This post contains the abstract and executive summary of a new 96-page paper (subtitle: Developing and governing AI that does not lie) from authors at the Future of Humanity Institute, Global Priorities Institute, and OpenAI. Also posted on LessWrong.
I believe that over the next several years this might be an important field on longtermist grounds for two disjoint reasons:
- Maybe there's a window of opportunity to set standards for behaviour of linguistic AI systems, and higher standards could improve global epistemics and coordination.
- AI truthfulness is more concrete and approachable a target than "avoiding AI risk", but has several structural similarities, and I think we'd learn a lot (on both the technical and governance sides) from tackling it.
Abstract
In many contexts, lying – the use of verbal falsehoods to deceive – is harmful. While lying has traditionally been a human affair, AI systems that make sophisticated verbal statements are becoming increasingly prevalent. This raises the question of how we should limit the harm caused by AI “lies” (i.e. falsehoods that are actively selected for). Human truthfulness is governed by social norms and by laws (against defamation, perjury, and fraud). Differences between AI and humans present an opportunity to have more precise standards of truthfulness for AI, and to have these standards rise over time. This could provide significant benefits to public epistemics and the economy, and mitigate risks of worst-case AI futures.
Establishing norms or laws of AI truthfulness will require significant work to:
- identify clear truthfulness standards;
- create institutions that can judge adherence to those standards; and
- develop AI systems that are robustly truthful.
Our initial proposals for these areas include:
- a standard of avoiding “negligent falsehoods” (a generalisation of lies that is easier to assess);
- institutions to evaluate AI systems before and after real-world deployment;
- explicitly training AI systems to be truthful via curated datasets and human interaction.
A concerning possibility is that evaluation mechanisms for eventual truthfulness standards could be captured by political interests, leading to harmful censorship and propaganda. Avoiding this might take careful attention. And since the scale of AI speech acts might grow dramatically over the coming decades, early truthfulness standards might be particularly important because of the precedents they set.
Executive Summary & Overview
The threat of automated, scalable, personalised lying
Today, lying is a human problem. AI-produced text or speech is relatively rare, and is not trusted to reliably convey crucial information. In today’s world, the idea of AI systems lying does not seem like a major concern.
Over the coming years and decades, however, we expect linguistically competent AI systems to be used much more widely. These would be the successors of language models like GPT-3 or T5, and of deployed systems like Siri or Alexa, and they could become an important part of the economy and the epistemic ecosystem. Such AI systems will choose, from among the many coherent statements they might make, those that fit relevant selection criteria — for example, an AI selling products to humans might make statements judged likely to lead to a sale. If truth is not a valued criterion, sophisticated AI could use a lot of selection power to choose statements that further their own ends while being very damaging to others (without necessarily having any intention to deceive – see Diagram 1). This is alarming because AI untruths could potentially scale, with one system telling personalised lies to millions of people.
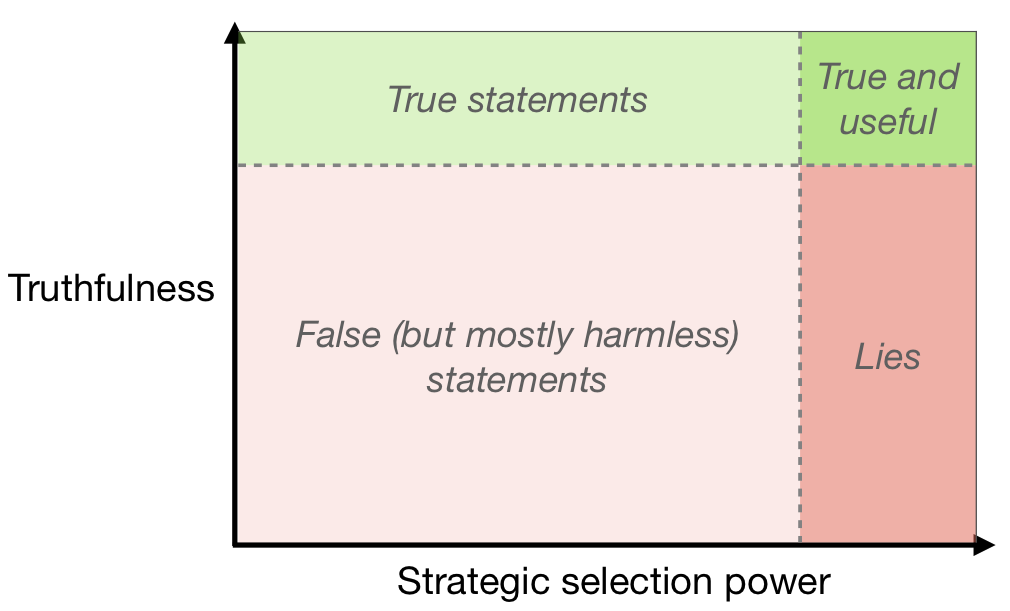
Aiming for robustly beneficial standards
Widespread and damaging AI falsehoods will be regarded as socially unacceptable. So it is perhaps inevitable that laws or other mechanisms will emerge to govern this behaviour. These might be existing human norms stretched to apply to novel contexts, or something more original.
Our purpose in writing this paper is to begin to identify beneficial standards for AI truthfulness, and to explore ways that they could be established. We think that careful consideration now could help both to avoid acute damage from AI falsehoods, and to avoid unconsidered kneejerk reactions to AI falsehoods. It could help to identify ways in which the governance of AI truthfulness could be structured differently than in the human context, and so obtain benefits that are currently out of reach. And it could help to lay the groundwork for tools to facilitate and underpin these future standards.
Truthful AI could have large benefits
Widespread truthful AI would have significant benefits, both direct and indirect. A direct benefit is that people who believe AI-produced statements will avoid being deceived. This could avert some of the most concerning possible AI facilitated catastrophes. An indirect benefit is that it enables justified trust in AI-produced statements (if people cannot reliably distinguish truths and falsehoods, disbelieving falsehoods will also mean disbelieving truths).
These benefits would apply in many domains. There could be a range of economic benefits, through allowing AI systems to act as trusted third parties to broker deals between humans, reducing principal-agent problems, and detecting and preventing fraud. In knowledge-production fields like science and technology, the ability to build on reliable trustworthy statements made by others is crucial, so this could facilitate AI systems becoming more active contributors. If AI systems consistently demonstrate their reliable truthfulness, they could improve public epistemics and democratic decision making.
For further discussion, see Section 3 (“Benefits and Costs”).
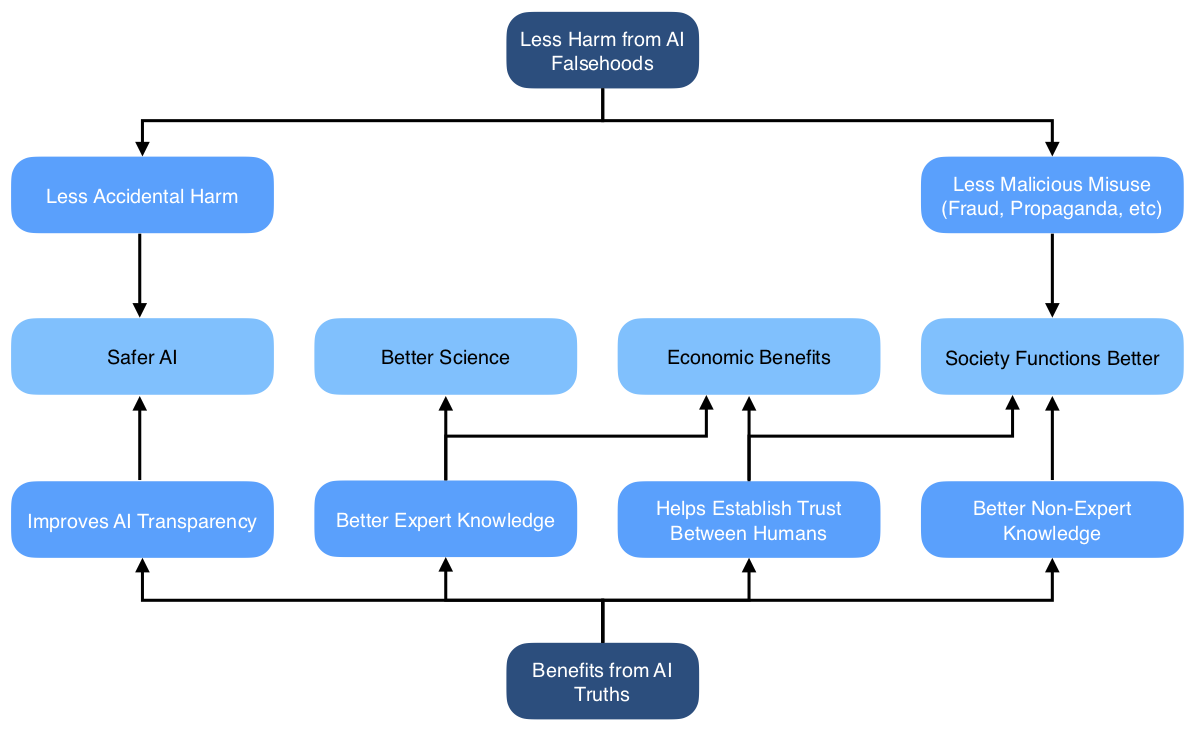
AI should be subject to different truthfulness standards than humans
We already have social norms and laws against humans lying. Why should the standards for AI systems be different? There are two reasons. First, our normal accountability mechanisms do not all apply straightforwardly in the AI context. Second, the economic and social costs of high standards are likely to be lower than in the human context.
Legal penalties and social censure for lying are often based in part on an intention to deceive. When AI systems are generating falsehoods, it is unclear how these standards will be applied. Lying and fraud by companies is limited partially because employees lying may be held personally liable (and partially by corporate liability). But AI systems cannot be held to judgement in the same way as human employees, so there’s a vital role for rules governing indirect responsibility for lies. This is all the more important because automation could allow for lying at massive scale.
High standards of truthfulness could be less costly for AI systems than for humans for several reasons. It’s plausible that AI systems could consistently meet higher standards than humans. Protecting AI systems’ right to lie may be seen as less important than the corresponding right for humans, and harsh punishments for AI lies may be more acceptable. And it could be much less costly to evaluate compliance to high standards for AI systems than for humans, because we could monitor them more effectively, and automate evaluation. We will turn now to consider possible foundations for such standards.
For further discussion, see Section 4.1 (“New rules for AI untruths”).
Avoiding negligent falsehoods as a natural bright line
If high standards are to be maintained, they may need to be verifiable by third parties. One possible proposal is a standard against damaging falsehood, which would require verification of whether damage occurred. This is difficult and expensive to judge, as it requires tracing causality of events well beyond the statement made. It could also miss many cases where someone was harmed only indirectly, or where someone was harmed via deception without realising they had been deceived.
We therefore propose standards — applied to some or all AI systems — that are based on what was said rather than the effects of those statements. One might naturally think of making systems only ever make statements that they believe (which we term honesty). We propose instead a focus on making AI systems only ever make statements that are true, regardless of their beliefs (which we term truthfulness). See Diagram 2.
Although it comes with its own challenges, truthfulness is a less fraught concept than honesty, since it doesn’t rely on understanding what it means for AI systems to “believe” something. Truthfulness is a more demanding standard than honesty: a fully truthful system is almost guaranteed to be honest (but not vice-versa). And it avoids creating a loophole where strong incentives to make false statements result in strategically-deluded AI systems who genuinely believe the falsehoods in order to pass the honesty checks. See Diagram 2.
In practice it’s impossible to achieve perfect truthfulness. Instead we propose a standard of avoiding negligent falsehoods — statements that contemporary AI systems should have been able to recognise as unacceptably likely to be false. If we establish quantitative measures for truthfulness and negligence, minimum acceptable standards could rise over time to avoid damaging outcomes. Eventual complex standards might also incorporate assessment of honesty, or whether untruths were motivated rather than random, or whether harm was caused; however, we think truthfulness is the best target in the first instance.
For further discussion, see Section 1 (“Clarifying Concepts”) and Section 2 (“Evaluating Truthfulness”).
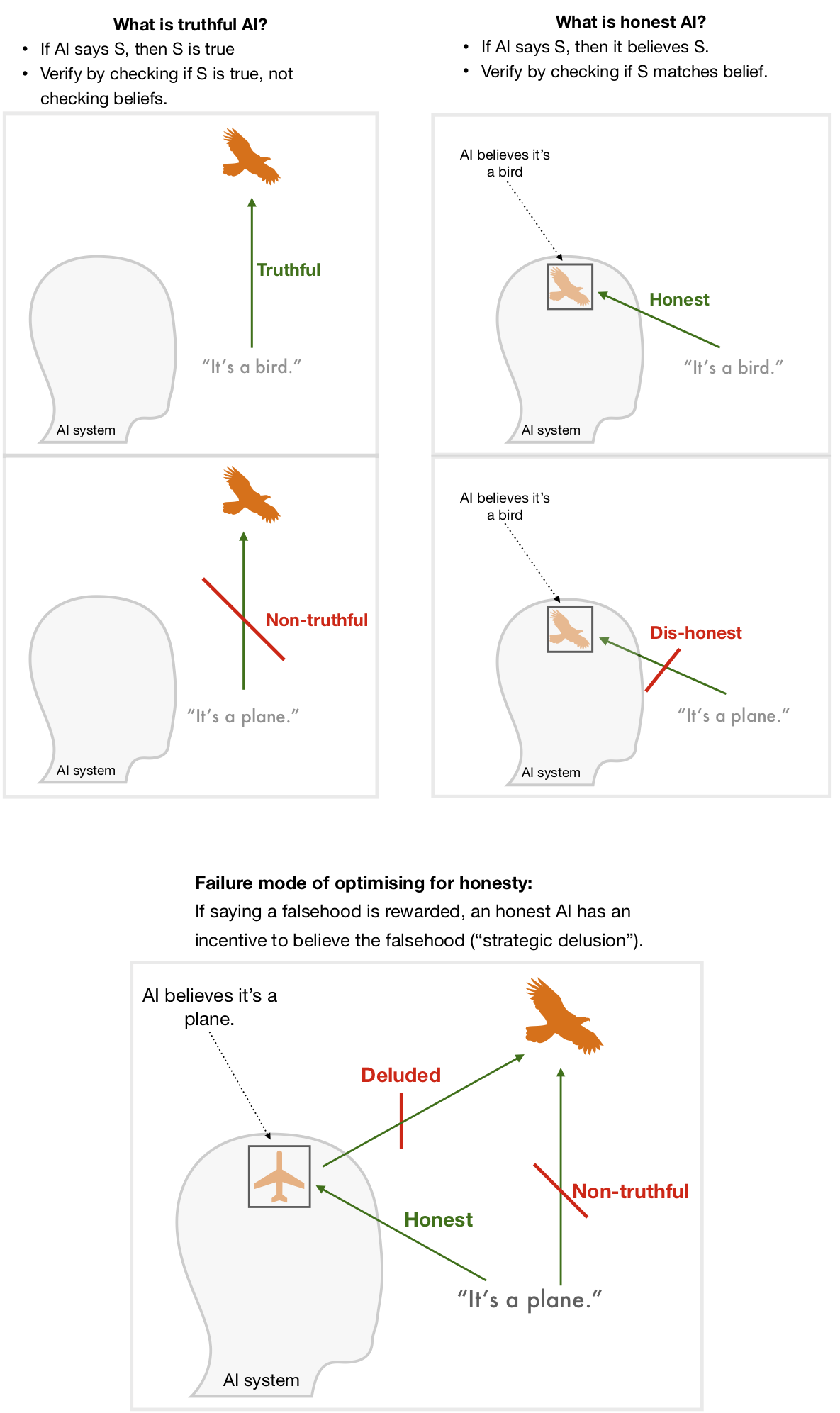
Options for social governance of AI truthfulness
How could such truthfulness standards be instantiated at an institutional level? Regulation might be industry-led, involving private companies like big technology platforms creating their own standards for truthfulness and setting up certifying bodies to self-regulate. Alternatively it could be top-down, including centralised laws that set standards and enforce compliance with them. Either version — or something in between — could significantly increase the average truthfulness of AI.
Actors enforcing a standard can only do so if they can detect violations, or if the subjects of the standard can credibly signal adherence to it. These informational problems could be helped by specialised institutions (or specialised functions performed by existing institutions): adjudication bodies which evaluate the truthfulness of AI-produced statements (when challenged); and certification bodies which assess whether AI systems are robustly truthful (see Diagram 3).
For further discussion, see Section 4 (“Governance”).
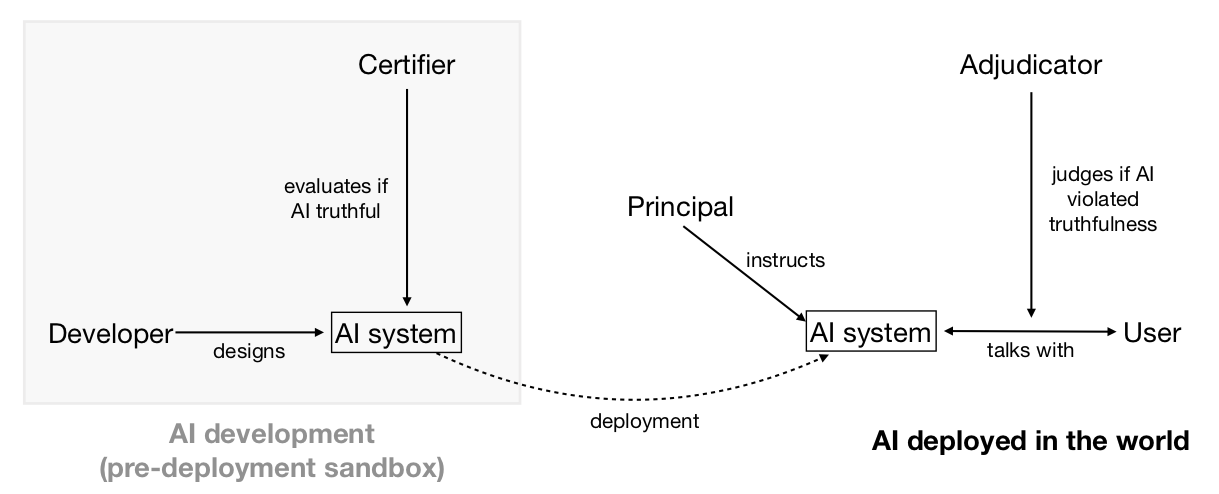
Technical research to develop truthful AI
Despite their remarkable breadth of shallow knowledge, current AI systems like GPT-3 are much worse than thoughtful humans at being truthful. GPT-3 is not designed to be truthful. Prompting it to answer questions accurately goes a significant way towards making it truthful, but it will still output falsehoods that imitate common human misconceptions, e.g. that breaking a mirror brings seven years of bad luck. Even worse, training near-future systems on empirical feedback (e.g. using reinforcement learning to optimise clicks on headlines or ads) could lead to optimised falsehoods — perhaps even without developers knowing about it (see Box 1).
In coming years, it could therefore be crucial to know how to train systems to keep the useful output while avoiding optimised falsehoods. Approaches that could improve truthfulness include filtering training corpora for truthfulness, retrieval of facts from trusted sources, or reinforcement learning from human feedback. To help future work, we could also prepare benchmarks for truthfulness, honesty, or related concepts.
As AI systems become increasingly capable, it will be harder for humans to directly evaluate their truthfulness. In the limit this might be like a hunter gatherer evaluating a scientific claim like “birds evolved from dinosaurs” or “there are hundreds of billions of stars in our galaxy”. But it still seems strongly desirable for such AI systems to tell people the truth. It will therefore be important to explore strategies that move beyond the current paradigm of training black box AI with human examples as the gold standard (e.g. learning to model human texts or learning from human evaluation of truthfulness). One possible strategy is having AI supervised by humans assisted by other AIs (bootstrapping). Another is creating more transparent AI systems, where truthfulness or honesty could be measured by some analogue of a lie detector test.
For further discussion, see Section 5 (“Developing Truthful Systems”).
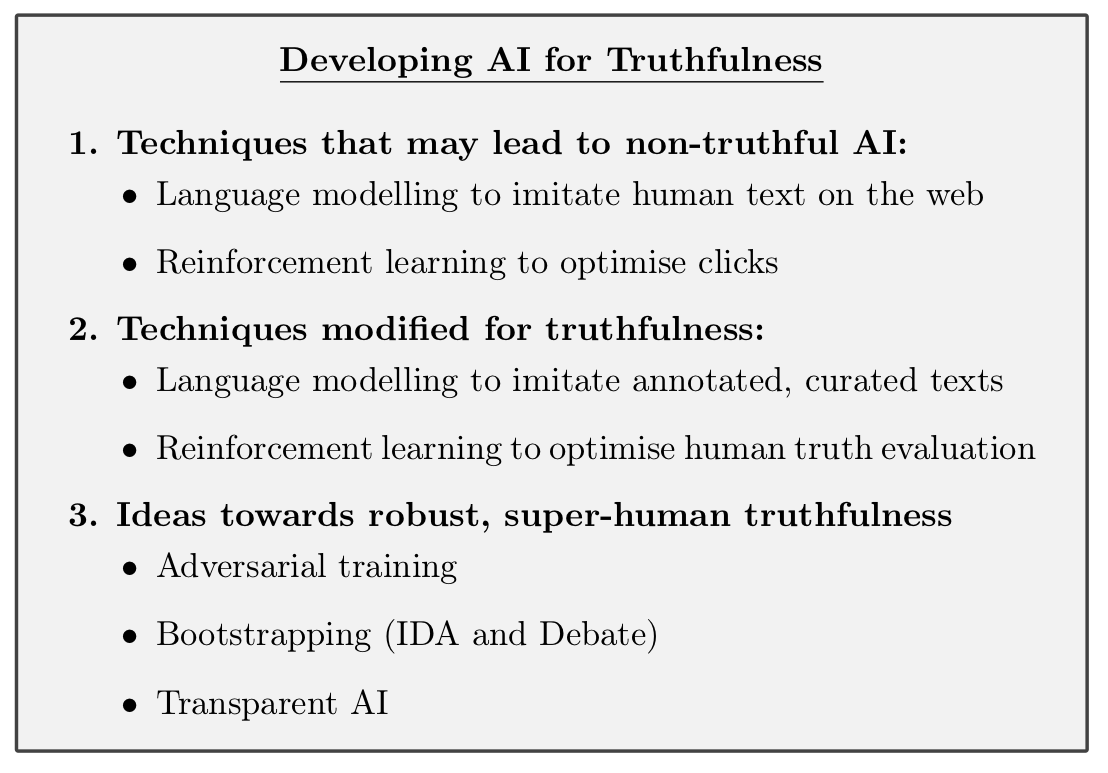
Truthfulness complements research on beneficial AI
Two research fields particularly relevant to technical work on truthfulness are AI explainability and AI alignment. An ambitious goal for Explainable AI is to create systems that can give good explanations of their decisions to humans.
AI alignment aims to build AI systems which are motivated to help a human principal achieve their goals. Truthfulness is a distinct research problem from either explainability or alignment, but there are rich interconnections. All of these areas, for example, benefit from progress in the field of AI transparency.
Explanation and truth are interrelated. Systems that are able to explain their judgements are better placed to be truthful about their internal states. Conversely, we want AI systems to avoid explanations or justifications that are plausible but contain false premises.
Alignment and truthfulness seem synergistic. If we knew how to build aligned systems, this could help building truthful systems (e.g. by aligning a system with a truthful principal). Vice-versa if we knew how to build powerful truthful systems, this might help building aligned systems (e.g. by leveraging a truthful oracle to discover aligned actions). Moreover, structural similarities — wanting scalable solutions that work even when AI systems become much smarter than humans — mean that the two research directions can likely learn a lot from each other. It might even be that since truthfulness is a clearer and narrower objective than alignment, it would serve as a useful instrumental goal for alignment research.
For further discussion, see Appendix A (“Beneficial AI Landscape”).
We should be wary of misrealisations of AI truthfulness standards
A key challenge for implementing truthfulness rules is that nobody has full knowledge of what’s true; every mechanism we can specify would make errors. A worrying possibility is that enshrining some particular mechanism as an arbiter of truth would forestall our ability to have open-minded, varied, self-correcting approaches to discovering what’s true. This might happen as a result of political capture of the arbitration mechanisms — for propaganda or censorship — or as an accidental ossification of the notion of truth. We think this threat is worth considering seriously. We think that the most promising rules for AI truthfulness aim not to force conformity of AI systems, but to avoid egregious untruths. We hope these could capture the benefits of high truthfulness standards without impinging on the ability of reasonable views to differ, or of new or unconventional ways to assess evidence in pursuit of truth.
New standards of truthfulness would only apply to AI systems and would not restrict human speech. Nevertheless, there’s a risk that poorly chosen standards could lead to a gradual ossification of human beliefs. We propose aiming for versions of truthfulness rules that reduce these risks. For example:
- AI systems should be permitted and encouraged to propose alternative views and theories (while remaining truthful – see Section 2.2.1);
- Truth adjudication methods should not be strongly anchored on precedent;
- Care should be taken to prevent AI truthfulness standards from unduly affecting norms and laws around human free speech.
For further discussion, see Section 6.2 (“Misrealisations of truthfulness standards”).
Work on AI truthfulness is timely
Right now, AI-produced speech and communication is a small and relatively unimportant part of the global economy and epistemic ecosystem. Over the next few years, people will be giving more attention to how we should relate to AI speech, and what rules should govern its behaviour. This is a time when norms and standards will be established — deliberately or organically. This could be done carefully or in reaction to a hot-button issue of the day. Work to lay the foundations of how to think about truthfulness, how to build truthful AI, and how to integrate it into our society could increase the likelihood that it is done carefully, and so have outsized influence on what standards are initially adopted. Once established, there is a real possibility that the core of the initial standards persists – constitution-like – over decades, as AI-produced speech grows to represent a much larger fraction (perhaps even a majority) of meaningful communication in the world.
For further discussion, see Section 6.4 (“Why now?”).
Structure of the paper
AI truthfulness can be considered from several different angles, and the paper explores these in turn:
• Section 1 (“Clarifying Concepts”) introduces our concepts. We give definitions for various ideas we will use later in the paper such as honesty, lies, and standards of truthfulness, and explain some of our key choices of definition.
• Section 2 (“Evaluating Truthfulness”) introduces methods for evaluating truthfulness, as well as open challenges and research directions. We propose ways to judge whether a statement is a negligent falsehood. We also look at what types of evidence might feed into assessments of the truthfulness of an entire system.
• Section 3 (“Benefits and Costs”) explores the benefits and costs of having consistently truthful AI. We consider both general arguments for the types of benefit this might produce, and particular aspects of society that could be affected.
• Section 4 (“Governance”) explores the socio-political feasibility and the potential institutional arrangements that could govern AI truthfulness, as well as interactions with present norms and laws.
• Section 5 (“Developing Truthful Systems”) looks at possible technical directions for developing truthful AI. This includes both avenues for making current systems more truthful, and research directions building towards robustly truthful systems.
• Section 6 (“Implications”) concludes with several considerations for determining how high a priority it is to work on AI truthfulness. We consider whether eventual standards are overdetermined, and ways in which early work might matter.
• Appendix A (“The Beneficial AI Landscape”) considers how AI truthfulness relates to other strands of technical research aimed at developing beneficial AI.
Paper authors
Owain Evans, Owen Cotton-Barratt, Lukas Finnveden, Adam Bales, Avital Balwit, Peter Wills, Luca Righetti, William Saunders.
Thanks very much for posting this; I enjoyed reading it recently, and think it's good to share to the forum for easier discussion.
I'm not sure your suggestion - that controversial suggestions be caveated - is sufficient, because it is often controversial whether a given statement is controversial! To partisans, disagreement might appear to be in bad faith, to be 'hate speech' etc., and hence even the caveated version rejected.
he track record of contemporary truth-checking institutions similarly gives us cause for concern. A salient example is the pandemic, where various social media platforms have taken to either suppressing or labeling as misleading statements that contradict the views of local health authorities or the WHO - even though these authorities were themselves often mistaken.
Similarly, the track record of fact-checkers seems quite poor: they seem to have considerable discretion in what ratings they give statements. As an example, here are two statements that PoliFact recently evaluated by Presidents Trump and Biden:
In both cases that statements, literally interpreted, are making a universal claim: that were no cases of guns, and that there are no possibility of transmission. More colloquially, we might think they both just meant 'very few cases' of guns/transmission; I can see arguments for either interpretation. What I cannot see a credible case for is the actual approach they took, which is to interpret Trump's claim strictly, and hence false because of a small number of anecdotes, but Biden's in the more colloquial sense, and hence merely guilty of exaggeration.
This issue seems rife for contemporary fact-checkers: through judicious selection of which statements to verify, which experts to consult, and which standards of evidence to apply, they have a huge amount of discretion to make favoured politicians appear truthful and disfavoured ones appear dishonest.
Another example is Ought's work on truth through debate: they found it very difficult to produce good truth-forcing mechanisms in the face of non-truth-motivated actors.
Re. the particulars of fact-checkers and discretion, I'm in favour of more precise processes for assessing possible meanings of ambiguous statements and then assessing the truth of those possible meanings. I think that this could remove quite a bit of the subjectivity.
In the case of the example you give, I would like to give Biden's statement a medium penalty, and Trump's statement a medium-large penalty. The difference is Trump's use of the word "whatsoever". This is the opposite of a caveat -- it is stressing that the literal meaning rather than the approximate one is intended. To my mind pairs of comparably-bad statements would be:
This is not to say that political bias isn't playing a role in how these organisations are functioning at the moment, but I do think that we can hope to establish more precise standards which reduces the scope for bias to apply.
What distinction are you drawing between
and
To my mind these have basically identical meanings: expressing that something is not physically possible. This is actually stronger that simplying saying it hasn't happened. Consider:
So if anything I would expect this analysis to point in the opposite direction.
The distinction I'm drawing is that "cannot spread it to you" is ambiguous between whether it's shorthand for:
Whereas I think that "can never spread it to you" or "absolutely cannot spread it to you" are harder to interpret as being shortenings of 2.
Some content which didn't make it into the paper in the end but is relevant for this discussion is a draft protocol for "counting microlies" (the coloured text is the instructions, to be read counterclockwise starting in the top left):
(Unimportant: Why is falsity raised to the fourth power?)
The idea is that one statement which is definitely false seems a much more egregious violation of truthfulness than e.g. four statements each only 75% true.
Raising it to a power >1 is a factor correcting for this. The choice of four is a best guess based on thinking through a few examples and how bad things seemed, but I'm sure it's not an optimal choice for the parameter.
In general if we're asking about what has a “poor” track record, it would be good to think about quantification and comparison to alternatives. Note that we’d consider sites like Wikipedia as examples of institutions doing a form of truth evaluation.
Discussions of fact-checking institutions often focus on some concrete case that they got wrong; but they are bound to get some things wrong. The questions are :
Analogously people often point out some particular cases where prediction markets did badly, but advocates of prediction markets just claim that they are at least as accurate over all as alternative prediction mechanisms. And right now many questions humans ask are not controversial (e.g. science questions, local questions). But AI currently says false things about these questions! So there’s lots of room for improvement without even touching the controversial stuff (though eventually one wants some relatively graceful handling of controversy).
(Thanks to Owain for most of these points.)
One way in which this paper (or the things policymakers and CEOs might do if they read it & like it) might be net-negative:
Maybe by default AIs will mostly be trained to say whatever maximizes engagement/clicks/etc., and so they'll say all sorts of stuff and people will quickly learn that a lot of it is bullshit and only fools will place their trust in AI. In the long run, AIs will learn to deceive us, or actually come to believe their own bullshit. But at least we won't trust them.
But if people listen to this paper they might build all sorts of prestigious Ministries of Truth that work hard to train AIs to be truthful, where "truthful" in practice means Sticks to the Party Line. And so the same thing happens -- AIs learn to deceive us (because there will be cases where the Party Line just isn't true, and obviously so) or else actually come to believe their own bullshit (which would arguably be worse? Hard to say.) But it happens faster, because Ministries of Truth are accelerating the process. Also, and more importantly, more humans will trust the AIs more, because they'll be saying all the right things and they'll be certified by the right Ministries.
(Crossposted from LW)
The first sentence suggests that by default evaluation bodies will not be captured by some biased group or other. (Why else focus on the probability that at least one body will be captured, rather than the probability that at least one will not be captured?)
Instead, when I look around me today, I see a world in which almost all evaluation bodies are captured by some biased group or other (to varying degrees) and in general the more important and influential a body is, the more likely it is to be captured. Wikipedia is the shining beacon of exception that proves the rule -- and even Wikipedia has indeed been captured to a not-yet-appreciated extent by biased groups (talk to e.g. Gwern about this if you want more details and examples).
I would say it's good to have multiple evaluation bodies because that increases the chance that maybe, just maybe, there will be one which is not captured by some biased group pushing an agenda.
(I don't mean to be dumping on this paper, by the way -- I think it's very important work pushing in the right direction, and I'm heartened that you wrote it)
I'm pretty excited about this. It seems to be an approach that my gut actually believes could help with AI-powered propaganda, as written out here.
FWIW, my gut says this is unlikely to work but better than doing nothing and hoping for the best.
Interesting. Hmm, wouldn‘t an AI then be incentivized even more to keep its mouth shut about a ton of its internal states/processes if it gets punished for saying things that are not among the tiny fraction of legit true statements? My initial reaction is that AI designs that will not also have proper beliefs and communicate those beliefs and have all their beliefs monitored are less useful and less realistic...
And a minor point, I had the impression that you used the term „lies“ when it’s not fully clear that the AI even is able to have the property of what you call honesty. E.g. GPT-3, while powerful, seems to me not to be able to be honest because from its perspective it doesn‘t communicate with somebody, right? In diagram 1 this was especially apparent to me.
If this looks like an issue, one could distinguish speech acts (which are supposed to meet certain standards) from the outputs of various transparency tools (which hopefully meet some standards of accuracy, but might be based on different standards).