Summary
- We are a new research organization working on investigating trends in Machine Learning and forecasting the development of Transformative Artificial Intelligence
- This work is done in close collaboration with other organizations, like Rethink Priorities and Open Philanthropy
- We will be hiring for 2-4 full-time roles this summer – more information here
- You can find up-to-date information about Epoch on our website

What is Epoch?
Epoch is a new research organization that works to support AI strategy and improve forecasts around the development of Transformative Artificial Intelligence (TAI) – AI systems that have the potential to have an effect on society as large as that of the industrial revolution.
Our founding team consists of seven members – Jaime Sevilla, Tamay Besiroglu, Lennart Heim, Pablo Villalobos, Eduardo Infante-Roldán, Marius Hobbhahn, and Anson Ho. Collectively, we have backgrounds in Machine Learning, Statistics, Economics, Forecasting, Physics, Computer Engineering, and Software Engineering.
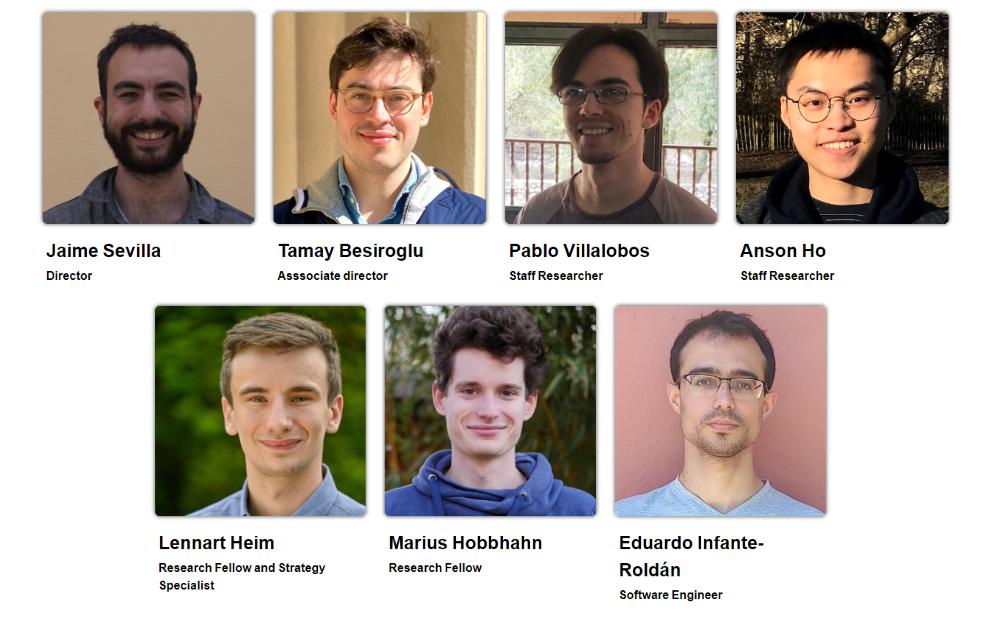
Our work involves close collaboration with other organizations, such as Open Philanthropy, and Rethink Priorities’ AI Governance and Strategy team [1]. We are advised by Tom Davidson from Open Philanthropy and Neil Thompson from MIT CSAIL. Rethink Priorities is also our fiscal sponsor.

Our mission
Epoch seeks to clarify when and how TAI capabilities will be developed.
We see these two problems as core questions for informing AI strategy decisions by grantmakers, policy-makers, and technical researchers.
We believe that to make good progress on these questions we need to advance towards a field of AI forecasting. We are committed to developing tools, gathering data and creating a scientific ecosystem to make collective progress towards this goal.
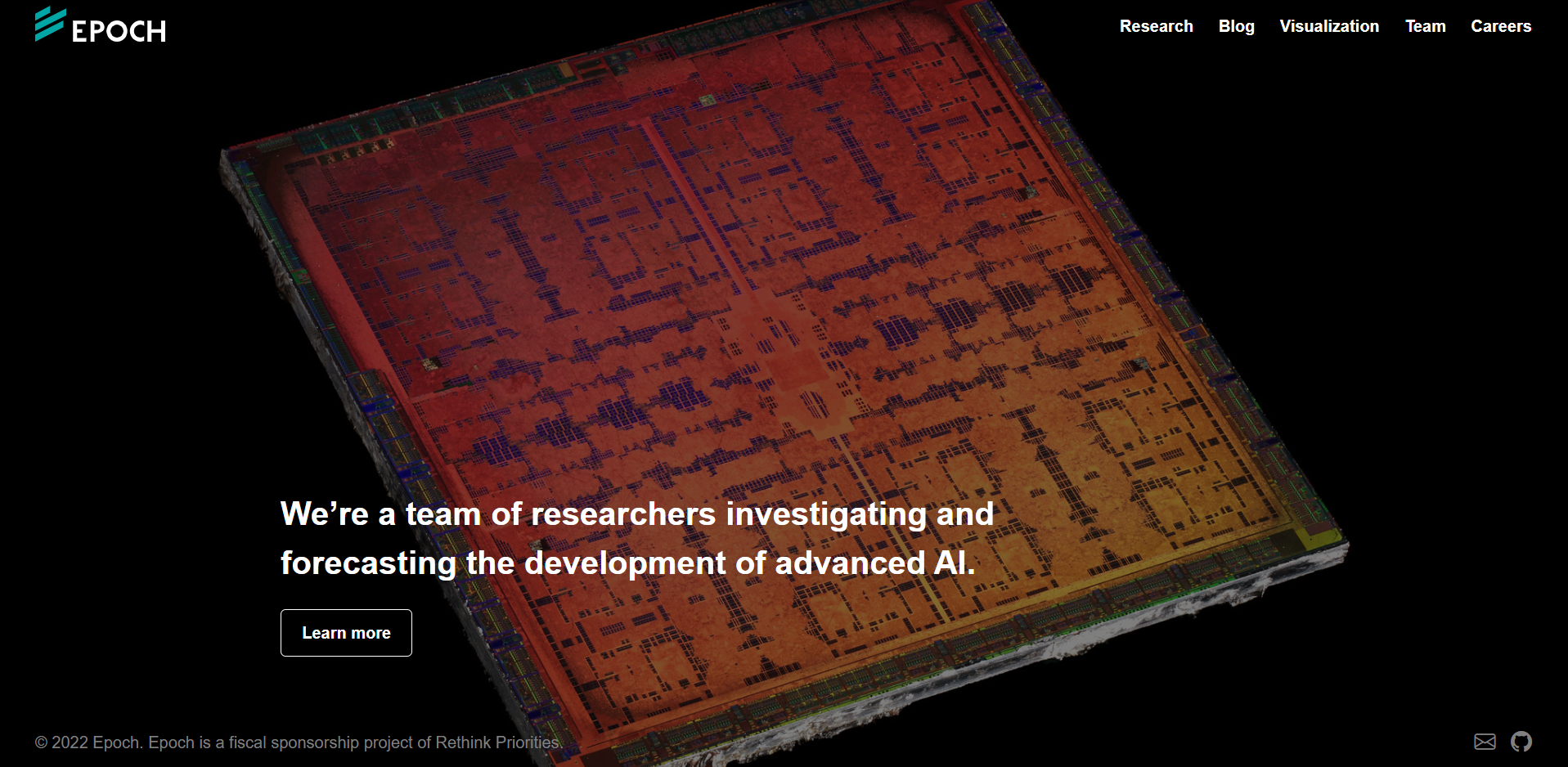
Our research agenda
Our work at Epoch encompasses two interconnected lines of research:
- The analysis of trends in Machine Learning. We aim to gather data on what has been happening in the field during the last two decades, explain it, and extrapolate the results to inform our views on the future of AI.
- The development of quantitative forecasting models related to advanced AI capabilities. We seek to use techniques from economics and statistics to predict when and how fast AI will be developed.
These two research strands feed into each other: the analysis of trends informs the choice of parameters in quantitative models, and the development of these models brings clarity on the most important trends to analyze.
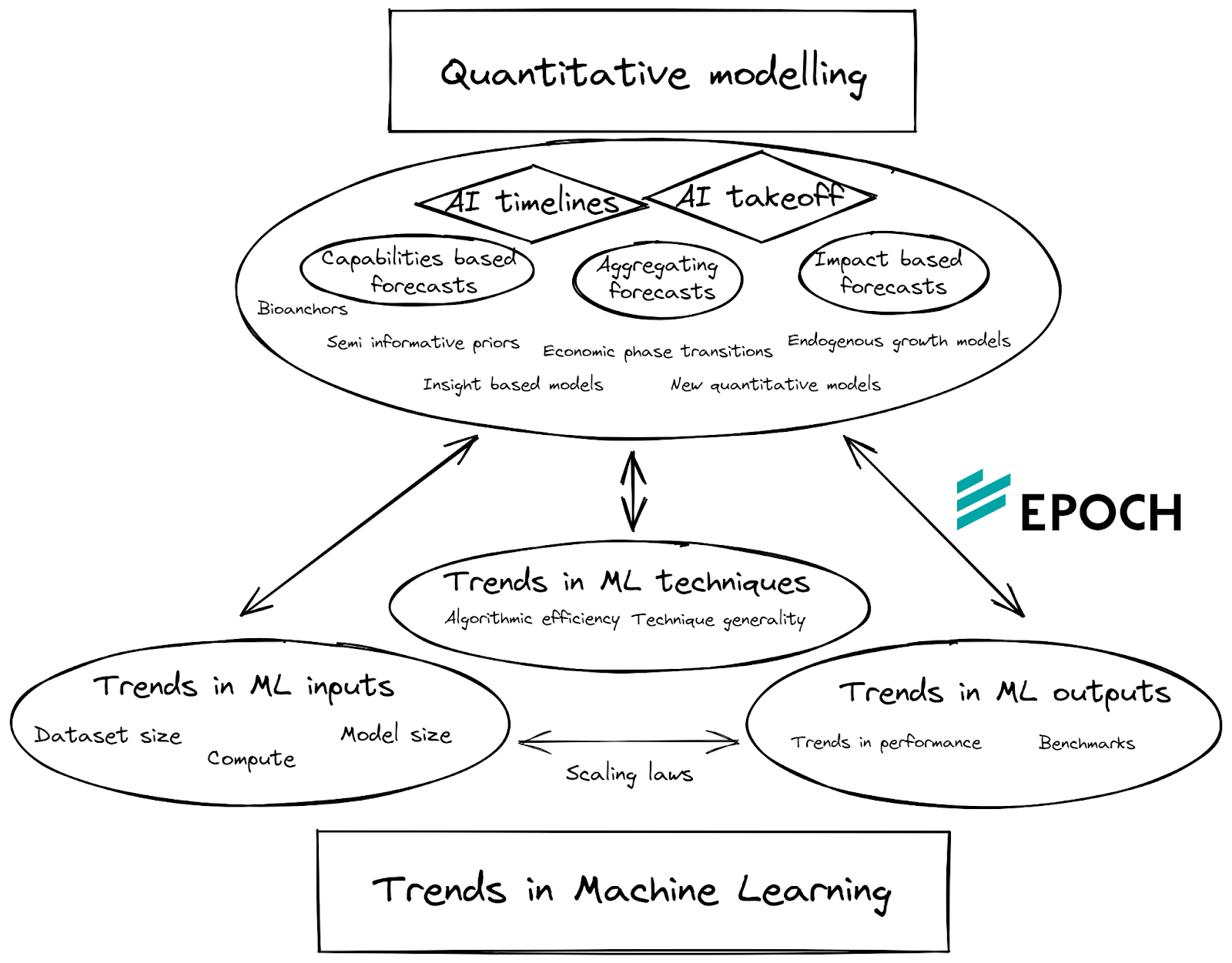
Besides this, we also plan to opportunistically research topics important for AI governance where we are well positioned to do so. These investigations might relate to compute governance, near-term advances in AI and other topics.
Our work so far
Earlier this year we published Compute Trends Across Three Eras of Machine Learning. We collected and analyzed data about the training compute budget of >100 Machine Learning models across history. Consistent with our commitment to field building, we have released the associated dataset and an interactive visualization tool to help other researchers understand these trends better. This work has been featured in Our World in Data, in The Economist and at the OECD.
More recently we have published Grokking “Forecasting TAI with biological anchors” and Grokking “Semi-informative priors over AI timelines”. In these pieces, Anson Ho dissects two popular AI forecasting models. These are the two first installments of a series of articles covering work on quantitative forecasting of when we will develop TAI.
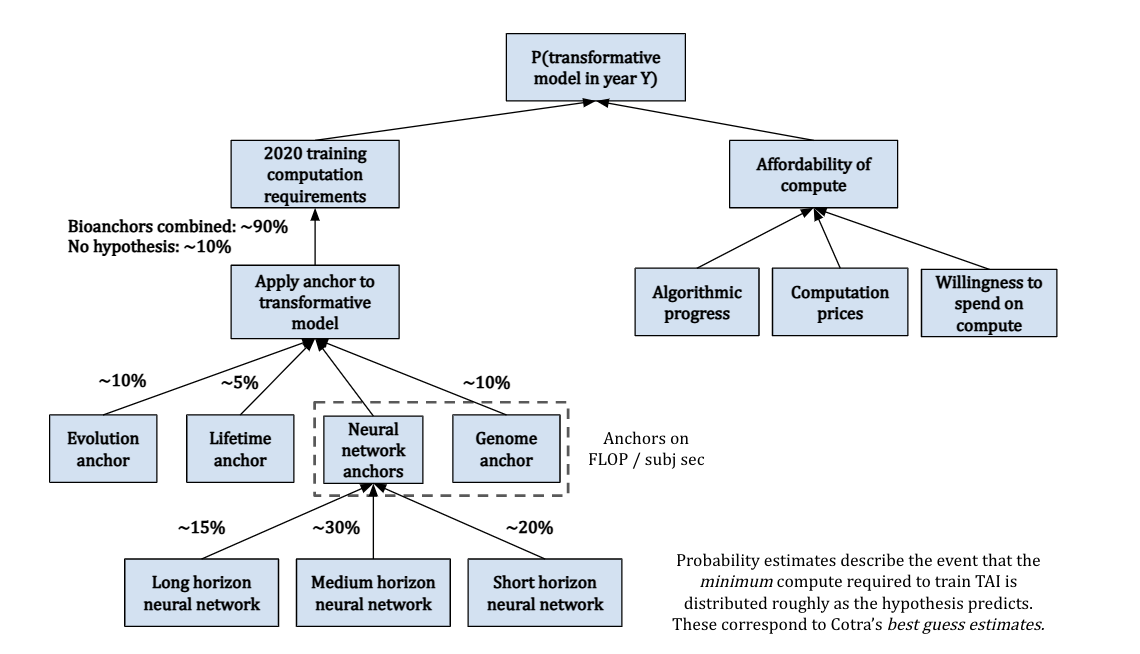
You can see more of our work on our blog. Here is a selection of further work by Epoch members:
![]() | ![]()
|
![]()
|
![]() |
Hiring
We expect to be hiring for several full-time research and management roles this summer. Salaries range from $60,000 for entry roles to $80,000 for senior roles.
If you think you might be a good fit for us, please apply! If you’re unsure whether this is the right role for you, we strongly encourage you to apply anyway. Please register your interest for these roles through our webpage.
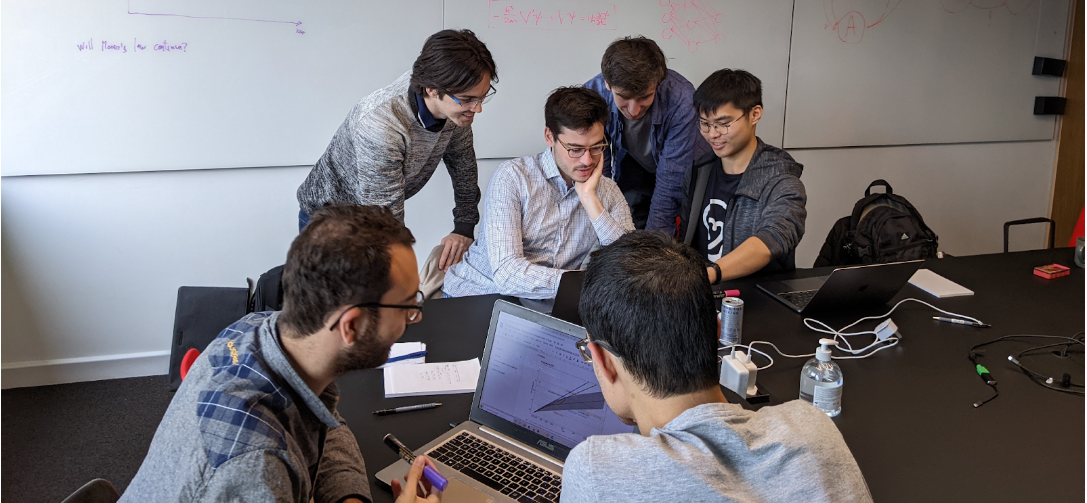
- ^
This blog post previously mentioned MIT; however, despite collaborating with individual researchers from that institution, we are not permitted to use the name of MIT to imply close collaboration or partnership with the institution.
Congrats guys – it's great to see this productive collaboration become a full-fledged organization!
Hey there!
Can you describe your meta process for deciding what analyses to work on and how to communicate them? Analyses about the future development of transformative AI can be extremely beneficial (including via publishing them and getting many people more informed). But getting many people more hyped about scaling up ML models, for example, can also be counterproductive. Notably, The Economist article that you linked to shows your work under the title "The blessings of scale". (I'm not making here a claim that that particular article is net-negative; just that the meta process above is very important.)
OBJECT LEVEL REPLY:
Our current publication policy is:
We think this policy has a good mix of being flexible and giving space for Epoch staff to raise concerns.
Out of curiosity, when you "announce intention to publish a paper or blogpost," how often has a staff member objected in the past, and how often has that led to major changes or not publishing?
I recall three in depth conversations about particular Epoch products. None of them led to a substantive change in publication and content.
OTOH I can think of at least three instances where we decided to not pursue projects or we edited some information out of an article guided by considerations like "we may not want to call attention about this topic".
In general I think we are good at preempting when something might be controversial or could be presented in a less conspicuous framing, and acting on it.
Cool, that's what I expected; I was just surprised by your focus in the above comment on intervening after something had already been written and on the intervention being don't publish rather than edit.
Why'd you strong-downvote?
That´s a good point - I expect most of these discussions to lead to edits rather than publications.
I downvoted because 1) I want to discourage more conversation on the topic and 2) I think its bad policy to ask organizations if they have any projects they decided to keep secret (because if its true they might have to lie about it)
In hindsight I think I am overthinking this, and I retracted my downvotes on this thread of comments.
META LEVEL REPLY
Thinking about the ways publications can be harmful is something that I wish was practiced more widely in the world, specially in the field of AI.
That being said, I believe that in EA, and in particular in AI Safety, the pendulum has swung too far - we would benefit from discussing these issues more openly.
In particular, I think that talking about AI scaling is unlikely to goad major companies to invest much more in AI (there are already huge incentives). And I think EAs and people otherwise invested in AI Safety would benefit from having access to the current best guesses of the people who spend more time thinking about the topic.
This does not exempt the responsibility for Epoch and other people working on AI Strategy to be mindful of how their work could result in harm, but I felt it was important to argue for more openness in the margin.
Very excited about your research agenda, and also how your research will feed into Rethink Priorities' AIGS work.
(I think Rethink's output in a couple other departments has been outstanding, and although some regression to the mean is to be expected I'm still predicting their AIGS work will be very net positive.)
Thanks! We’re very excited to be both an accelerant and a partner for Epoch’s work