TLDR: Jargon often worsens the communication of EA ideas and makes it harder for us to update our models of the world. I think EAs should apply strategies to notice/reduce jargon, and I offer a few examples of jargon-reducing techniques.
A few weeks ago, I attended EA Global and a retreat about community building. At both events, we discussed EA-related ideas and how to most effectively communicate them.
One idea I’ve been reflecting on: It can be tempting to use jargon when explaining EA ideas, even in contexts in which jargon is not helpful.
In this post, I describe some drawbacks of EA jargon & offer some suggestions for EAs who want to reduce their use of jargon. (Note: Some of these ideas are inspired by Robert Wilbin's talk and forum post about EA jargon. I have tried to avoid covering the same points he raises, but I strongly suggest checking out those resources.)
What is jargon?
Jargon is “special words or expressions that are used by a particular profession or group and are difficult for others to understand.” It can refer to any terms/phrases that are used in shorthand to communicate broader ideas. Examples include: “Epistemic humility,” “Shapley values,” “Population ethics,” “The INT framework,” and “longtermism.”
Why should we reduce jargon?
- Spreading knowledge more efficiently. Reducing jargon will increase the rate at which ideas are spread (especially to people who are not already in the EA community). Logic: Less Jargon → Clearer messages → more people understand them → faster rate of information transfer → more EAs!
- Spreading knowledge more accurately (and reducing low-fidelity messaging). Jargon can make it more likely for people to misinterpret EA ideas. Misinterpretations are generally bad, and they can be really bad if influential communicators (e.g., journalists) disseminate misinterpretations of EA concepts to large numbers of people.
These two benefits have focused largely on how others react to jargon. The next two focus on how jargon may directly benefit the person who is communicating:
- Improving our own reasoning. In some cases, jargon can improve precision. In others, jargon makes ideas less precise. Consider claims like: “Factory farming is a neglected cause area” or “AI safety is important if you believe in longtermism.” These claims would be clearer—and the speaker would be forced to think more deeply about their position—if we removed the italicized word and replaced it with a more specific and precise belief (see this post for more examples of imprecise/misused jargon).
- Making it easier for others to prove us wrong. I have found that others disagree with me more frequently when I deliberately avoid jargon. There are probably two main reasons why.
- First, people are often reluctant to admit that they don’t know something. There’s a strong temptation to simply nod along and agree, especially for new members of a group, even if they’re deeply confused.
- Second, jargon can make it seem like people agree, even though they actually disagree. This is because jargon is ambiguous and can often be interpreted in many ways. Consider the statement, “Factory farming is a neglected cause area.” The speaker could mean any of the following:
- Outside of the EA community, there are not enough people or organizations focused on fighting factory farming.
- Within the EA community, there are not many people or organizations focused on fighting factory farming.
- There is not enough money devoted to fighting factory farming.
- There are not enough people fighting factory farming.
- There are not enough individuals with a specific set of skills fighting factory farming.
- Notice that two people could agree with the broad claim “factory farming is a neglected cause area” despite having rather different understandings of what “neglected” means and rather different actual beliefs. Jargon makes it easier for people to assume that they agree (because on some high-level, they do) and makes it harder for people to identify the specific areas in which they disagree. This is especially likely given that people often interpret ambiguous information in ways that match their own beliefs.
- This is harmful because we want people to disagree with us, so that we can update our beliefs about the world. When truth-seeking is a primary objective, reducing jargon will make (productive) disagreements easier to spot.
- Note: Sometimes, jargon increases the precision of our claims and therefore leads to more opportunities for productive disagreement. If you are confident that the people you are talking to a) know the jargon, b) interpret the jargon in the same way as you do, and c) are comfortable revealing when they are confused, then the expected value of jargon increases. I think these cases are the exception rather than the rule.
How can we reduce jargon?
- Establish and reinforce norms around asking for clarification. I think the EA community generally does a good job with this one. Examples: Promoting a culture of remaining open to disagreement, not reacting with hostility or shock if someone doesn’t know something, and taking a “growth mindset” lens.
- For instance, in Penn EA meetings, I sometimes try to ask questions like “In this context, what exactly do you mean when you say longtermism?” or “Before we dive in further, can you provide a quick explanation of population ethics?” In my experience, this rarely wastes much time, and it often makes other people more comfortable asking questions as the conversation gets deeper.
- Ask people to call out whenever you’re using jargon.
- Immediate feedback is especially helpful.
- Model behaviors that reduce or clarify jargon (especially if you are an organizer, leader, or someone who others look up to)
- Create “jargon glossaries”
- A “jargon glossary” involves two steps. First, brainstorm terms that are commonly used in EA. Then, define them.
- This can also be a group activity:
- 1) Prepare a list of terms
- 2) Spend 5-10 minutes writing down definitions individually
- 3) Discuss as a group. Pay attention to ways in which members disagree, or have different perspectives/lenses, on what certain terms mean.
- For inspiration, see this slide from Robert Wilbin’s presentation.
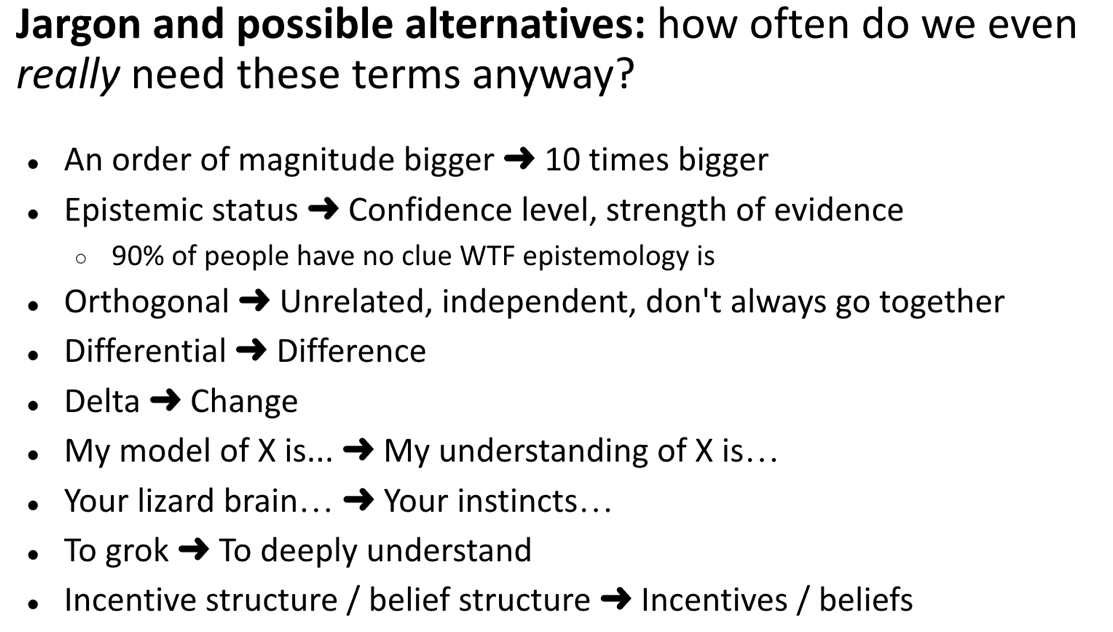
Conclusion
Optimizing metacommunication techniques in EA ideas is difficult, especially when trying to communicate highly nuanced ideas while maintaining high-fidelity communication and strong epistemics.
In other words: Communicating about EA is hard. We discuss complicated ideas, and we want them to be discussed clearly and rigorously.
To do this better, I suggest that we proactively notice and challenge jargon.
This is my current model of the world, but it could very well be wrong. I welcome disagreements and feedback in the comments!
I’m grateful to Aaron Gertler, Chana Messinger, Jack Goldberg, and Liam Alexander for feedback on this post.
I suspect people overestimate the harm of jargon for hypothetical "other people" and underestimate the value. In particular, polls I've run on social media have historically gotten results where people have consistently expressed a preference for more jargon rather than for less jargon.
Now, of course, these results are biased by the audience I have, rather than my "target audience," who may have different jargon preferences than the people who bother to listen to me on social media.
But if anything, I think my own target audience is more familiar with EA jargon, rather than less, compared to my actual audience.
I think my points are less true for people in an outreach-focused position, like organizers of university groups.
Jargon glossaries sound like a great idea! (I'd be very excited to see them integrated with the wiki.)
A post I quite like on the topic of jargon: 3 suggestions about jargon in EA. The tl;dr is that jargon is relatively often misused, that it's great to explain or hyperlink a particular piece of jargon the first time it's used in a post/piece of writing (if it's being used), and that we should avoid incorrectly implying that things originated in EA.
(I especially like the second point; I love hyperlinks and appreciate it when people give me a term to Google.)
Also, you linked Rob Wiblin's presentation (thank you!)-- the corresponding post has a bunch of comments.
This is an idea I've considered and I'd be interested in making it happen if I continue working on the Wiki. If anyone has suggestions, feel free to leave them below or contact me privately.
Like Lizka said, glossaries seem to be a great idea!
Drawing on the posts and projects for software here, here, here, and here, there seems to be a concrete, accessible software project for creating a glossary procedurally.
(Somewhat technical stuff below, I wrote this quickly and it's sort of long.)
Sketch of project
You can programmatically create an EA Jargon glossary that can complement, not replace a human glossary. It can continuously refresh itself, capturing new words as time passes.
This is writing a Python script or module that finds EA forum words and associates it with definitions.
To be concrete, here is one sketch of how to how to build this:
Because the core work is essentially word counting and the later steps can be very sophisticated, this project would be accessible to people newer in NLP, and also interest more advanced practitioners.
By the way, this seems like this totally could get funded with an infrastructure grant. Maybe if you wanted go in this direction, optionally:
Maybe there's reasons to get an EA infrastructure grant to do this:
Anyways, apologies for being long. I just sometimes get excited and like to write about ideas like this. Feel free to ignore me and just do it!