Arb is a new research consultancy. You can reach us at hi@arbresearch.com . Listen to this post on the Nonlinear Library.
The superforecasting phenomenon - that certain teams of forecasters are better than other prediction mechanisms like large crowds and simple statistical rules - seems sound. But serious interest in superforecasting stems from the reported triumph of forecaster generalists over non-forecaster experts. (Another version says that they also outperform analysts with classified information.)
So distinguish some claims:
- "Forecasters > the public"
- "Forecasters > simple models"
- "Forecasters > experts"
3a. "Forecasters > experts with classified info"
3b. "Averaged forecasters > experts"
3c. "Aggregated forecasters > experts"
Is (3) true? This post reviews all the studies we could find on experts vs forecasters. (We also attempt to cover the related question of prediction markets vs experts.)
Conclusions
First, our conclusions. These look pessimistic, but are mostly pretty uncertain:
- We think claim (1) is true with 99% confidence[1] and claim (2) is true with 95% confidence. But surprisingly few studies compare experts to generalists (i.e. study claim 3). Of those we found, the analysis quality and transparency leave much to be desired. The best study found that forecasters and health professionals performed similarly. In other studies, experts had goals besides accuracy, or there were too few of them to produce a good aggregate prediction.
- (3a) A common misconception is that superforecasters outperformed intelligence analysts by 30%. Instead: Goldstein et al showed that [EDIT: the Good Judgment Project's best-performing aggregation method][2] outperformed the intelligence community, but this was partly due to the different aggregation technique used (the GJP weighting algorithm performs better than prediction markets, given the apparently low volumes of the ICPM market). The forecaster prediction market performed about as well as the intelligence analyst prediction market; and in general, prediction pools outperform prediction markets in the current market regime (e.g. low subsidies, low volume, perverse incentives, narrow demographics). [85% confidence]
- (3b) In the same study, the forecaster average was notably worse than the intelligence community.
- (3c) Ideally, we would pit a crowd of forecasters against a crowd of experts. Only one study, an unpublished extension of Sell et al. manages this; it found a small (~3%) forecaster advantage.
- The bar may be low. That is: it doesn't seem that hard to become a top forecaster, at present. Expertise, plus basic forecasting training and active willingness to forecast regularly, were enough to be on par with the best forecasters. [33%]
[3] - In more complex domains, like ML, there could be significant returns to expertise. So it might be better to broaden focus from generalist forecasters to competent ML pros who are excited about forecasting. [40%]
Table of studies
Due to issues with rendering tables on the forum, please, see the table in a google doc version of this post.
Search criteria
We were given a set of initial studies to branch out from.
- Good Judgement Project
- Tom McAndrew studies
- Hypermind + Johns Hopkins
And some general suggestions for scholarship:
- look for review articles
- look for textbooks and handbooks or companions
- find key terms
- go through researchers’ homepages/google scholar
Superforecasting began with IARPA’s ACE tournament. (Misha thinks the evidence in Tetlock’s Expert Political Judgment doesn’t fit for our purposes: there were no known skilled-amateur forecasters at that point.)
A Google Scholar search for studies funded by IARPA ACE yielded no studies. We looked at other IARPA projects (ForeST, HCT, and OSI), which sounded remotely relevant to our goals.
We searched Google Scholar for (non-exhaustive list): “good judgment project”, “superforecasters”, “collective intelligence”, “wisdom of crowds”, “crowd prediction”, “judgemental forecasting”, …, and various combinations of these, and “comparison”, “experts”, …
We got niche prediction markets from the Database of Prediction Marketsand searched for studies mentioning them. Hollywood SX and FantasySCOTUS paid off as a result. We also searched for things people commonly predict: sports, elections, Oscars, and macroeconomics.
In the process, we read the papers for additional keywords and references. We also looked for other papers from the authors we encountered.
On AI forecasting
In more complex domains, like ML, there could be significant returns to knowledge and expertise. It seems to us that moving from generalist forecasters to competent ML practitioners/researchers might be in order, because:
- To predict e.g. scaling laws and emerging capabilities, people need to understand them, which requires some expertise and understanding of ML
- It's unclear whether general forecasters actually outperform experts in a legible domain, even though we believe in the phenomenon of superforecasting, (that some people are much better forecasters than most). We also liked David Manheim's take on Superforecasting.
- We think that this will plausibly reduce ML researchers’ aversion to forecasting proposals — and if we were to execute it, we would be selecting good forecasters based on their performance anyway. It seems potentially feasible.
Finally, we note that the above is heavily limited by lack of data (lack of data collected and a lack of availability). We hope that the experimental data gets reanalyzed at least.
This report represents 2.1 person-weeks of effort.
Thanks to Emile Servan-Schreiber, Luke Muehlhauser, and Javier Prieto for comments. These commenters don't necessarily endorse any of this. Mistakes are our own. Research funded by Open Philanthropy.
Appendix: Table of less relevant studies
Appendix: markets vs pools
Appendix: Expert Political Judgment (2005)
Appendix: by methods compared
Changelog for this post
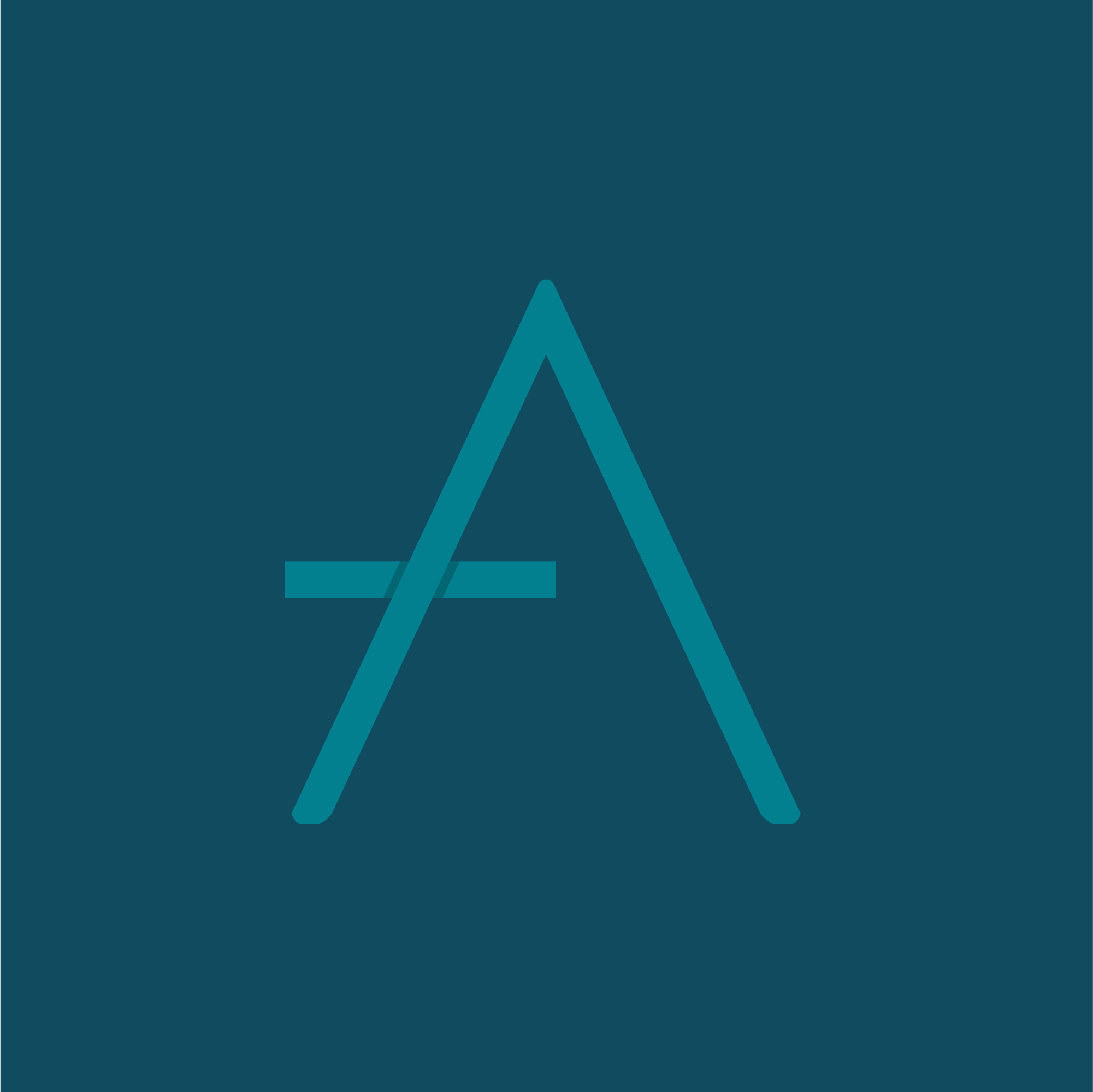
skin clear, crops flourishing, grades up.
- ^
This is almost a trivial claim, since forecasters are by definition more interested in current affairs than average, and much more interested in epistemics than average. So we’d select for the subset of “the public” who should outperform simply through increased effort, even if all the practice and formal calibration training did nothing, and it probably does do something.
- ^
Previously this section said "superforecasters"; after discussion, it seems more prudent to say "the Good Judgment Project's best-performing aggregation method". See this comment for details.
- ^
Our exact probability hinges on what's considered low and on how good e.g. Hypermind's trained forecasters are. This is less obvious than it seems: in the CSET Foretell tournament, the top forecasters were not uniformly good; a team which included Misha finished with a 4x better relative Brier score than the "top forecaster" team. Further, our priors are mixed: (a) common sense makes us favor experts, (b) but common sense also somewhat favors expert forecasters, (c) Tetlock's work on expert political judgment pushes us away from politics experts, and finally (d) we have first-hand experience about superforecasting being real.
- ^
All Surveys Logit "takes the most recent forecasts from a selection of individuals in GJP’s survey elicitation condition, weights them based on a forecaster’s historical accuracy, expertise, and psychometric profile, and then extremizes the aggregate forecast (towards 1 or 0) using an optimized extremization coefficient.” Note that this method was selected post hoc, which raises the question of multiple comparisons; the authors respond that “several other GJP methods were of similar accuracy (<2% difference in accuracy).”
- ^
There is some inconclusive research comparing real- and play-money: Servan-Schreiber et al. (2004) find no significant difference for predicting NFL (American football); Rosenbloom & Notz (2006) find that in non-sports events, real-money markets are more accurate and that they are comparably accurate for sports markets; and Slamka et al. (2008) finds real- and play-money prediction markets comparable for UEFA (soccer).
- ^
MMBD is not a proper scoring rule (one incentivizing truthful reporting). If a question has a chance of resolving early (e.g., all questions of the form “will X occur by date?”), the rule incentivizes forecasters to report higher probabilities for such outcomes. This could have affected GJP (avg and best) predictors, who were rewarded for it; but should have not affected ICPM and GJP (PM), as these used the Logarithmic Market Scoring Rule.
See Sempere & Lawsen (2021) for details. - ^
Our understanding is that these were not averaged. On average there were ~2.5 imputed predictions per report.
- ^
It's unclear if imputers did a reasonable job separating their personal views from their imputations. Mandel (2019) notes that the Pearson correlation between mean Brier scores for personal and imputed forecasts is very high, r(3)=.98, p=.005. Imputers average Brier scores ranged from .145 to .362 suggesting that traditional analysis’ apparent accuracy depends on whether interpreters are better or worse forecasters. Lehner and Stastny (2019) responded.
- ^
"We replicated some of these markets in the ICPM, or identified closely analogous predictions if they existed, so that direct comparisons between the two prediction markets could be made over time."
- ^
“We repeatedly collected forecasts from our markets and our experts to sample various time horizons, ranging from very near-term forecasts to as long as 4 months before a subject was resolved. All told, we collected 152 individual forecasts from the ICPM, InTrade, and individual IC experts over approximately matching topics and time horizons.”
- ^
A perfectly calibrated forecaster expects on average brier points from their prediction. So this average Brier suggests that a “typical" InTrade prediction was either <4% or >96%. From experience, this feels too confident and suggests that questions were either biased towards low noise or that luck is partly responsible for such good performance.
- ^
Personal communication with Servan-Schreiber.
- ^
Given N log scores, scaled rank assigns a value of 1/N to the smallest log score, a value of 2/N to the second smallest log score, and so on, assigning a value of 1 to the highest log score. (As with log scores, here computed from probability density functions, — the higher rank the better.)
- ^
It’s unclear to me how well they did compare to a prior based on how often SCOTUS reverses the decisions. The historical average is ~70% with ~80% reversals in 2008, the relevant term.
Interesting side-finding: prediction markets seem notably worse than cleverly aggregated prediction pools (at least when liquidity is as low as in the play markets). Not many studies, but see Appendix A for what we've found.
Thank you for writing this overview! I think it's very useful. A few notes on the famous "30%" claim:
One under-appreciated takeaway that you hint at is that prediction markets (rather than non-market aggregation platforms) are poorly suited to classified environments. Here's a quote from a white paper I co-wrote last year:[2]
More broadly, I would like to push back a little against the idea that your point 3(a) ( whether supers outperform IC analysts) is really much evidence for or against 3 (whether supers outperform domain experts).
First, the IARPA tournaments asked a wide range of questions, but intelligence analysts tend to be specialized. If you're looking at the ICPM, are you really looking at the performance of domain experts? Or are you looking at e.g. an expert on politics in the Horn of Africa trying to forecast the price of the Ruble? On the one hand, since participants self-selected which questions they answered, we might expect domain experts to stick to their domain. On the other, analysts might have seen it as a "game," a "break," or "professional development" -- in short, an opportunity to try their hand something outside their expertise. The point is that we simply don't know whether the ICPM really reflects "expert" opinion.
Second, I am inclined to believe that comparisons between IC analysts and supers may tell us more about the secrecy heuristic than about forecaster performance. From the same white paper:
I personally see much of the promise of forecasting platforms not as a tool for beating experts, but as a tool for identifying them more reliably (more reliably than by the usual signals, like a PhD).
Tetlock discusses this a bit in Chapter 4 of Superforecasting.
Keeping Score: A New Approach to Geopolitical Forecasting, https://global.upenn.edu/sites/default/files/perry-world-house/Keeping%20Score%20Forecasting%20White%20Paper.pdf.
Travers et al., "The Secrecy Heuristic," https://www.jstor.org/stable/43785861.
This is extremely helpful and a deep cut - thanks Christian. I've linked to it in the post.
Yeah, our read of Goldstein isn't much evidence against (3), we're just resetting the table, since previously people used it as strong evidence for (3).
Thanks Gavin! That makes sense on how you view this and (3).
David Manheim's 2020 viewpoint prefigures some of the above, but goes further in questioning the superforecaster phenomenon (by reducing it to intelligence + open-mindedness + giving a damn).
Thanks for this, it's really helpful! I find it very plausible to me that "generalist forecasters are the most accurate source for predictions on ~any question" has become too much of a community shibboleth. This is a useful correction.
Given how widely the "forecasters are better than experts!" meme has spread, point 3a seems particularly important to me (emphasis mine):
I would have found a couple more discussion paragraphs helpful. As written, it's difficult for me to tell which studies you think are most influential in shaping the conclusions you lay out in the summary paragraph at the beginning of the post. The "Summary" section of the post isn't actually summarizing the rest of the post; instead, that's just where your discussion and conclusions are being presented.
I'm excited to potentially see more critical analysis of the forecasting literature! Plus ideas for new studies that can help identify the conditions under which forecasters are most accurate/helpful.
Renamed the summary section, thanks
Thank you! We might consider editing the summary. This particular point is mostly supported by our takes on Goldstein et al (2015) and by Appendix A.
These claims about Superforecasting are eye-catching. However, it's difficult to draw any conclusions when most of the research cited doesn't in fact include Superforecasters. In our view, it isn't a matter of Superforecasters vs experts: the Boolean is "and" as much as possible to get the best results.
For those who are interested in taking a deeper dive into the peer-reviewed literature, though, take a look here:
https://goodjudgment.com/about/the-science-of-superforecasting/
Some of our work on combining forecasters and experts is here:
https://www.foreignaffairs.com/articles/united-states/2020-10-13/better-crystal-ball
https://warontherocks.com/2021/07/did-sino-american-relations-have-to-deteriorate-a-better-way-of-doing-counterfactual-thought-experiments/
Where's the "delta" upvote when I need it? :)
Appreciate that, Yonatan! :)
In principle, I like the research question and the comparison above is probably the most you can make out from what is published. That said, it is the year 2022, capabilites and methodology have advanced enormously at least with those PM firms operating successfully in the commercial world markets. So it's the proverbial comparing apples and oranges on several dimensions to talk about how "prediction markets" (sic) perform for whatever. Different platform implementations have very different capabilities suited to very different tasks. Moreover, like any advanced tool, practical application of the more advanced PM platforms need a high degree of methodic knowhow on how to use their specific capabilities - based on real experience of what works and what does't.
As a semi-active user of prediction markets and a person who looked up a bunch of studies about them, I don't see that many innovations or at least anything that crucially changes the picture. I would be excited to be proven wrong, and am curious to know what you would characterize as advances in capability and methodology.
I am partly basing my impression on Mellers & Tetlock (2019), they write "We gradually got better at improving prediction polls with various behavioral and statistical interventions, but it proved stubbornly hard to improve prediction markets." And my impression is that they experimented quite a bit with them.
So here's a potentially fatal flaw in this analysis:
You write, "Goldstein et al showed that superforecasters outperformed the intelligence community...."
But the Goldstein paper was not about the Superforecasters. Your analysis, footnote 4, says, "'All Surveys Logit' takes the most recent forecasts from a selection of individuals in GJP’s survey elicitation condition...."
Thousands of individuals were in GJP's survey elicitation condition, of whom only fraction (a few dozen) were Superforecasters.
So Goldstein did not find that "superforecasters outperformed the intelligence community"; rather, he found that [thousands of regular forecasters + a few dozen Superforecasters] outperformed the intelligence community. That's an even lower bar.
Please check for yourself. All GJP data is publicly-available here: https://dataverse.harvard.edu/dataverse/gjp.
Thanks for engaging with our post!
Here is Mellers et al. (2017) about the study:
(Emphasis mine.)
I believe their assessment of whether it's fair to call one of "GJP best methods" "superforecasters" is more authoritative as the term originated from their research (and comes with a better understanding of methodology).
Anyways, the "GJP best method" used all Brier score boosting adjustments discussed in the literature (maybe excluding teaming), including selecting individuals (see below). And, IIRC, superforecasters are basically forecasters selected based on their performance.
Hi @Misha, Thank you for your patience and sorry for the delay.
I triple-checked. Without any doubt, the "All Surveys Logit" used forecast data from thousands of "regular" forecasters and several dozen Superforecasters.
So it is the case that [regular forecasters + Superforecasters] outperformed U.S. intelligence analysts on the same questions by roughly 30%. It is NOT the case that the ICPM was compared directly and solely against Superforecasters.
It may be true, as you say, that there is a "common misconception...that superforecasters outperformed intelligence analysts by 30%" -- but the Goldstein paper does not contain data that permits a direct comparison of intelligence analysts and Superforecasters.
The sentence in the 2017 article you cite contains an error. Simple typo? No idea. But typos happen and it's not the end of the world. For example, in the table above, in the box with the Goldstein study, we see "N = 193 geopolitical questions." That's a typo. It is N = 139.
All Survey Logit was the best method out of the many methods the study tried. Their class of methods is flexible enough to include superforecasters as they were trying weighting forecasters by past performance (and as the research was done based on year 3 data the superforecasters were a salient option). By construction ASL is superforecaster level or above.
Oh my! May I ask, have you actually contacted anyone at Good Judgment to check? Because your assertion is simply not correct.
Upd 2022-03-14: Good Judgement Inc representative confirmed that Goldstein et al (2015) didn't have a superforecaster-only pool. Unfortunately, the citations above are indeed misleading; as of now, we are not aware of research comparing superforecasters and ICPM.
Upd 2022-03-08: after some thought, we decided to revisit the post to be more precise. While this study has been referenced multiple times as superforecasters vs ICPM it's unclear whether one of the twenty algorithms compared used only superforecasters (which seems plausible, see below). We still believe that Goldstein et al bear on how well the best prediction pools do, compared to ICPM. The main question about All Surveys Logit, whether the performance gap is due to the different aggregation algorithms used, also applies to claims about superforecasters.
Lastly, even if we assume that claims of superforecasters performance in comparison with IC haven't been backed by this (or any other) study[1], the substantive claim hold: the 30% edge is likely partly due to the different aggregation techniques used stands.
As I reassert in this comment, everyone refers to this study as a justification; and upon extensive literature search, I haven't found other comparisons. ↩︎
Hi again Misha,
Not sure what the finding here is: "...the 30% edge is likely partly due to the different aggregation techniques used...." [emphasis mine]
How can we know more than likely partly? On what basis can we make a determination? Goldstein et. al. posit several hypotheses for the 30% advantage Good Judgment had over the ICPM: 1) GJ folks were paid; 2) a "secrecy heuristic" posited by Travers et. al.; 3) aggregation algorithms; 4) etc.
Have you disaggregated these effects such that we can know the extent to which the aggregation techniques boosted accuracy? Maybe the effect was entirely related to the $150 Amazon gift cards that GJ forecasters received for 12 months work? Maybe the "secrecy heuristic" explains the delta?
Thank you, Tim! Likely partly due to is my impressions of what's going on based on existing research; I think we know that it is "likely partly" but probably not much more based on current literature.
The line of reasoning which I find plausible is "GJP PM and GJP All Surveys Logit" is more or less the same pool of people but the one aggregation algorithm is much better than another; it's plausible that "IC All Surveys Logit would improve on ICPM quite dramatically." And because the difference between GJP PM and ICPM is small it feels plausible that if the best aggregation method would be applied to IC, IC would cut the aforementioned 30% gap.
(I am happy to change my mind upon seeing more research comparing strong forecasters and domain experts.)
Just emailed Good Judgment Inc about it.
Thanks for catching a typo! Appreciate the heads up.
From the conclusion of this new paper https://psyarxiv.com/rm49a/
We checked to see if Tetlock's 2005 book had anything to tell us about our question.
Despite my own and others' recollection that it shows that top generalists match experts, the main RFE experiment turns out to compare PhD area experts against PhD experts outside their precise area. The confusion arises because he uses the word "dilettante" for these latter experts, and doesn't define this until the last appendix.
Be sure to check out the vast chasm between the experts and random undergrads.
One nice little study which was out of scope: ClearerThinking vs Good Judgment Inc vs MTurk on Trump policies. (This has been advertised as superforecasters vs experts, but it isn't.)
this might be due to a change on EA forum since you initially posted this post, but the left and right columns of the table of studies are quite unreadable for me on desktop, on both Chrome and Edge. see screenshot for what it looks like from my end. is there any other format I can read this post in?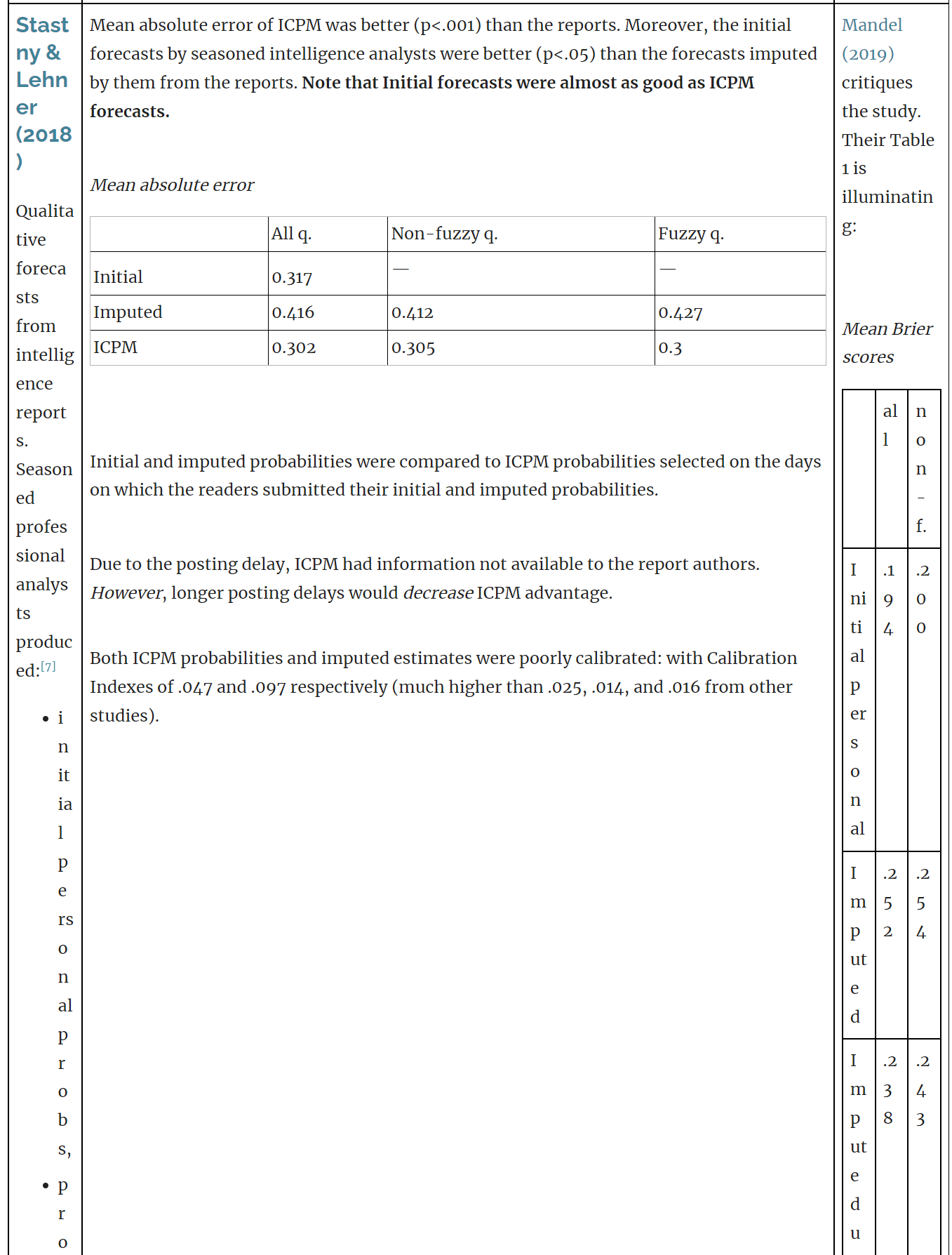
Oh that is annoying, thanks for pointing it out. I've just tried to use the new column width feature to fix it, but no luck.
Here's a slightly more readable gdoc.
Our recent submission, "Training experts to be forecasters", to the cause exploration prize may be of interest (I certainly found this post interesting as a justification for some of the ideas we experiment with).
https://forum.effectivealtruism.org/posts/WFbf2d4LHjgvWJCus/cause-exploration-prizes-training-experts-to-be-forecasters
Can someone clarify these statements from Summary (3a)? They seem to be at odds....
A: "A common misconception is that superforecasters outperformed intelligence analysts by 30%."
B: "Instead: Goldstein et al showed that superforecasters outperformed the intelligence community..."[then a table listing the ICPM MMDB as 0.23 versus the GJP Best MMDB as 0.15].
--> Wouldn't that be 34% better?
Indeed, but the misconception/lack of nuance is specifically about 30% here is Wikipedia on Good Judgement Project. I guess it's either about looking at preliminary data or rounding.
It is, but we're talking about the misconception, which became "30 percent" in (e.g.) this article.
Sorry, I'm confused. Do you mean the misconception is that rather than "30%" we should be saying that GJP was "34.7%" better than the ICPM?
It's indeed the case that GJP was 34.7% better than the ICPM. But it's not the case that GJP participants were 34.7% better than intelligence analysts. The intelligent analyst used prediction markets that are generally worse than prediction pools (see Appendix A), so we are not comparing apples to apples.
It would be fair to judge IC for using prediction markets rather than prediction pools after seeing research coming out of GJP. But we don't know how an intelligence analyst prediction pool would perform compared to the GJP prediction pool. We have reasons to believe that difference might not be that impressive based on ICPM vs GJP PM and based on Sell et al (2021).
There's three things
The important misconception is using (2) as if it was (1). Sentence A is about misunderstanding the relationship between the above three things, so it seems fine to use the number from (3). We haven't seen anyone with misconceptions about the precise 34.7% figure and we're not attributing the error to Goldstein et al.
Curious: You say the 2015 Seth Goldstein "unpublished document" was "used to justify the famous 'Supers are 30% better than the CIA' claim."
But that was reported two years earlier, in 2013: https://www.washingtonpost.com/opinions/david-ignatius-more-chatter-than-needed/2013/11/01/1194a984-425a-11e3-a624-41d661b0bb78_story.html.
So how was the 2015 paper the justification?
The linked story doesn't cite another paper, so it's hard to guess their actual source. Generally, academic research takes a while to be written and get published; the 2015 version of the paper seems to be the latest draft in circulation. It's not uncommon to share and cite papers before they get published.
Thanks for the clarification, @Misha-Yagudin.
So to be clear, in his November 1, 2013 article, David Ignatius had access to forecasting data from the period August 1, 2013 through May 9, 2014!! (See section 5 of the Seth Goldstein paper underlying your analysis).
That, my friend, is quite the feat!!
Good catch, Tim! Well, at least Good Judgement Inc. (and some papers I've seen) cite Goldstein et al (2015) straight after David Ignatius's 30% claim: https://goodjudgment.com/resources/the-superforecasters-track-record/superforecasters-vs-the-icpm/
If you by any chance have another paper[1] or resource in mind regarding the 30% claim, I would love to include it in the review.
Note that Goldstein et al don't make that claim themselves, their discussion and conclusion are nuanced. ↩︎
Christian Ruhl confirms that results from ACE were leaked early to Ignatius.