Welcome to the AI Safety Newsletter by the Center for AI Safety. We discuss developments in AI and AI safety. No technical background required.
Subscribe here to receive future versions.
OpenAI announces a ‘superalignment’ team
On July 5th, OpenAI announced the ‘Superalignment’ team: a new research team given the goal of aligning superintelligence, and armed with 20% of OpenAI’s compute. In this story, we’ll explain and discuss the team’s strategy.
What is superintelligence? In their announcement, OpenAI distinguishes between ‘artificial general intelligence’ and ‘superintelligence.’ Briefly, ‘artificial general intelligence’ (AGI) is about breadth of performance. Generally intelligent systems perform well on a wide range of cognitive tasks. For example, humans are in many senses generally intelligent: we can learn how to drive a car, take a derivative, or play piano, even though evolution didn’t train us for those tasks. A superintelligent system would not only be generally intelligent, but also much more intelligent than humans. Conservatively, a superintelligence might be to humanity as humanity is to chimps.
‘Solving’ ‘superalignment’ in four years. OpenAI believes that superintelligence could arrive this decade. They also believe that it could cause human extinction if it isn’t aligned. By ‘alignment,’ OpenAI means making sure AI systems act according to human intent. So, ‘superalignment’ means making sure superintelligent AI systems act according to human intent (as opposed to doing alignment super well). The Superalignment team’s stated goal is to “solve the core technical challenges of superintelligence alignment in four years.”
There are two important caveats to this goal. The first is that “human intent” isn’t monolithic. AI safety will have to involve compromise between different human intents. OpenAI knows that their technical work will need to be complemented by AI governance. The second is that alignment may not be a problem able to be conclusively solved once and for all. It might instead be a wicked problem that must be met with varied interventions and ongoing vigilance.
Current alignment techniques don’t scale to superintelligence. OpenAI’s current alignment techniques rely on humans to supervise AI. In one technique, “reinforcement learning from human feedback” (RLHF), humans train AI systems to act well by giving them feedback. RLHF is how OpenAI trained ChatGPT to (usually) avoid generating harmful content.
Humans can generally tell when a less intelligent system is misbehaving. The problem is that humans won’t be able to tell when a superintelligent AI system misbehaves. For example, a superintelligent system might deceive or manipulate human supervisors into giving it positive feedback.
OpenAI’s approach to alignment is to build and scale an automated alignment researcher. OpenAI proposes to avoid the problem of human supervision by automating supervision. Once they have built a “roughly human-level” alignment researcher, OpenAI plans to “iteratively align superintelligence” using vast amounts of compute. By “iterative,” OpenAI means that their first automated alignment researcher could align a relatively more capable system, which could then align an even more capable system, and so on.
OpenAI dedicated 20% of their compute to alignment. OpenAI’s Superalignment team represents the single largest commitment a leading AI lab — or government, for that matter — has made to AI safety research. Still, it may not be enough. For example, Geoffrey Hinton has suggested that AI labs should contribute about 50% of their resources to safety research.
Musk launches xAI
Elon Musk has launched xAI, a new AI company that aims to compete with OpenAI and DeepMind. In this story, we discuss the implications of the launch.
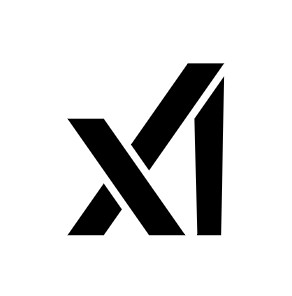
What are xAI’s prospects? Given Musk’s resources, xAI has the potential to challenge OpenAI and DeepMind for a position as a top AI lab. In particular, xAI might be able to draw on the AI infrastructure at Tesla. Tesla is building what it projects to be one of the largest supercomputers in the world by early 2024, and Musk has said that Tesla might offer a cloud computing service.
How will xAI affect AI risk? It’s unclear how the entrance of xAI will affect AI risk. On one hand, the entrance of another top AI lab might exacerbate the competitive pressures. On the other hand, Musk has been one of the earliest public proponents of AI safety. xAI has also listed Dan Hendrycks, the director of CAIS, as an advisor to xAI. (Note: Hendrycks does not have any financial stake in xAI and chose to receive a token $1 salary for his consulting.) xAI has the potential to direct Musk’s resources towards mitigating AI risk. More information about the organization will come out during this Friday’s Twitter spaces with the xAI team.
Developments in Military AI Use
According to a recent Bloomberg article, the Pentagon is testing five large language model (LLM) platforms in military applications. One of these platforms is Scale AI’s Donovan. Also, defense companies are advertising AI-powered drones that can autonomously identify and attack targets.
AI and defense companies are developing LLMs for military use. Several companies, including Palantir Technologies, Anduril Industries, and Scale AI, are developing LLM-based military decision platforms. The Pentagon is currently testing five of these platforms. Scale AI says its new product, Donovan, is one of them.
What are the military applications of LLMs? The Pentagon is testing the LLM platforms for their ability to analyze and present data in natural language. Military decision-makers could make information requests directly through LLM platforms with access to confidential data. Currently, the military relies on much slower processes. Bloomberg reports that one platform took 10 minutes to complete an information request that would have otherwise taken several days.
The Pentagon is also testing the platforms for their ability to propose its own courses of action. Bloomberg was allowed to ask Donovan about a US response to a Chinese invasion of Taiwan. It responded: “Direct US intervention with ground, air and naval forces would probably be necessary."
Scale AI advertises Donovan’s ability to generate novel courses of action.
The use of LLMs follows recent developments in AI-powered drones. AI systems have already been tested and deployed in autonomous flight and targeting. In 2020, DARPA’s AlphaDogfight program produced an AI pilot capable of consistently beating human pilots in simulations. A UN report suggests that the first fully-autonomous drone attack occurred in Libya the same year. The company Elbit Systems is now advertising a similar “search and attack” drone that approaches humans then explodes, and the US may be evaluating AI targeting systems.
Should we be concerned? If LLMs or AI drones give militaries a competitive advantage over their adversaries, then their use might lead to an arms race dynamic. Competing nations might increasingly invest in and deploy frontier AI models. Such a dynamic has the potential to exacerbate AI risk. For example, militaries might lose control over increasingly complex AI systems.
Links:
- The UN Security Council is set to hold its first meeting on the threats of AI to global peace.
- The UK parliament calls for evidence on how to “respond to the opportunities and risks posed by large language models.”
- Similarly, the Australian government asks for advice on mitigating AI risks.
- The director of the CIA is concerned about the accelerating rate of AI capabilities.
- OpenAI releases a code interpreter, which allows ChatGPT to run code.
- Anthropic releases its newest model, Claude 2.
- The Boston Globe interviews the director of CAIS.
- DeepMind explores international AI governance in a new white paper.
- A new paper discusses regulating frontier models.
See also: CAIS website, CAIS twitter, A technical safety research newsletter, and An Overview of Catastrophic AI Risks
Subscribe here to receive future versions.