In a recent answer to Issa’s Why "cause area" as the unit of analysis?, Michael Plant presents his take on cause prioritization and points to his thesis. As part of my cause prioritization analysis work with QURI I read the relevant parts of his thesis and found them interesting and novel, so I want to bring more attention to it.
In his Ph.D. thesis, Michael Plant (the founder of Happier Lives Institute) reviews the foundations of EA, presents constructive criticism on the importance of saving lives, sheds more light on how we can effectively make more people happier, describes weaknesses in the current approaches to cause prioritization and suggests a practical refinement - "Cause Mapping". In this post, I summarize the key points of chapters 5 and 6 about cause prioritization of Michael's thesis.
Main points
- Cause areas can be thought of as "problems", while interventions can be thought of as corresponding "solutions".
- Cause Prioritization is an attempt to implicitly compare between the best achievable interventions in each cause area, without knowing in advance what are the most promising interventions.
- We should be wary of dismissing cause areas too early - it's easy to miss out on the best interventions and we might be discouraged from rechecking them at a later time.
- "Cause Mapping", a systematized method for cause prioritization, partially addresses these problems by presenting a more exhaustive approach with a greater potential to figure out what further information is needed. Broadly, it is done by mapping out potential intervention areas and the possible obstacles to applying them.
Points 1,2,3 are discussed in the next section and point 4 is discussed in the section about chapter 6. Both of these sections are my attempt to succinctly introduce Michael's work in the corresponding chapters, without my personal thoughts. I present some of my personal takeaways in the final section.
Chapter 5 - Cause Prioritization
Michael starts chapter 5 by explaining how people in EA tend to use "cause prioritization" in practice and how that can be defined -
Cause prioritisation: comparing the marginal cost-effectiveness of different problems. This is done using the three-factor framework, i.e. assessing causes by their scale, neglectedness and solvability
Cause prioritization is followed by "intervention prioritization", where quantitative cost-effectiveness estimates are used to compare interventions for promising causes. Generally, "causes" and "interventions" are just different words for "problems" and "solutions".
Cause Promisingness is Cost-Effectiveness of Best Intervention
Michael notes that the question of "How promising is a given cause area X rather than a cause area Y?" is actually "How cost-effective are the best available interventions for addressing X rather than Y?". This is natural - all we actually care about (from a consequentialist point of view) is how actions we can take translate into real-world value. This, then, raises the problem of how can we in fact separate cause prioritization from intervention prioritization
[...] it’s not immediately clear how we can determine which causes are higher priority prior to considering, at least implicitly, the interventions we might use in each case and how cost-effective those interventions are. [...] The explanation seems to be that we can evaluate problems as a whole if and when we can say something about the cost-effectiveness of all the solutions to a given problem.
Scale, Neglectedness and Solvability as Negative Indicators
One way to use the scale-neglectedness-solvability framework is to spot cases where the cause area clearly doesn't seem promising. Michael writes these explicitly -
- Small Scale: If the problem is so tiny and it's clear that putting any effort toward solving it would be a waste.
- Low Neglectedness: If the problem will be solved by others regardless of what we do, and our actions have no (or tiny) counterfactual impact.
- Low Solvability: If the problem is not solvable by any means.
Overall
In total, this analysis suggests that we should conceptualize our approach to prioritization as having three steps:
- ‘Screen out’ problems where it’s clear all their solutions are cost-ineffective. This is done by appealing to one or more of scale, neglectedness, and solvability individually.
- Make an intuitive cost-effectiveness evaluation of the most promising solution(s) to each problem. This is done by combining scale, neglectedness and solvability.
- Make explicit, quantitative cost-effectiveness evaluations of particular solutions to problems.
Issues with this approach
Despite the reasonableness of this approach, there are two potentially important problems with this framework that Michael raises:
- First, this method requires us to be aware of existing solutions, which we might easily miss.
- Second, if we have thrown out a cause area because we overlooked something, the method discourages us to look again.
This leads Michael to suggest a more exhaustive approach.
Chapter 6 - Cause Mapping
Michael suggests a systematic method for generating additional potentially promising causes, which helps to refine our understanding of our original causes and relations between them. Broadly, this is done by listing the types of actions one can take and their potential obstacles per each problem we care about solving, and then see how these obstacles can be addressed (and by doing that we have a new cause area).
Let's break this down, using Michael's definitions and his examples from the perspective of increasing well-being (of people alive today). In the thesis work, this method had actually helped Michael discover potential avenues for further research and serves as a basis for further work in happiness research.
- List primary causes. We start by listing the main problems which we want to solve. These are problems that we care directly about solving, rather than solving as means to solving other problems, and are thus named "primary causes". We screen out primary causes where it's clear that all of their solutions are not cost-effective by appealing to one or more of scale, neglectedness, and solvability individually (as above).
- Focusing on well-being, Michael lists Mundane, sub-optimal happiness; Mental health; Pain; and Poverty.
- List main mechanisms. For each primary cause, we can list the different types of methods that can make progress on them. These basically intend to capture the possible actions we can take and the types of interventions available.
- In the case of happiness, Michael offers an over-arching categorization into six categories that broadly apply for all four primary causes:
- External: altering someone’s objective circumstances, such as wealth, education, physical environment or the society they live in.
- Temporal: how people choose to spend their time.
- Psychological: changing how people think, e.g. cognitive treatments for
- Chemical: using mood-enhancing substances, e.g. alcohol, painkillers or anti mental health. depressants.
- Physical: direct manipulation of the body or brain, e.g. surgical procedures, Deep Brain Stimulation (‘DBS’).
- Biological: genetically modifying people to be happier.
- In the case of happiness, Michael offers an over-arching categorization into six categories that broadly apply for all four primary causes:
- Assess main obstacles in the way of each mechanism. These obstacles might differ in magnitude for different people or different organizations as they have different comparative advantages and disadvantages, so prioritization should depend on the specific situation we are in.
- There are five main types of obstacles presented:
- Research: the mechanism is (theoretically) usable, but more know-how is required.
- Behaviour/motivation: people don’t want to use the mechanism, even though it’s available.
- Education: people would use it, but they don’t know about it.
- Resources: people know about it and would use it but can’t afford it.
- Policy: the state needs to act before the mechanism can be used.
- There are five main types of obstacles presented:
- List solutions. Each combination of a mechanism and an obstacle for it presents us with an opportunity to generate solutions, or particular actions we can take to do good.
- Taking a look at the primary cause of "(unhappiness caused by) poverty". The main way to directly address it is by the "external" mechanism of giving people more resources or by means that they could have bought had they had more money. Here we know quite a bit about the most promising potential interventions. Givewell's recommended charities are one good option, and their main obstacle to do more good is more money ("Resources"). Thus efforts to increase funding going there (say, by donation or fundraising) might be good. Another relevant way to cause change is around institutional change, whose main obstacle is "Policy" - leading us to suggest solutions around international aid reform, say.
- Set out Secondary and Meta Causes. (These will be explained below)
- Evaluate the solutions for cost-effectiveness.
Before we explain the last two steps, take a look at the following table where Michael suggests some solutions in the case of happiness interventions. Rows are the Mechanism, columns are the Primary Causes, and for each solution, its corresponding Obstacles are in parenthesis.
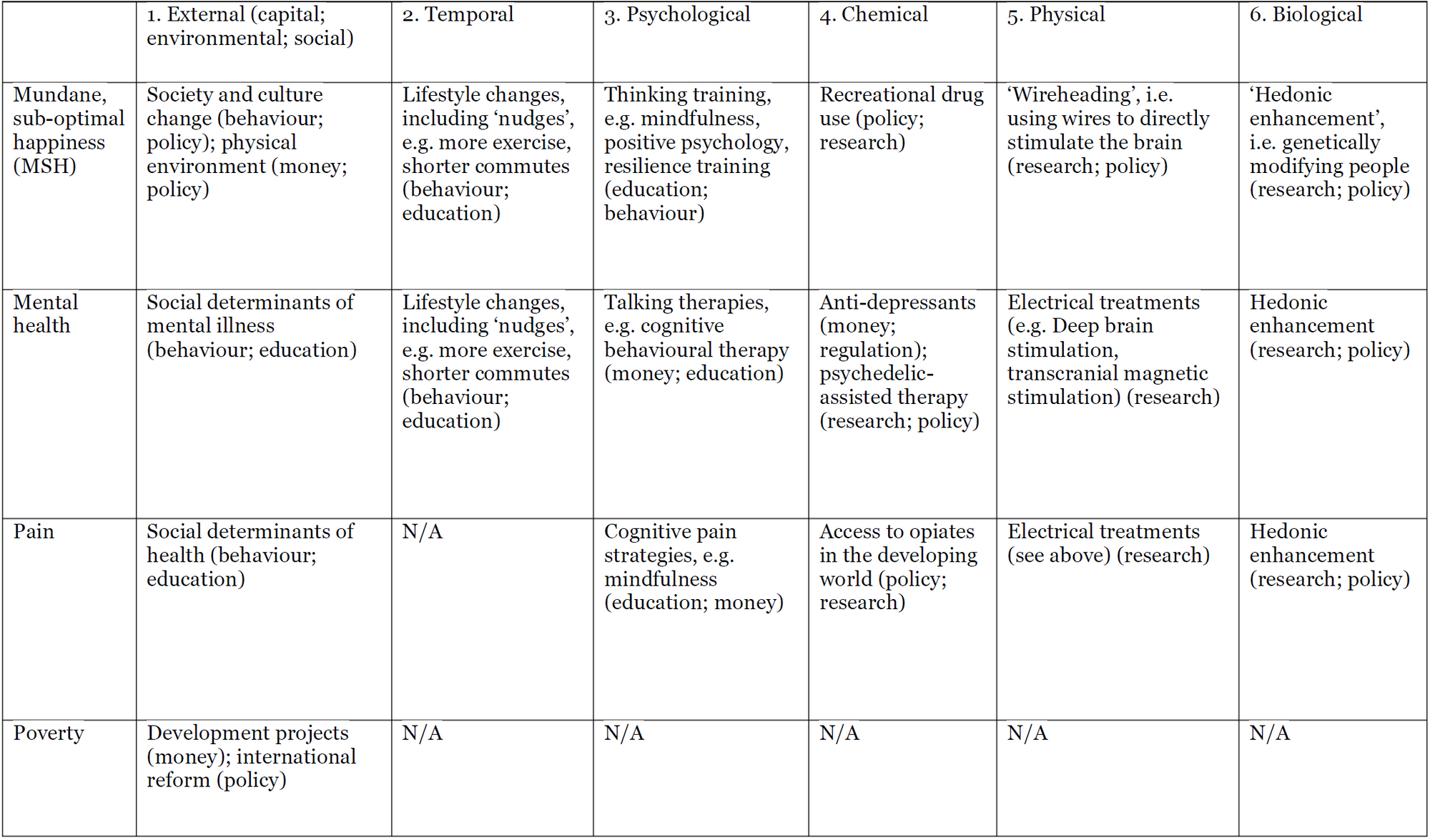
Taking a look at the table, we see that many solutions are applicable to several primary causes. This is reasonable, as the different problems have similar characteristics and obstacles. Therefore, our next stage is to cluster together similar solutions. These solution clusters by themselves can be considered "cause areas" (or possibly "intervention areas"). Michael suggests distinguishing between two types of solution clusters.
Secondary Causes are types of actions or solutions that by themselves partially solve the primary cause. The distinction of a "primary" from a "secondary" cause is by noting that the first is a problem and the latter is a type of intervention. Meta Causes are, in contrast, only instrumentally useful in that they only do good by having beneficial consequences on several (primary or secondary) causes instead of doing good directly.
The following diagram continues the example in the table above, where secondary causes are linked to the relevant obstacles they address (and meta causes has arrows for illustration purposes).
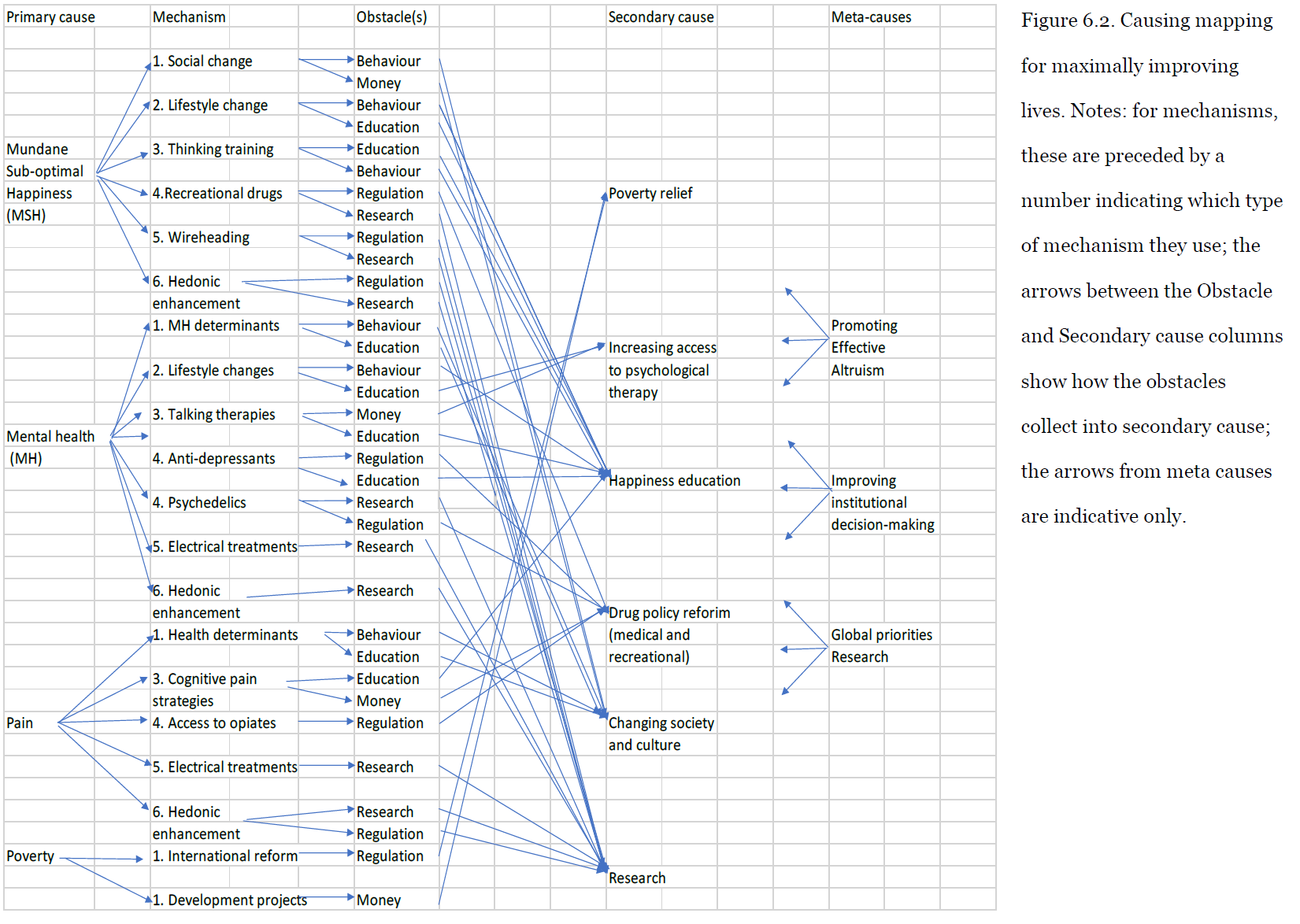
To prioritize meta causes one needs to have a very detailed understanding of all of the other relevant causes. Michael does not say much here.
In regards to secondary causes though, Michael writes the following.
The secondary causes are best understood as a ‘longlist’ of areas for further investigation in the sense that we hope they will contain all the good options, but not all the options they contain are good—by differentiation, we would say a ‘shortlist’ would have only good options.
making progress on evaluating the secondary causes requires doing one or more of three tasks:
- First, running new empirical experiments to test key claims. For instance, running randomised-controlled trials using happiness measures to test the cost-effectiveness of mental health and anti-poverty interventions in the developing world.
- Second, building cost-effectiveness models of interventions where evidence already exists, such as on the effect of positive psychology programmes that teach children to be happier.
- Third, establishing the particular places money could be donated to and comparing the effectiveness of particular organizations in that field.
Conclusion and Personal Thoughts
I, and others, think that there is room for a lot more useful work in cause prioritization. Taking some time to read more about this subject and how people in the community have thought about cause prioritization, there is clearly some confusion around the basic terms and processes involved, and there might be better ways to do cause prioritization which are right around the corner and better means to coordinate around our project of figuring out how to do the most good.
Overall I agree with the main thrust of this work, and I really enjoyed reading this. I especially think that it is important to be wary not to dismiss causes too soon (and give forgotten cause candidates further thought). I also think that when we think about a cause we should (mostly?) keep in mind the potential interventions in that area and map these thoroughly.
I'd be interested in comparing this approach to cause areas to something like GiveWell's definition: “a particular set of problems, or opportunities, such that the people and organizations working on them are likely to interact with each other, and such that evaluating many of these people and organizations requires knowledge of overlapping subjects.”
Another interesting point raised in this text is the process of defining secondary and meta causes. Generally speaking, a lot of causes have very complicated theories of change, and I'm curious to see a general framework that can handle interrelations between causes.
Thanks to Michael Plant for reviewing this post and giving useful feedback.
I found this really interesting and never would have read the full thing (sorry Michael!), so thanks for posting this summary. It was a pleasure to read
Yep, for exactly this reason, I'm glad someone kindly summarised and surfaced these dark corners of my thesis.
Michael Plant made a similar point in the comment you cite at the start of this post. I responded that I didn't think the point was quite right. The fleshed out picture of Michael's reasoning given in this post resolves some of what I said in response (regarding not knowing in advance what the best interventions in each area are). But I think the reasoning given in this post still isn't quite right, because I don't think we only care about the best interventions in each area; I think we also care about other identifiable positive outliers. Reasons for that include the facts that:
And there are probably cases in which we have to or should "invest" in a cause area in a general way, not just invest in one specific intervention. So it's useful to know which cause area will be able to best use a large chunk of a certain type of resources, not just which cause area contains the one intervention that is most cost-effective given generic resources on the current margin.
For example, let's suppose for the sake of discussion that technical AI safety research is the best solution within the x-risk cause area, that deworming is the best solution in the global health & development cause area, and that technical AI safety is better than deworming. (Personally, I believe the third claim, and am more agnostic about the other two, but this is just an example.) In that case, in comparing the cause areas (to inform decisions like what skills EAs should skill up in, what networks we should build, what careers people should pursue, and where money should go), it would still be useful to know what the other frontrunner solutions are, and how they compare across cause areas.
I think this is, to some extent, a reason why GiveWell's definition (mentioned at the end of this post) is useful.
Hmm. It seems like the only way this differs from my account is that 'cause comparisons' are/should be the comparison of the top interventions, rather than just intervention. But the 'cause comparison' is still impossible without (implicitly) evaluating the specific things you can do.
Yes, I think that sounds correct to me. I think that that's what I was trying to get across with "But I think the reasoning given in this post still isn't quite right, because I don't think we only care about the best interventions in each area; I think we also care about other identifiable positive outliers."
I.e., I do think that, other that that point, I agree with your discussion of what the question "How promising is a given cause area X rather than a cause area Y?" should be interpreted and roughly how it should be tackled.
I agree with this. I think one important consideration here is who are the agents for which we are doing the prioritization.
If our goal is to start a new charity and we are comparing causes, then all we should care about is the best intervention (we can find) - the one which we will end up implementing. If, in contrast, our goal is to develop a diverse community of people interested in exploring and solving some cause, we might care about a broader range of interventions, as well as potentially some qualities of the problem which help increase overall cohesiveness between the different actors
I agree with this.
I'm not sure I understand this. Could you expand?
Sure. So, consider x-risk as an example cause area. It is a pretty broad cause area and contains secondary causes like mitigating AI-risk or Biorisk. Developing this as a common cause area involves advances like understanding what are the different risks, identifying relevant political and legal actions, making a strong ethical case, and gathering broad support.
So even if we think that the best interventions are likely in, say, AI-safety, it might be better to develop a community around a broader cause area. (So, here I'm thinking of cause area more like that in Givewell's 2013 definition).
This is a good point that I hadn't thought of.
But I slightly disagree with charity example. The main reason is that the intervention that's in general best may not be the one that's best for whatever audience we're talking to, due to personal fit. (In both cases, "best" should be interpreted as "best in expectation, on the margin, given our current knowledge and time available for searching", but that's irrelevant to the point I want to make.)
This is most obvious if we're planning to ourselves run the charity. It's less obvious if we're doing something more like what Charity Entrepreneurship does, where we'll ultimately seek out people from a large pool, since then we can seek people out partly based on personal fit for our charity idea. But:
A smaller reason why I disagree is that, even if our primary goal is to start a new charity, it may be the case that a non-negligible fraction of the impact of our research comes from other effects (e.g., informing donors, researchers, people deciding on careers unrelated to charity entrepreneurship). This seems to be the case for Charity Entrepreneurship, and analogous things seem to be the case for 80,000 Hours, GiveWell, etc. But this point feels more like a nit-pick.
In any case, as mentioned, I do think that your point is a good one, and I think I only slightly disagree :)
I share this view. I also feel like it might tie in somewhat with discussion related to Cause X, the possibility of an ongoing moral catastrophe, etc.
...that said, I guess it's easy to agree that we shouldn't dismiss something "too soon", and I'm not actually sure whether EA is currently erring towards dismissing things too soon or not quickly enough.
And I guess there's also a way your statement is in tension with the standard discussion of Cause X, the possibility of an ongoing moral catastrophe, etc.; if we spend more resources (including time) re-assessing causes that have been preliminarily dismissed, this leaves us with fewer resources available for identifying and (further) assessing cause candidates that haven't been dismissed yet.
The point about 'dismissing too soon' comes from the realisation that one doesn't really evaluate the cost-effectiveness of resources to causes (entire problems), but you only evaluate solutions. Someone who thought they were able to evaluate causes as a whole, and so hadn't really looked at what you might do, would be liable to discount problems too soon.
This is all fairly abstract, but I suppose I take something like a 'no shortcuts' view to cause prioritisation: you actually have to look hard at what you might do, rather than appealing to heuristics to do the work for you.
The connection between those two ideas is a good point that I don't think I'd fully taken away from this post, so thanks for making it more explicit.
I'm not sure precisely what you mean, so I'm not sure whether I agree. Here's an attempt to flesh out one thing might mean, which is something I'd agree with:
"Cause prioritisation depends on at least implicit consideration of how cost-effective the most cost-effective set of interventions within a given cause area are. Therefore, to be very confident about the relative priority of different cause areas, you would have to be confident that you've determined which interventions within each of those cause areas would be most cost-effective and that you've determined the relative cost-effective of those interventions. The further you are from that ideal, the less confident you should be.
However, that ideal would be very hard to reach. It would also require choosing areas to build up expertise and connections in, in order to better determine which interventions could be done, which would be most cost-effective, and how cost-effective they'd be. So in practice, the EA community will do more good if we often use cheaper heuristics to make tentative decisions about which areas to prioritise learning about or working on.
But we should remember that we can't be very confident about these things, and that it's quite possible that cause areas we haven't considered or that we considered and then de-prioritised are actually more important. (And one of the many reasons for this is that we almost certainly ignored some possible interventions in those cause areas, and failed to do good cost-effectiveness analyses of the interventions we did consider.)"
Does this roughly match what you meant / what you believe?
In brief, I'm sceptical there are good heuristics for assessing an entire problem. Ask yourself: what are they, and what is the justification for them? What do, rather, is have intuitive views about how effective particular solutions to given problems are. So we should think more carefully about those.
If it helps, for context, I started writing my thesis is 2015. At that time, EAs (following, I think, Will's book at 80k's then analysis) seemed to think you could make enormous progress on what the priorities are by appealing to very vague and abstract heuristics like "the bigger the problem, the higher the EV". This all seemed and seems v suspicious to me. People don't do this so much anymore.
Hmm, I feel we might be talking past each other slightly or something.
My impression is that Happier Lives Institute is already taking or planning to take both: (a) actions largely optimised for helping us identify the most cost-effective actions to improve near-term human wellbeing[1], and evaluate their cost-effectiveness, and (b) actions largely optimised for relatively directly improving near-term human wellbeing.
The fact that you're doing or planning to do (b) implies that you have at least implicitly prioritised near-term human wellbeing over other issues, right? And since you've done it before we've thoroughly considered a wide range of interventions in a wide range of cause areas and decently evaluated their cost-effectiveness, it seems you are in some sense appealing to heuristics for the purpose of cause prioritisation?
So it seems like maybe what you're saying is mainly that we should remember that our cause priorities should currently be considered quite preliminary and uncertain, rather than that we can't have cause priorities yet?
(Also, FWIW, for tentative cause prioritisation it does seem to me that there are a range of heuristics which can be useful, even if they're not totally decisive. I have in mind things including but not limited to ITN. But there's already been a lot of debate on the value of many of those specific heuristics, and I imagine you discuss some in your thesis but I haven't read it.)
[1] I'm not sure if this is precisely how you'd define HLI's focus. By "near-term" I have in mind something like "within the next 100 years".
This matches at least my take on this.
prescriptively, I would add that this contributes to the importance of being open to other people's ideas about how to do good (even if they are not familiar with EA).
Thanks for this summary! I found it interesting - perhaps particularly the distinction between primary, secondary, and meta causes, and the discussion of how one can go from considering primary causes to considering secondary and meta causes.
I also find it interesting that it sounds like Michael was able to use the process of writing a philosophy PhD thesis to do work that's not only directly relevant to specific actions, but also has in fact been acted on (via his creation of a related charity)!
This post also vaguely reminds me of a post I coauthored last year, which might be of interest to some readers: Four components of strategy research. (I say "to some readers" rather than "to you" since I know you already read & commented on the post :) )
The post "[Outlines] one way to decompose strategy research[.] Specifically, we break it down into the following four components: mapping the space, constructing strategies, modelling causality, and prioritizing between strategies".