This table explores the ethical expected-value of a career in AI safety, under different opinions about AI and the long-term future. I made it for considering the robustness of the value of my possible career choice to different ways my opinions could change, but it may be useful to others also considering a career in AI safety, or for convincing people more skeptical of fast AI timelines that safety work is still important.
My main finding is that you need to hold a pretty specific combination of confident beliefs for AI safety work not to seem tremendously valuable in expected impact, and I personally find those beliefs pretty untenable. I also made a sheet where you can put in your own numbers, to see the implications of your own opinion.
Read the “Explanation” section below if you’re confused about anything.
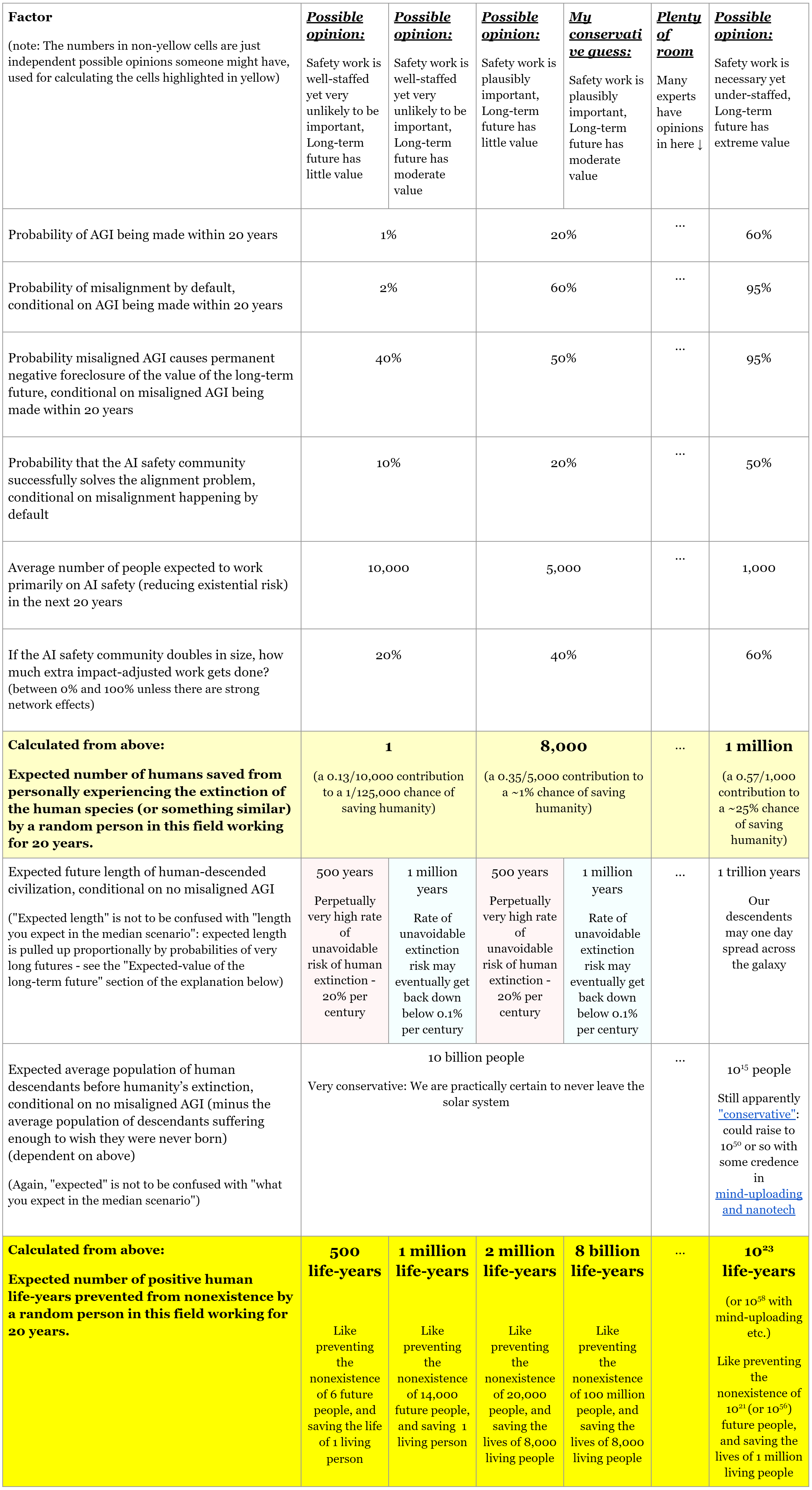
Main takeaway:
You need to hold a pretty specific combination of confident beliefs for AI safety work not to seem tremendously valuable in expected impact.
i.e. something like those in the leftmost column: safety work is likely to be well-staffed, yet very unlikely to be useful, and humanity will go extinct soon with very high probability anyway. I personally find those beliefs pretty untenable - especially the amount of certainty they require about AGI and the long-term future of humanity.
For the AI skeptical: This doesn't mean we're all going to die! - Just that this work seems like a robustly good opportunity because the stakes are so high relative to the chances of having a positive impact, even if we're very likely to be fine anyway.
For the non-skeptical: Reasonable people certainly disagree whether the median scenario looks anything like "we're fine", and I'm very sympathetic to that too. It's just that for answering the binary question of "should more people be working on this?", the median scenario is surprisingly unimportant right now. I'm showing that even people with heavy skepticism and very slow AI timelines should agree that more AI safety work is important, so long as they have some reasonable uncertainties in their views.
Alternate refutations of the importance of AI safety work
(and my responses):
- You could believe that the future of humanity is net negative and humans should be wiped out.
→ But would a world ruled by some sort of paperclips-style AGI be better than humanity under your moral framework? Surely it would be much worse for the environment, and all other life on earth. In any case, the advantage of having humans around for a while longer is that we have more time to figure out what would be morally best for the universe, then do it. Or, better yet, program an actually aligned AGI to do it. Personally I'd prefer we be replaced by wildlife, or a universe of little happy things, rather than a misaligned AGI turning the planet into paperclips or something. See also: suffering risks which might be caused by a misaligned AGI, or the possibility that we might be able to abolish all suffering of humans and all other animals in the future.
- You might think the long-term future is unpredictable and virtually impossible to influence intentionally like this.
→ Sure, the long-term impact of pretty much all our actions has tremendous sign uncertainty, meaning long-term considerations usually wash-out compared to short-term ones, but in this case, are you really so sure? Extinction seems like a pretty clear-cut case in terms of the direction of long-term expected value, relatively speaking. Still, it's possible that there will be a strong enough flow of negative (unforeseen) consequences to outweigh the positives. We should take these seriously, and try to make them less unforseen so we can correct for them, or at least have more accurate expected-value estimates. But given what's at stake, they would need to be pretty darn negative to pull down the expected values enough to outweigh a non-trivial risk of extinction.
- You could very strongly believe a different view of population ethics, or very strongly reject anything like consequentialism, giving less than a 0.01% credence to any moral theory which values the mere existence of happy beings in the future. A ~0.01% credence would let you sit in the second column, but still reject AI safety work.
→ But 0.01% credence is pretty damn sure. Are you sure you’re so sure? See moral uncertainty for a discussion of how you should act when you’re uncertain about the correct moral framework. Regardless, safety is still worth working on if you fall in any except the first two columns, even if you certainly don’t care about the existence or nonexistence of future beings.
- You don’t think we’re under any obligation to do what seems morally best.
→ Perhaps, sure, and probably no one should make you feel guilty for not devoting your career to this. But this is a great opportunity to have a very large positive impact.
- You might react negatively to things which feel like Pascal’s mugging.
→ See Robert Miles' video for a response to this criticism of AI safety work. Basically, it’s worth reasoning about expected values based on evidence.
- You think that most of the important work is done by a small fraction of the AI alignment researchers, so you will personally be unlikely to have an impact as strong as the table suggests.
→ This is true, but before you go into the field you may have no information about where in the efficacy spectrum of AI researchers you will fall. Rather than assuming you will fall near the median, it makes sense to assume you will be like a random person drawn from the distribution of AI safety researchers, in which case your expected contribution is the same as the average contribution, not the median. This is why this table is for a “random” person in the field, rather than the median person (who would have smaller expected impact). Upon entering the field (or just on reviewing your own personal fit) you may receive sufficiently strong indications that you will not be able to be a part of the most efficacious fraction of AI safety researchers. After reassessing the expected value of your impact, it may make sense to switch careers, especially if the field ever becomes primarily funding-constrained and you believe new hires may have a bigger expected impact than you. However, this issue of replaceability reducing your counterfactual impact is much less of a worry in AI alignment than most other fields, and less of a worry in general than you might expect. There seems to be a lot of room to grow the whole field of AI safety right now, so it’s far from a zero-sum game.
- You think there are higher expected-value considerations pushing in the other direction, or plenty of other highly positive careers a potential AI safety researcher could go into instead, and perhaps their expected-value is just harder to quantify.
→ Fair enough, that’s a matter of evidence. I’m sure there are plenty of other careers out there with huge positive moral impacts. The more we can identify the better.
- Your priors are just very low, perhaps due to anthropic reasoning that this can’t be the most important century, or that such a proliferation of future generations can't exist.
→ You are entitled to your priors, but you ought to be very careful with anthropic reasoning. Using it to lower your priors this much seems almost as bad as using it to support something like the doomsday argument. Personally, I’m quite a fan of “fully non-indexical” anthropic reasoning, where you must “condition on all evidence - not just on the fact that you are an intelligent observer, or that you are human, but on the fact that you are a human with a specific set of memories”, in which case I think anthropic reasoning tells you very little about this issue. (podcast for clarification)
Explanation
AI (Artificial Intelligence) safety research seems to have tremendous expected moral value. Most of this expected value probably does not come from the median scenario, so the work will probably look less impactful in hindsight, but this should be no argument against it, as the potential impacts are just so huge, and their probabilities seem plausibly nontrivial. Additionally, there is a range of possibly tractable and useful research areas, widening further as AI capabilities increase.
My credence in (strong) AGI soon:
My definition of strong AGI (Artificial General Intelligence): A system which can (or which can be finetuned to) accomplish most current economically useful objectives more effectively than a very effective person in that field. This includes reasoning about the world (and the humans in it), and making predictions.
- Within 10 years: ~10%, Within 20 years: ~20%, Within 50 years: ~50%
- I’m very uncertain of these, and I hold them very weakly. They’re largely based on priors. Ideally, there would be much more solid research informing all of these credences, but I basically just have to go with what I think for now. For alternative numbers (mostly faster), see:
- Experts' Predictions about the Future of AI
- Draft report on AI timelines by Ajeya Cotra
- Google's "Pathways" plans to immediately start implementing more AGI-like architectures
- DeepMind's stated aim is to "solve intelligence, developing more general and capable problem-solving systems, known as artificial general intelligence (AGI)."
- OpenAI's mission statement: "We will attempt to directly build safe and beneficial AGI ... "
- Metaculus aggregated predictions:
Why have you looked at mostly slower timelines here when most of the sources you link have faster timelines?
- For my selfish personal use, I wanted to see just how far my opinions would have to change before a career in AI safety would no longer seem extremely beneficial. Then, seeing that they would have to move a tremendous amount, I can very confidently commit to AI safety without worrying about reconsidering my career choice in light of new evidence every day. (Just every few years or something)
- The people with fast timelines are probably more likely to buy into the usefulness of AI safety work already (I think?), so showing that even most slow-timeline scenarios have great expected value is useful for convincing more mainstream people about the value of AI safety work.
Based on (hurdles still in front of AGI):
- Long-term planning (abstraction from immediate actions to long-term plans and back)
- Internal model of the world which can be updated at runtime to resolve inconsistencies
- Sufficient training compute and data, or increased compute & data efficiency
These are not independent; progress on one is likely to be useful to the others, and more data & compute alone could concievably be sufficient for all. On the other hand, I’m sure I’m missing some.
AGI development paths / warning signs (conditional on AGI):
- Breakthrough out of the blue: 15%
- One team over the course of a few years (some clearish signs, things getting very strange): 25%
- Multiple teams over the course of a few years (multiple clearish signs, things getting very strange): 40%
- Anything slower / more multipolar: 20%
- The West ahead in development: 70%
- Slow takeoff speed once developed (AGI has most of its potential for existential risk before any self-improving "singularity"): 60%
Again, I hold these credences very weakly. Substitute your own.
Impacts of AGI:
- Implications are massively underestimated by most people (for strong AGI)
- It’s such a pivotal technology that it seems impossible to picture how the world will look in 100 years without knowing how (or whether) AGI goes
Misalignment risk:
Various actors (eg. companies looking for profit) would benefit from making AI systems which behave like “agents” pursuing some external goals in the world. When machine-learning systems are trained, however, their learned internal “goals” (to the extent that they have them) are merely selected to produce good behaivour on the training data, and so their internal goals often differ importantly from the external goals on which they were trained, which can lead to them competently pursuing an unintended goal.
As an analogy, evolution selected us to “maximize inclusive reproductive fitness” creating a jumble of values and goals which led to behaivour looking a lot like “maximizing inclusive reproductive fitness” in our past evolutionary environment. However our internalized goals are quite different to “maximize inclusive reproductive fitness”. This misalignment is apparent now that many things (eg. contraceptives, abundant sugar, technology, global society) have taken us out of evolution’s “training environment”. See mesa-optimization and inner-alignment (video).
Additionally, we cannot currently infer a model's internal goals (or if it has any) by looking inside it, so we are currently only able to infer discrepancies through behaviour. As a result, detecting when a system is an "agent" or is optimizing for some goal is difficult, and goal-pursuing agents might emerge for instrumental reasons in cases where we are not explicity selecting the AI to behave like a goal-pursuing agent.
AIs could have just about any internal goal, and just about any goal is compatible with ssuperhuman capabilities. A “misaligned” AI would be any AI with an internal goal not fully aligned with our values, with the discrepancy eventually being actualized in some important way. See the orthogonality thesis (video).
An AI with coherent intrinisic goals is incentivized to do whatever it can to raise the probability of achieving them. Some ways that a misaligned AI could fail to have its goals achieved: Humans shutting it down; Humans changing its goals; Humans discovering that it is misaligned before it is in a position where humans are no longer able to change it. See instrumental convergence (video)
Finally, even if the internal goal of the AI matches the external goal on which it was trained, it seems hard to find goals which are both well-specified and aligned with human values when pursued by AIs with exremely high capabilities.
- Misalignment risk seems greatest during wartime (but probably not dominated by wartime risk).
- Outside of wartime, surely no one would be stupid enough to let a paperclips-style single-optimizer go wild. Right? Still, significant probability that one may be developed, maybe just because it’s easier to train an AI to optimize for one thing, like it’s easier to train GPT to optimize next-word performance.
- To me, the most likely scenario is a subtler, mesa-alignment type problem. There will be warning signs in stupider iterations being deceptive or competently optimizing for the wrong thing, but perhaps the applied fix turns out to be more of a bandaid than anticipated, until too late.
- What might the first AGIs look like? Optimizing actors (hopefully not)? Feedback responding actors? Queryable world models? Risk depends on that.
- Overall, hard to say (especially taking into account existing trajectory of AI safety work), but significant probability of subtle misalignment, which might not be fixable until too late.
- Again, the largest potential for counterfactual impact may not necessarily come from scenarios like the median (although in this case it might).
Existential risk:
Definition of existential risk
- Misaligned AGI seems like the most plausible path to permanently cutting off humanity’s potential (See “The Precipice” for a comparison with other risks).
- Forgoing trillions of descendant-years of potential flourishing throughout the galaxy would probably be very bad.
- A sufficiently cognitively powerful system will be able to outwit humanity at every turn, and do whatever it wants.
- Any interaction with humans is enough of a channel to allow a superintelligence to manipulate them, escape, and do whatever it wants.
- If it determined humanity to be a threat to its goals, a superintelligence could, for example, remotely trick, blackmail, or manipulate someone in a biolab to unknowingly synthesize a virus which might wipe out humanity. (or not - superintelligence could probably come up with something much more effective).
The main way we might make a misaligned powerful AI and still be lucky enough to not all die is if it happens to be incompetent enough in some relevant area far outside its training set that we can leverage that area to outwit and stop it. In other words, If the AI has low alignment robustness, but also low capability robustness, we might be ok. Additionally, see Counterarguments to the basic AI risk case for many more ways we might be ok.
Opportunities for intervention:
There are many important, neglected, tractable (enough) research topics to pursue:
- Interpretability / Transparency
- Being able to give useful answers to "Why did the AI do that?" (eg. ARC)
- Determining how AIs work mechanistically, so we can predict how their behavior will generalize to new situations (eg. Neel Nanda or Redwood Research)
- Extracting how AIs represent concepts and knowledge about their environment (eg. Eliciting latent knowledge or ROME)
- Determining whether an AI is "agentic"
- Extracting how AIs represent their goals
- AI "mind reading" and deception detection
- Understanding how concepts, abstractions, heuristic values, goals, and/or agency are formed through training, so that one can predict ahead of time how AIs trained in various ways will generalize to new situations (eg. Shard Theory or John Wentworth)
- Increasing alignment-robustness to distributional shifts, with an extremely low tolerance for error (training to avoid Goal Misgeneralization)
- Looking for trends of potentially worrying behaviors in current AIs, to determine how risky the next generations might be (eg. Beth Barnes)
- Inverse reinforcement learning / value learning
- Corrigibility (making systems which won't resist being shut down)
- Myopia (making systems which don't have potentially dangerous goals)
- Theoretical work on what a safe AGI could look like
- Many more (I’m not an expert, but this is proof of concept for some tractable areas):
Expected-value of the long-term future:
This is only upper-bounded by the laws of physics and the time until all the stars die out. The lower bound depends on your optimism for the human species. The potential is vast - If they aren’t extinct, far future beings in a post-scarcity society will have had the time to figure out how to be much happier, more fulfilled, more numerous, and more ethical, (or to change their biologies to achieve this) spreading more of whatever is good throughout the galaxy, or preserving or restoring it if it was already there.
A note on expected values: Unless you give a vanishingly small probability to futures where we expand through the universe, or develop mind-uploading technology, these should influence your expected values for the number of beings in the future, pulling your expected numbers up far above the median scenario. And if you think the probability is vanishingly small, it still has to vanish as fast as the potential population increases - getting down to less than about 1 in 1050 once you start talking about scenarios with virtual-mind carrying machines expanding through the universe.
Put differently, most of the people who will ever live in the future possible histories of the universe will probably live in fairly low-probability scenarios, made up for by their extreme proliferation in that low-probability scenario (unless you think extreme proliferation is the median scenario, or extreme proliferation is even more extremely unlikely). This is somewhat akin to where we find ourselves today, as viewed from the distant (geologic-time) past.
Let me know if you have any feedback.
Thanks for this exercise, it's great to do this kind of thinking explicitly and get other eyes on it.
One issue that jumps out at me to adjust: the calculation of researcher impact doesn't seem to be marginal impact. You give a 10% chance of the alignment research community averting disaster conditional on misalignment by default in the scenarios where safety work is plausibly important, then divide that by the expected number of people in the field to get a per-researcher impact. But in expectation you should expect marginal impact to be less than average impact: the chance the alignment community averts disaster with 500 people seems like a lot more than half the chance it would do so with 1000 people.
I would distribute my credence in alignment research making the difference over a number of doublings of the cumulative quality-adjusted efforts, e.g. say that you get an x% reduction of risk per doubling over some range.
Although in that framework if you would likely have doom with zero effort, that means we have more probability of making the difference to distribute across the effort levels above zero. The results could be pretty similar but a bit smaller than yours above if we thought that the marginal doubling of cumulative effort was worth a 5-10% relative risk reduction.
This is a good point I hadn't considered. I've added a few rows calculating a marginal correction-factor to the google sheet and I'll update the table if you think they're sensible.
The new correction factor is based on integrating an exponentially decaying function from N_researchers to N_researchers+1, with the decay rate set by a question about the effect of halving the size of the AI alignment community. Make sure to expand the hidden rows in the doc if you want to see the calculations.
Caveats: No one likes me. I don't know anything about AI safety, and I have trouble reading spreadsheets. I use paperclips sometimes to make sculptures.
Ok, this statement about marginal effects is internally consistent....but this seems more than a little nitpicky?
It would be great for my comment here is to be wrong and be stomped all over!
Also, if there is a more substantial reason this post can be expanded, that seems useful.
Please don't ban me.
I didn't read it actually.
Like, Chris Olah might be brilliant and 100x better than every other AI safety person/approach. At the same time, we could easily imagine that, no matter what, he's not going to get AI safety by himself, but an entire org like Anthropic might, right?
As an example, one activist doesn't seem to think any current AI safety intervention is effective at all.
In that person's worldview/opinion, applying a log production function doesn't seem right. It's unlikely that say, 7 doublings would do it (100x more quality adjusted people) in this rigid function, since the base probability is so low.
In reality, I think that in that person's worldview, certain configurations of 100x more talent would be effective.
One issue here with some of the latter numbers is that a lot of the work is being done by the expected value of the far future being very high, and (to a lesser extent) by us living in the hinge of history.
Among the set of potential longtermist projects to work on (e.g. AI alignment, vs. technical biosecurity, or EA community building, or longtermist grantmaking, or AI policy, or macrostrategy), I don't think the present analysis of very high ethical value (in absolute terms) should be dispositive in causing someone to choose careers in AI alignment.
Yes, that is true. I'm sure those other careers are also tremendously valuable. Frankly I have no idea if they're more or less valuable than direct AI safety work. I wasn't making any attempt to compare them (though doing so would be useful). My main counterfactual was a regular career in academia or something, and I chose to look at AI safety because I think I might have good personal fit and I saw opportunities to get into that area.
Thanks, this makes sense!
I do appreciate you (and others) thinking clearly about this, and your interest in safeguarding the future.
This seems like one of the best written summaries of AI safety.
The OP is a great writer, covers a lot of considerations, and is succinct and well organized.
People at any of the AI safety orgs should probably reach out to this person, so they can do AI safety stuff.
(Holden Karnofsky’s posts are good too).
This is a touchingly earnest comment. Also is your ldap qiurui? If those words mean nothing to you, I've got the wrong guy :)
(I cancelled the vote on my comment so it doesn't appear in the "newsfeed", this is because it's sort of like a PM and of low interest to others.)
No, I don't know what that means. But, yes I'm earnest about my comment and thanks for the appreciation.
Thanks! Means a lot :)
(I promise this is not my alt account flattering myself)
I'll be attending MLAB2 in Berkeley this August so hopefully I'll meet some people there.
Hey there!
The word "unexpected" sort of makes that sentence trivially true. If we remove it, I'm not sure the sentence is true. [EDIT: while writing this I misinterpreted the sentence as: "AI safety research seems unlikely to end up causing more harm than good"] Some of the things to consider (written quickly, plausibly contains errors, not a complete list):
And here's the CEO of Conjecture (59:50) [EDIT: this is from 2020, probably before Conjecture was created]:
Also, low quality research or poor discussion can make it less likely that important decision makers will take AI safety seriously.
Important point. I changed
to
I added an EDIT block in the first paragraph after quoting you (I've misinterpreted your sentence).
Nice! I really like this analysis, particularly the opportunity to see how many present-day lives would be saved in expectation. I mostly agree with it, but two small disagreements:
First, I’d say that there are already more than 100 people working directly on AI safety, making that an unreasonable lower bound for the number of people working on it over the next 20 years. This would include most of the staff of Anthropic, Redwood, MIRI, Cohere, and CHAI; many people at OpenAI, Deepmind, CSET, and FHI; and various individuals at Berkeley, NYU, Cornell, Harvard, MIT, and elsewhere. There’s also tons of funding and field-building going on right now which should increase future contributions. This is a perennial question that deserves a more detailed analysis than this comment, but here’s some sources that might be useful:
https://forum.effectivealtruism.org/posts/8ErtxW7FRPGMtDqJy/the-academic-contribution-to-ai-safety-seems-large
Ben Todd would guess it’s about 100 people, so maybe my estimate was wrong: https://twitter.com/ben_j_todd/status/1489985966714544134?s=21&t=Swy2p2vMZmUSi3HaGDFFAQ
Second, I strongly believe that most of the impact in AI safety will come from a handful of the most impactful individuals. Moreover I think it’s reasonable to make guesses about where you’ll fall in that distribution. For example, somebody with a history of published research who can get into a top PhD program has a much higher expected impact than somebody who doesn’t have strong career capital to leverage for AI safety. The question of whether you could become one of the most successful people in your field might be the most important component of personal fit and could plausibly dominate considerations of scale and neglectedness in an impact analysis.
For more analysis of the heavy-tailed nature of academic success, see: https://forum.effectivealtruism.org/posts/PFxmd5bf7nqGNLYCg/a-bird-s-eye-view-of-the-ml-field-pragmatic-ai-safety-2
But great post, thanks for sharing!
Yeah your first point is probably true, 100 may be unreasonable even as a lower bound (in the rightmost column). I should change it.
--
Following your second point, I changed:
to