in the final survey that they were worried that they had too much background and were pleasantly surprised by the extent to which the content was useful anyway.
Am I eligible if I’m not sure I want to do applied ML long-term, because maybe I should do some other kind of work (eg non-applied alignment work, or movement building) instead?
Yes.
Am I eligible if I don’t plan to work in effective altruism, in some way?
Feel free to apply, but the selection process will strongly favour participants who want to work on AI alignment or other parts of effective altruism.
How does this interact with other summer activities?
This overlaps with MLSS by a single week; you’re able to skip that last week of MLSS if necessary, which means that you can do both. You’ll have to sort out other conflicts yourself.
Apply now
You can apply here. Feel free to send questions to Max Nadeau at max@rdwrs.com. Applications close on May 27.
As a fellow graduate of App Academy, I can certainly speak to and strongly recommend the full-time immersive workshop approach to learning a huge amount in a short time. That said, like many people I personally don't have the free time to focus on it full-time just yet. Any chance the curriculum (github repo?) could be made available for asynchronous solo progress?
I'm thinking of organizing an unofficial version of this in Finland. So this would be nice for sure :)
Hey,
Is the material available somewhere so we can study it without the official course?
Thx
Edit: No, the material is not publicly available
If someone doesn't have much prior ML experience, can they still be a TA, assuming they have a month to dedicate to learning the curriculum before the program starts?
If yes, would the TA's learning during that month be self-guided? Or would it take place in a structure/environment similar to the one the students will experience?
It is possible but unlikely that such a person would be a TA. Someone with little prior ML experience would be a better fit as a participant.
This sounds really exciting!
I'm a bit unclear on the below point:
Do you mean you don't think MLAB would be a good use of time for people who do "plan to do technical alignment research long term"?
We intended that sentence to be read as: "In addition to people who plan on doing technical alignment, MLAB can be valuable to other sorts of people (e.g. theoretical researchers)".