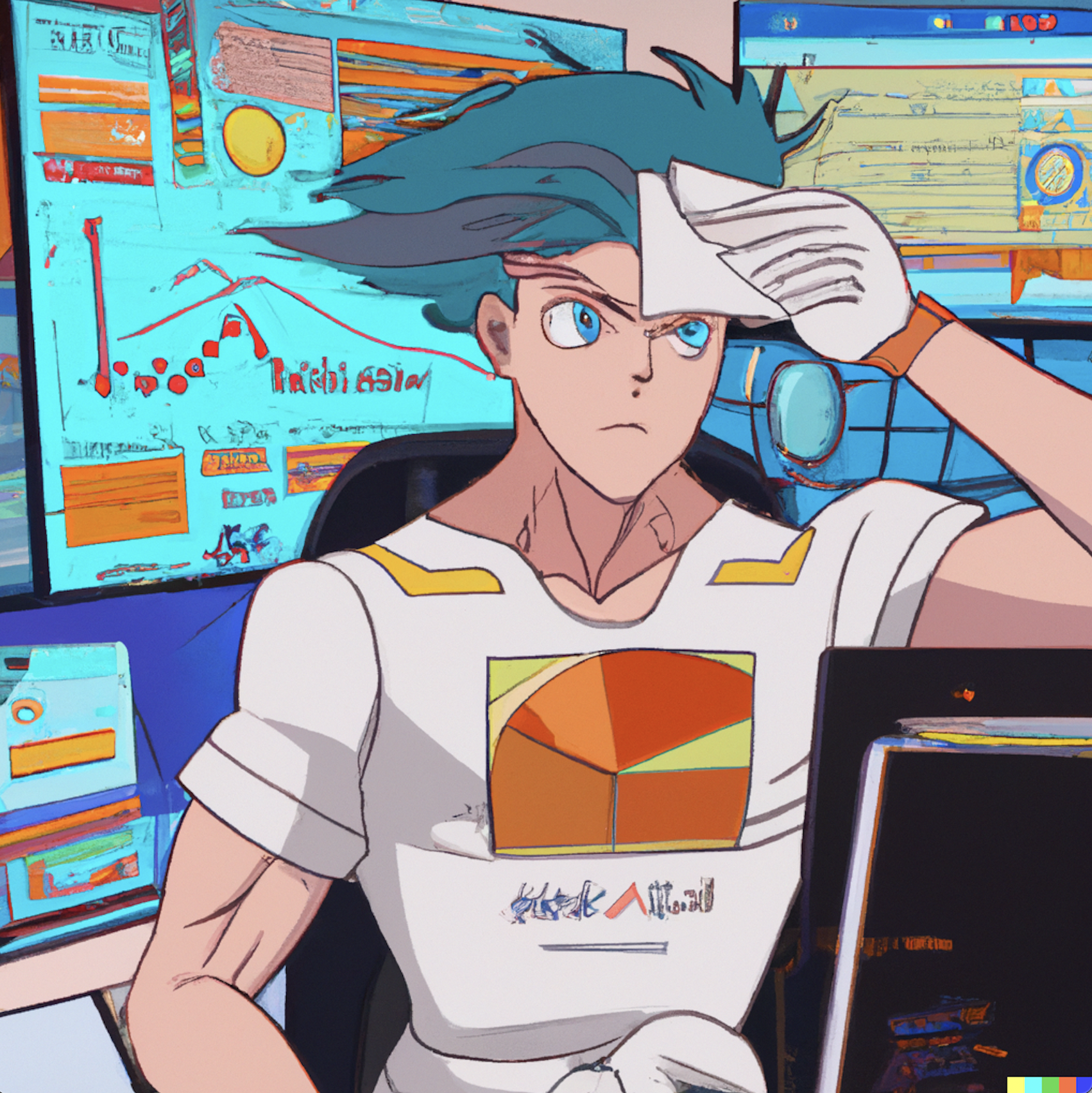
Motivation
The team at QURI has recently released Squiggle, a very new and experimental programming language for probabilistic estimation. We’re curious about what promising use cases it could enable, and we are launching a prize to incentivize people to find this out.
Prize
We offer a $600 first-place prize, a $300 second-place prize, and a $100 third-place prize. The prize will be paid for by the Quantified Uncertainty Research Institute (QURI).
Rules
To enter, first make a public post online between now and September 1, 2022. We encourage you to either post directly or make a link post to either the EA Forum or to LessWrong. Second, complete this form, also before September 1, 2022.
We’ll aim to make decisions by October 1, 2022.
More specifically:
- Experiment with Squiggle. Make an interesting model with it or develop a tech prototype or integration using it.
- Make a (short is fine) blog post about your experiment. If you post to the EA Forum or LessWrong, others can more easily share their opinions. In your post, if you could include some honest feedback on how you found working with Squiggle, that would be appreciated, but it's not required.
- Submit this form with the key details. Submit it by September 1, 2022.
Support & Feedback
If you’d like feedback or would like to discuss possible projects, please reach out! (via direct message or email.) We’ll invite you to our Slack and can give feedback and advice. Also, feel free to file issues or comments/questions/suggestions on the Squiggle Github page.
Judges
The judges will be Nuño Sempere, Ozzie Gooen, and Quinn Dougherty. The rest of the Squiggle team will also consult. We’ll select winners for their importance, novelty, and presentation.
Ideas
Some ideas, any of which could be taken from the recent post:
Software Ideas
- Build integrations with other tools. Google Docs, Airtable, Roam Research, Obsidian, Python, other forecasting platforms, etc.
- Integrate Squiggle in other software platforms or tools. Maybe use it to power an online calculator to return probability distributions instead of numbers.
- Make open-source React components around probability distributions better visualize the outputs of Squiggle models.
- Build neat tools on top of Squiggle. For example, Squiggle could help power a node-based graphical editor or a calendar-based time estimation tool. Users might not need to know anything about Squiggle; it would just be used for internal math.
- Make Observable dashboards that take in real-time data from the web, and use Squiggle to do some simple math on them and return probability distributions or functions. (For instance, see this post that uses Squiggle in Observable.)
Research Ideas
- Estimate the entire value of the far future over time.
- Estimate EA funding over time.
- Convert several of the AI timelines estimates into Squiggle, then use Squiggle to help aggregate them, e.g. Cotra’s report, or Carlsmith’s. Carlsmith’s report mostly uses probabilities, but one could try to use distributions over probabilities representing the range of expert opinion instead.
Some existing tools that use Squiggle (for inspiration)
- This tool converts Guesstimate models into Squiggle models, by Lorenzo.
- Google Sheets integration by Nuño Sempere
- An API to interact with Squiggle by Nuño Sempere
- A work-in-progress interactive tool for making hierarchical estimates by Nuño Sempere
- An experimental Squiggle CLI for allowing imports, by QURI.
Reminder: Only a few days left for this! Submissions are closed on September 1st.